Utilization Of The Signature Method To Identify The Early Onset Of Sepsis From Multivariate Physiological Time Series In Critical Care Monitoring
CRITICAL CARE MEDICINE(2020)
摘要
Objectives: Patients in an ICU are particularly vulnerable to sepsis. It is therefore important to detect its onset as early as possible. This study focuses on the development and validation of a new signature-based regression model, augmented with a particular choice of the handcrafted features, to identify a patient's risk of sepsis based on physiologic data streams. The model makes a positive or negative prediction of sepsis for every time interval since admission to the ICU. Design: The data were sourced from the PhysioNet/Computing in Cardiology Challenge 2019 on the "Early Prediction of Sepsis from Clinical Data." It consisted of ICU patient data from three separate hospital systems. Algorithms were scored against a specially designed utility function that rewards early predictions in the most clinically relevant region around sepsis onset and penalizes late predictions and false positives. Setting: The work was completed as part of the PhysioNet 2019 Challenge alongside 104 other teams. Patients: PhysioNet sourced over 60,000 ICU patients with up to 40 clinical variables for each hour of a patient's ICU stay. The Sepsis-3 criteria was used to define the onset of sepsis. Interventions: None. Measurements and Main Results: The algorithm yielded a utility function score which was the first placed entry in the official phase of the challenge.
更多查看译文
关键词
competition, critical care, early detection and treatment, PhysioNet, sepsis
AI 理解论文
溯源树
样例
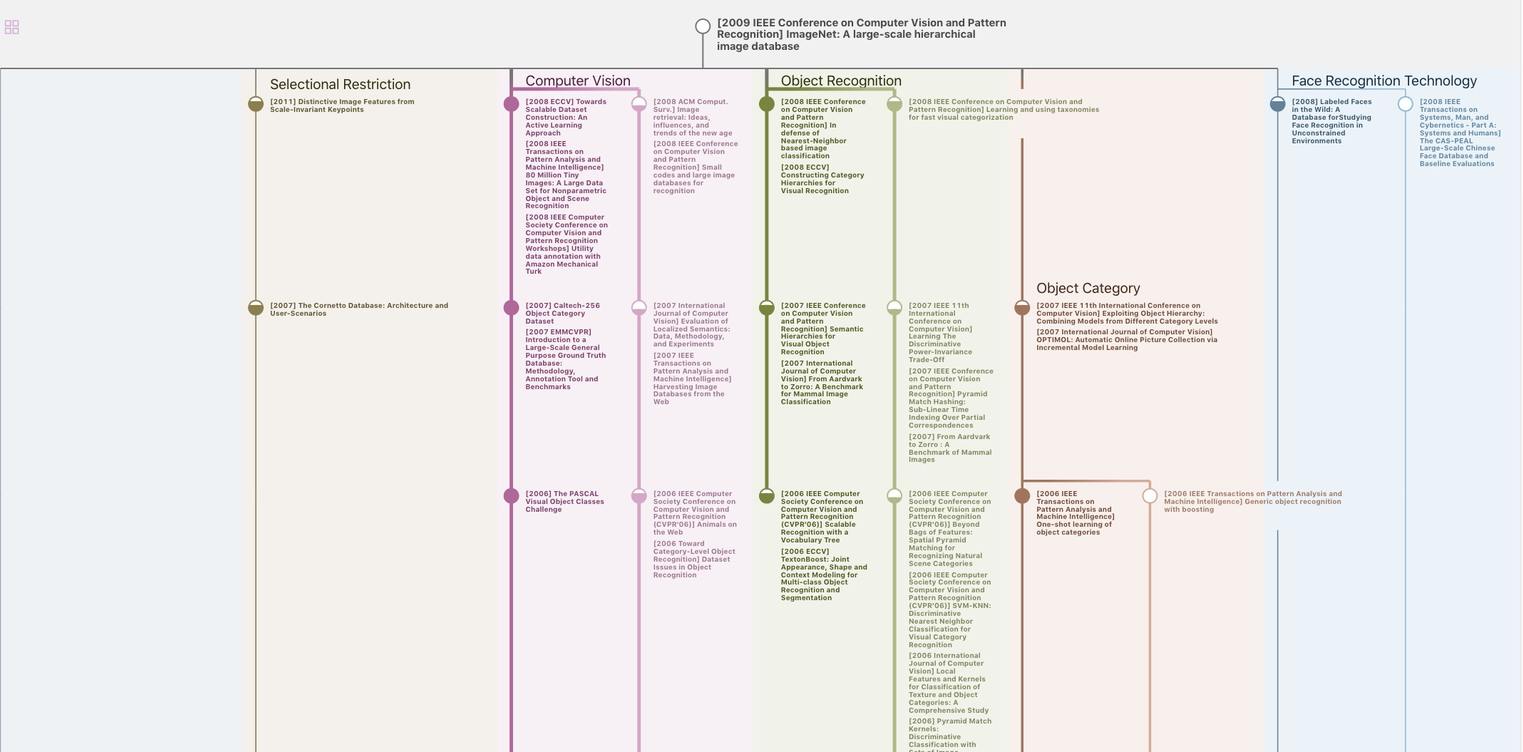
生成溯源树,研究论文发展脉络
Chat Paper
正在生成论文摘要