Real-time Bayesian personalization via a learnable brain tumor growth model
arxiv(2020)
摘要
Modeling of brain tumor dynamics has the potential to advance therapeutic planning. Current modeling approaches resort to numerical solvers that simulate the tumor progression according to a given differential equation. Using highly-efficient numerical solvers, a single forward simulation takes up to a few minutes of compute. At the same time, clinical applications of the tumor modeling often imply solving an inverse problem, requiring up to tens of thousands forward model evaluations when used for a Bayesian model personalization via sampling. This results in a total inference time prohibitively expensive for clinical translation. Moreover, while recent data-driven approaches become capable of emulating physics simulation, they tend to fail in generalizing over the variability of the boundary conditions imposed by the patient-specific anatomy. In this paper, we propose a learnable surrogate with anatomy encoder for simulating tumor growth which maps the biophysical model parameters directly to simulation outputs, i.e. the local tumor cell densities. We test the surrogate on Bayesian tumor model personalization for a cohort of glioma patients. Bayesian inference using the proposed neural surrogate yields estimates analogous to those obtained by solving the forward model with a regular numerical solver. The near real-time computation cost renders the proposed method suitable for clinical settings. The code is available at https://github.com/IvanEz/tumor-surrogate.
更多查看译文
关键词
bayesian personalization,tumor,brain,real-time
AI 理解论文
溯源树
样例
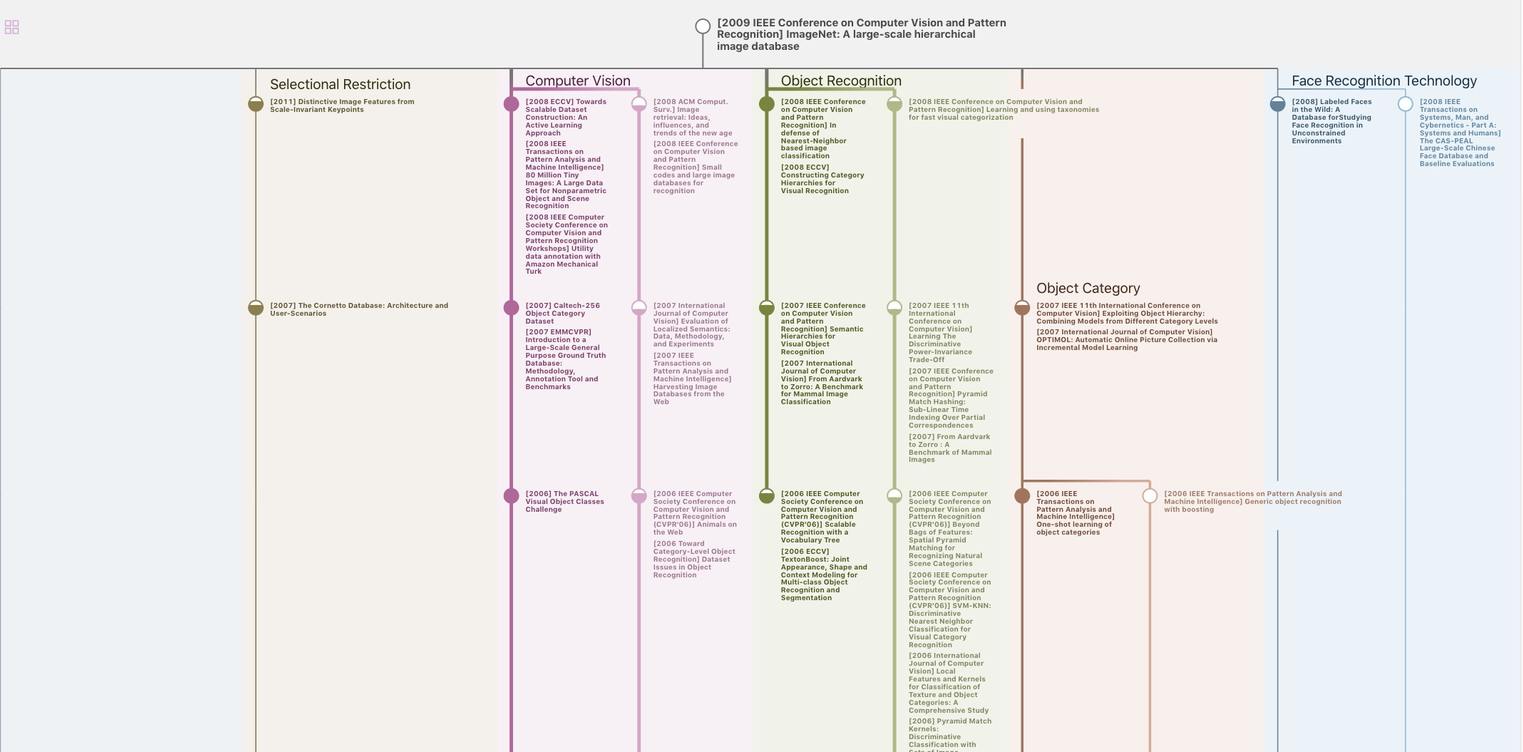
生成溯源树,研究论文发展脉络
Chat Paper
正在生成论文摘要