Online Disease Diagnosis with Inductive Heterogeneous Graph Convolutional Networks
the web conference(2021)
摘要
ABSTRACT We propose a Healthcare Graph Convolutional Network (HealGCN) to offer disease self-diagnosis service for online users based on Electronic Healthcare Records (EHRs). Two main challenges are focused in this paper for online disease diagnosis: (1) serving cold-start users via graph convolutional networks and (2) handling scarce clinical description via a symptom retrieval system. To this end, we first organize the EHR data into a heterogeneous graph that is capable of modeling complex interactions among users, symptoms and diseases, and tailor the graph representation learning towards disease diagnosis with an inductive learning paradigm. Then, we build a disease self-diagnosis system with a corresponding EHR Graph-based Symptom Retrieval System (GraphRet) that can search and provide a list of relevant alternative symptoms by tracing the predefined meta-paths. GraphRet helps enrich the seed symptom set through the EHR graph when confronting users with scarce descriptions, hence yield better diagnosis accuracy. At last, we validate the superiority of our model on a large-scale EHR dataset.
更多查看译文
关键词
disease diagnosis, graph neural network, online healthcare service
AI 理解论文
溯源树
样例
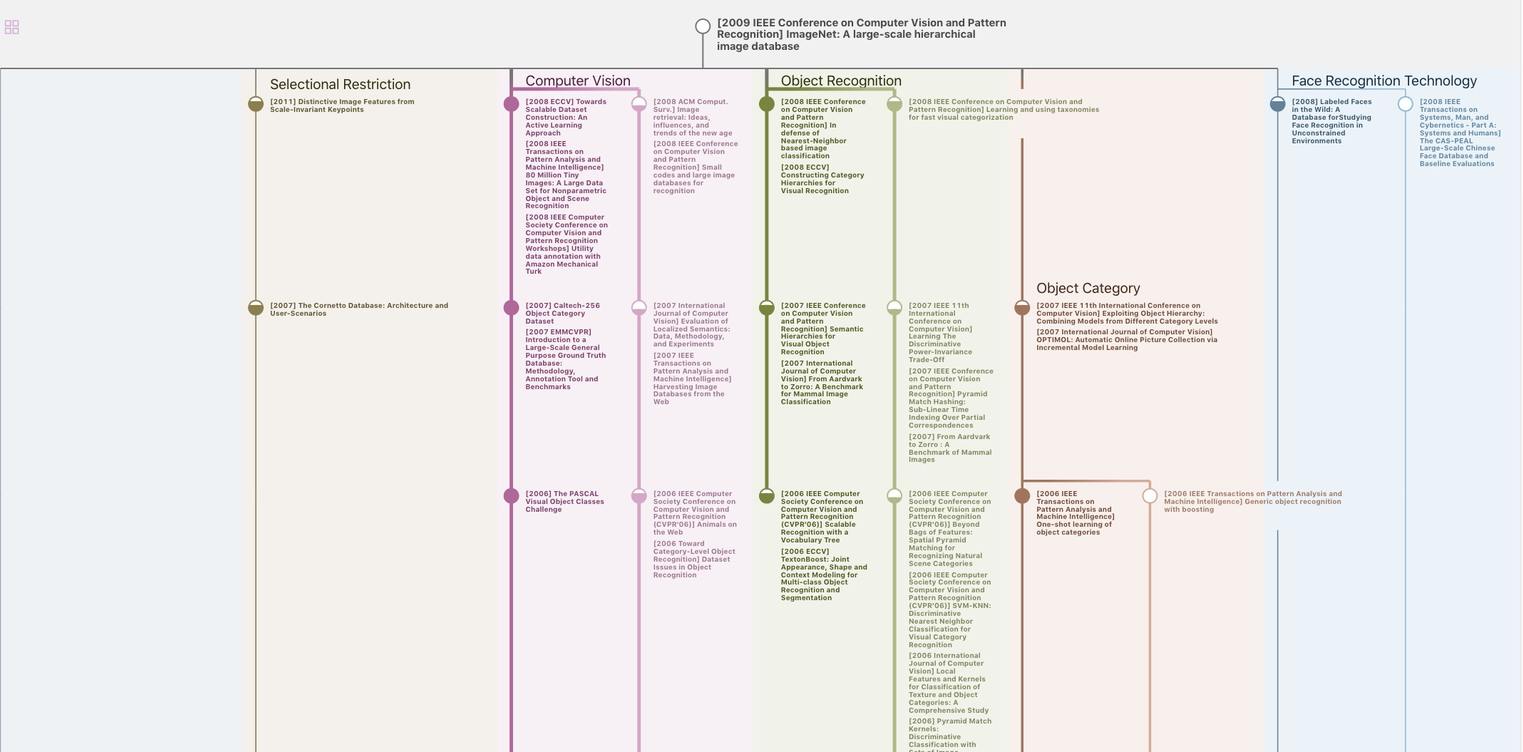
生成溯源树,研究论文发展脉络
Chat Paper
正在生成论文摘要