Alternating Direction Method Of Multipliers For Quantization
24TH INTERNATIONAL CONFERENCE ON ARTIFICIAL INTELLIGENCE AND STATISTICS (AISTATS)(2021)
摘要
Quantization of the parameters of machine learning models, such as deep neural networks, requires solving constrained optimization problems, where the constraint set is formed by the Cartesian product of many simple discrete sets. For such optimization problems, we study the performance of the Alternating Direction Method of Multipliers for Quantization (ADMM-Q) algorithm, which is a variant of the widely-used ADMM method applied to our discrete optimization problem. We establish the convergence of the iterates of ADMM-Q to certain stationary points. In addition, our results shows that the Lagrangian function of ADMM converges monotonically. To the best of our knowledge, this is the first analysis of an ADMM-type method for problems with discrete variables/constraints. Based on our theoretical insights, we develop a few variants of ADMM-Q that can handle inexact update rules, and have improved performance via the use of "soft projection" and "injecting randomness" to the algorithm. We empirically evaluate the efficacy of our proposed approaches on two problem: 1) solving quantized quadratic optimization problems and 2) training neural networks. Our numerical experiments shows that ADMM-Q outperforms other competing algorithms.
更多查看译文
关键词
quantization,multipliers,direction method
AI 理解论文
溯源树
样例
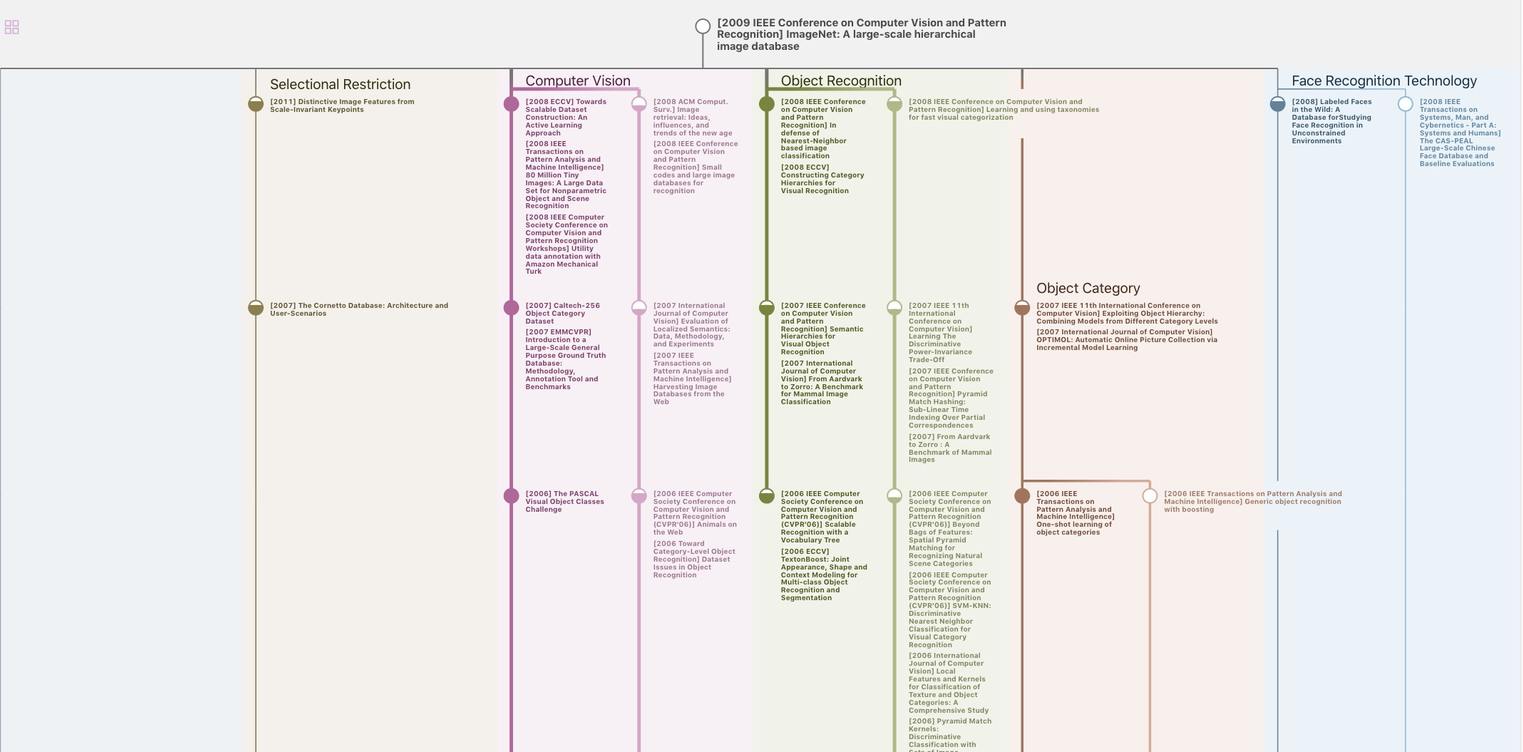
生成溯源树,研究论文发展脉络
Chat Paper
正在生成论文摘要