Momentum-based Gradient Methods in Multi-Objective Recommendation.
MORS@RecSys(2021)
Abstract
Multi-objective gradient methods are becoming the standard for solving multi-objective problems. Among others, they show promising results in developing multi-objective recommender systems with both correlated and conflicting objectives. Classic multi-gradient~descent usually relies on the combination of the gradients, not including the computation of first and second moments of the gradients. This leads to a brittle behavior and misses important areas in the solution space. In this work, we create a multi-objective model-agnostic Adamize method that leverages the benefits of the Adam optimizer in single-objective problems. This corrects and stabilizes~the~gradients of every objective before calculating a common gradient descent vector that optimizes all the objectives simultaneously. We evaluate the benefits of Multi-objective Adamize on two multi-objective recommender systems and for three different objective combinations, both correlated or conflicting. We report significant improvements, measured with three different Pareto front metrics: hypervolume, coverage, and spacing. Finally, we show that the \textit{Adamized} Pareto front strictly dominates the previous one on multiple objective pairs.
MoreTranslated text
Key words
gradient methods,momentum-based,multi-objective
AI Read Science
Must-Reading Tree
Example
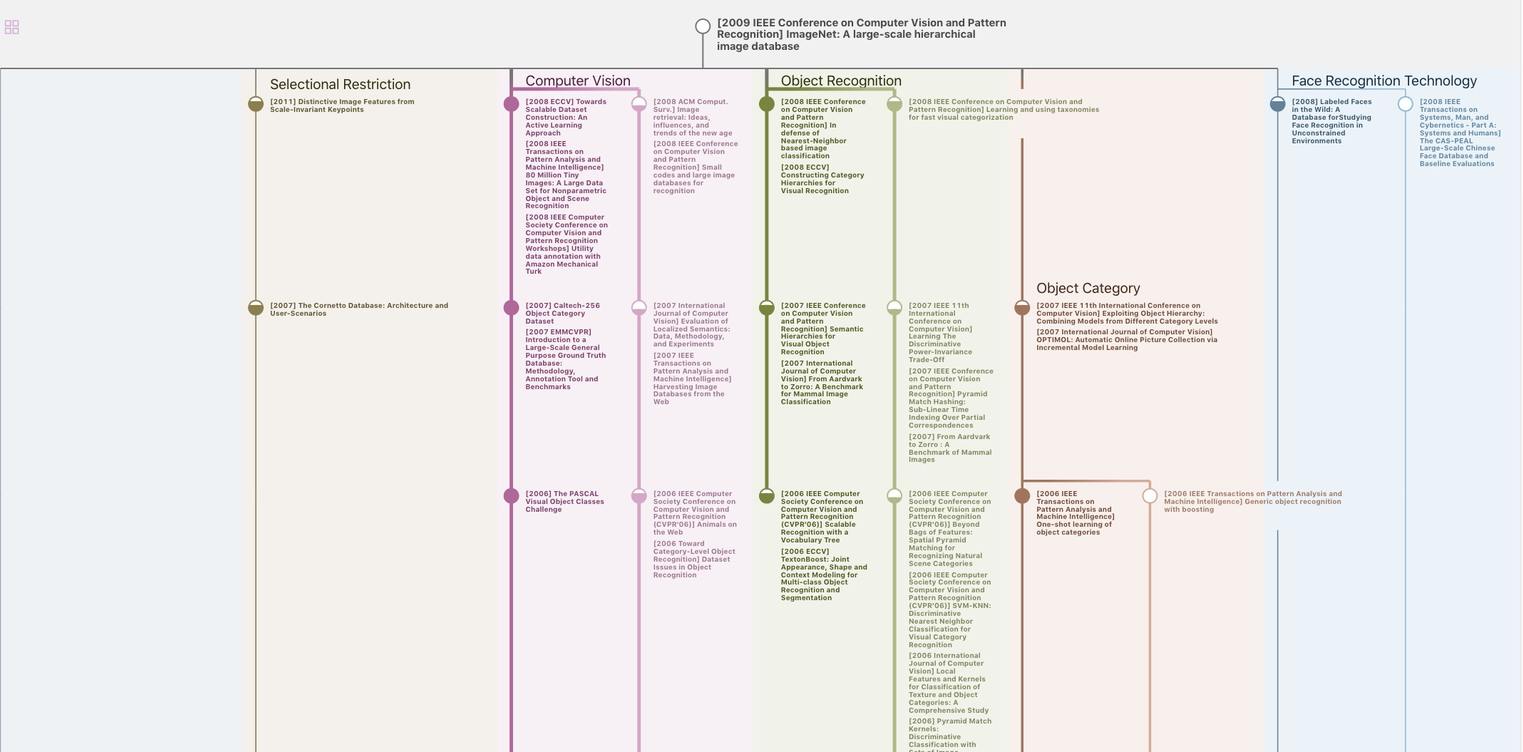
Generate MRT to find the research sequence of this paper
Chat Paper
Summary is being generated by the instructions you defined