Beyond Observed Connections : Link Injection
arXiv (Cornell University)(2020)
Abstract
In this paper, we proposed the \textit{link injection}, a novel method that helps any differentiable graph machine learning models to go beyond observed connections from the input data in an end-to-end learning fashion. It finds out (weak) connections in favor of the current task that is not present in the input data via a parametric link injection layer. We evaluate our method on both node classification and link prediction tasks using a series of state-of-the-art graph convolution networks. Results show that the link injection helps a variety of models to achieve better performances on both applications. Further empirical analysis shows a great potential of this method in efficiently exploiting unseen connections from the injected links.
MoreTranslated text
Key words
observed connections,link injection
AI Read Science
Must-Reading Tree
Example
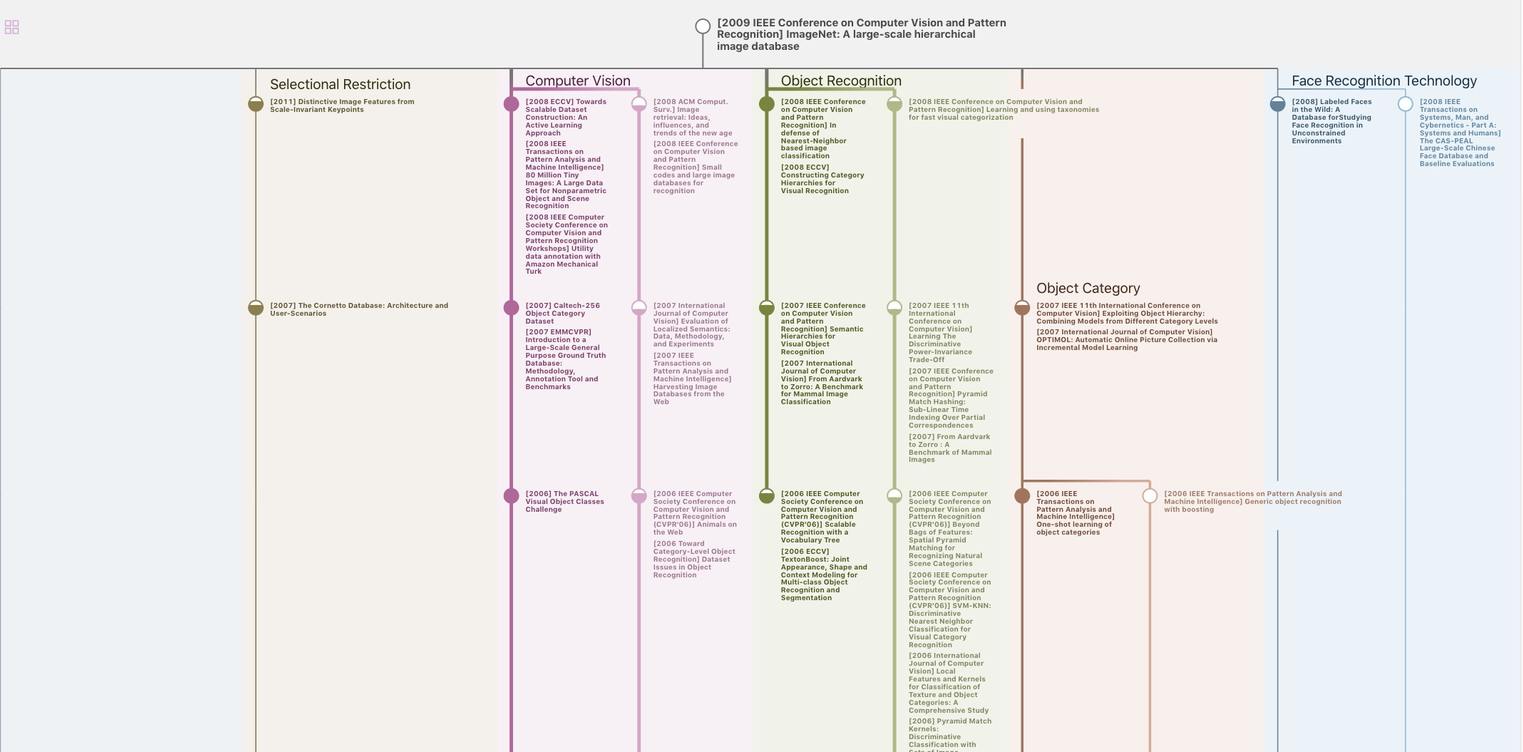
Generate MRT to find the research sequence of this paper
Chat Paper
Summary is being generated by the instructions you defined