S-SGD: Symmetrical Stochastic Gradient Descent with Weight Noise Injection for Reaching Flat Minima
arxiv(2020)
摘要
The stochastic gradient descent (SGD) method is most widely used for deep neural network (DNN) training. However, the method does not always converge to a flat minimum of the loss surface that can demonstrate high generalization capability. Weight noise injection has been extensively studied for finding flat minima using the SGD method. We devise a new weight-noise injection-based SGD method that adds symmetrical noises to the DNN weights. The training with symmetrical noise evaluates the loss surface at two adjacent points, by which convergence to sharp minima can be avoided. Fixed-magnitude symmetric noises are added to minimize training instability. The proposed method is compared with the conventional SGD method and previous weight-noise injection algorithms using convolutional neural networks for image classification. Particularly, performance improvements in large batch training are demonstrated. This method shows superior performance compared with conventional SGD and weight-noise injection methods regardless of the batch-size and learning rate scheduling algorithms.
更多查看译文
关键词
symmetrical stochastic gradient descent,flat minima,weight noise injection,s-sgd
AI 理解论文
溯源树
样例
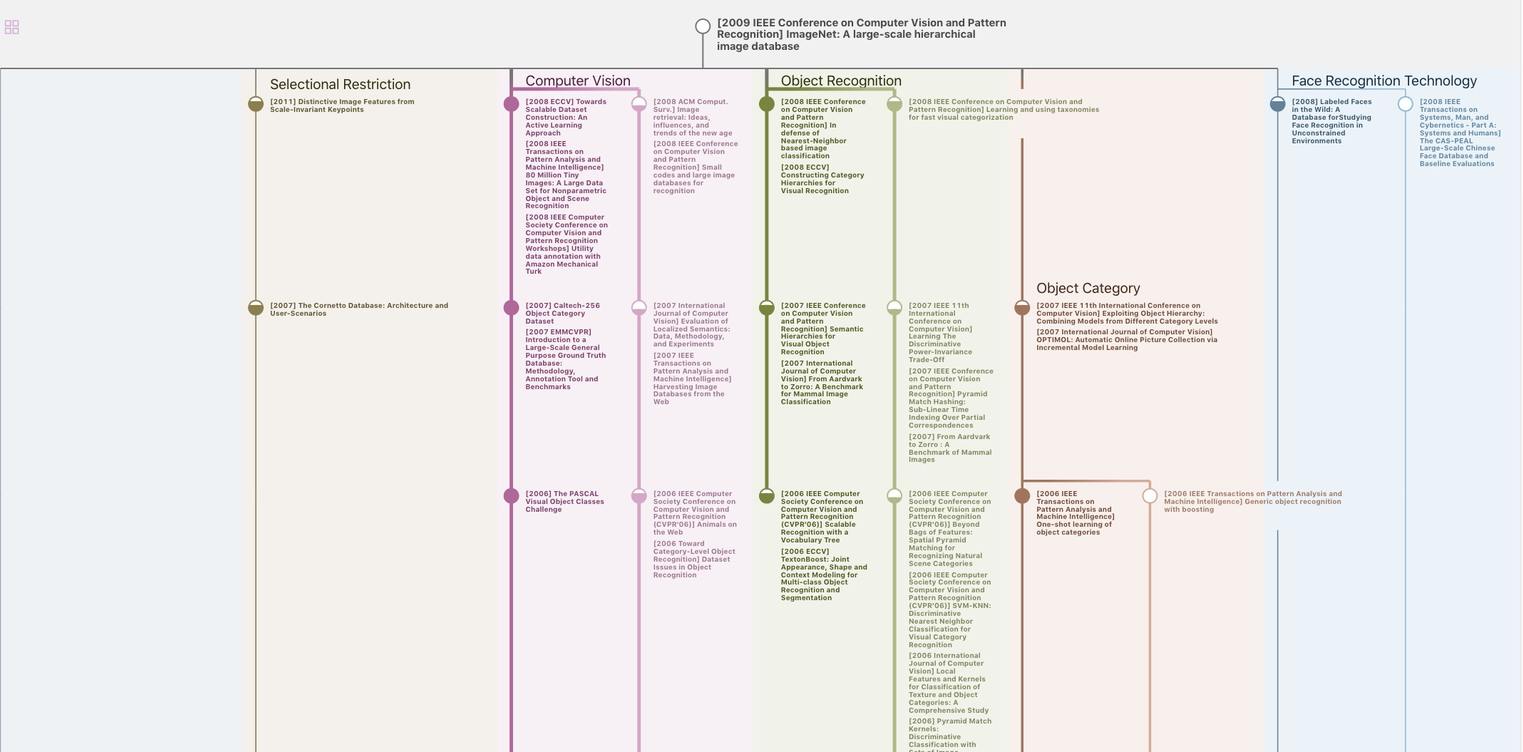
生成溯源树,研究论文发展脉络
Chat Paper
正在生成论文摘要