High-Energy Density Hohlraum Design Using Forward And Inverse Deep Neural Networks
PHYSICS LETTERS A(2021)
摘要
We present the results of a study where we use machine learning to enhance hohlraum design for opacity measurement experiments. Opacity experiments on laser facilities use hohlraums, which, when their interior walls are illuminated by the National Ignition Facility (NIF) lasers, produce a high radiation flux that heats a central sample to a temperature that is constant over a measurement time window. Given a baseline hohlraum design and a computational model, we train a deep neural network to predict the time evolution of the radiation temperature as measured by the Dante diagnostic. This enables us to rapidly explore design space and determine the effect of adjusting design parameters. We also construct an "inverse" machine learning model that predicts the design parameters given a desired time history of radiation temperature. Calculations using the machine learning model demonstrate that improved performance over the baseline hohlraum could reduce sensitivities and uncertainties in experimental opacity measurements. (C) 2021 Elsevier B.V. All rights reserved.
更多查看译文
关键词
High energy density physics, Opacity measurements, Hohlraum modeling, Scientific machine learning
AI 理解论文
溯源树
样例
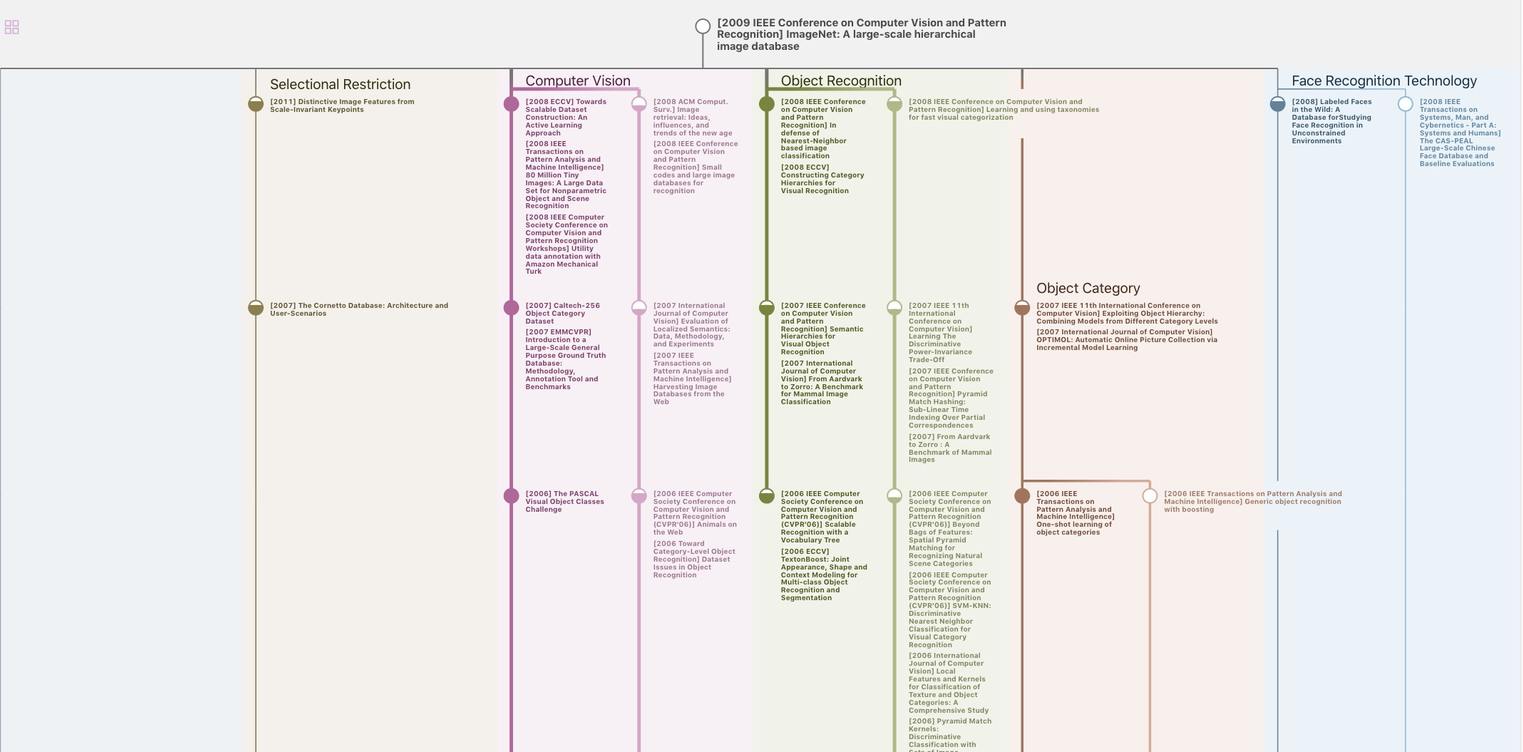
生成溯源树,研究论文发展脉络
Chat Paper
正在生成论文摘要