Sanitizing Synthetic Training Data Generation for Question Answering over Knowledge Graphs
ICTIR '20: The 2020 ACM SIGIR International Conference on the Theory of Information Retrieval Virtual Event Norway September, 2020(2020)
Abstract
Synthetic data generation is important to training and evaluating neural models for question answering over knowledge graphs. The quality of the data and the partitioning of the datasets into training, validation and test splits impact the performance of the models trained on this data. If the synthetic data generation depends on templates, as is the predominant approach for this task, there may be a leakage of information via a shared basis of templates across data splits if the partitioning is not performed hygienically. This paper investigates the extent of such information leakage across data splits, and the ability of trained models to generalize to test data when the leakage is controlled. We find that information leakage indeed occurs and that it affects performance. At the same time, the trained models do generalize to test data under the sanitized partitioning presented here. Importantly, these findings extend beyond the particular flavor of question answering task we studied and raise a series of difficult questions around template-based synthetic data generation that will necessitate additional research.
MoreTranslated text
Key words
knowledge graphs
AI Read Science
Must-Reading Tree
Example
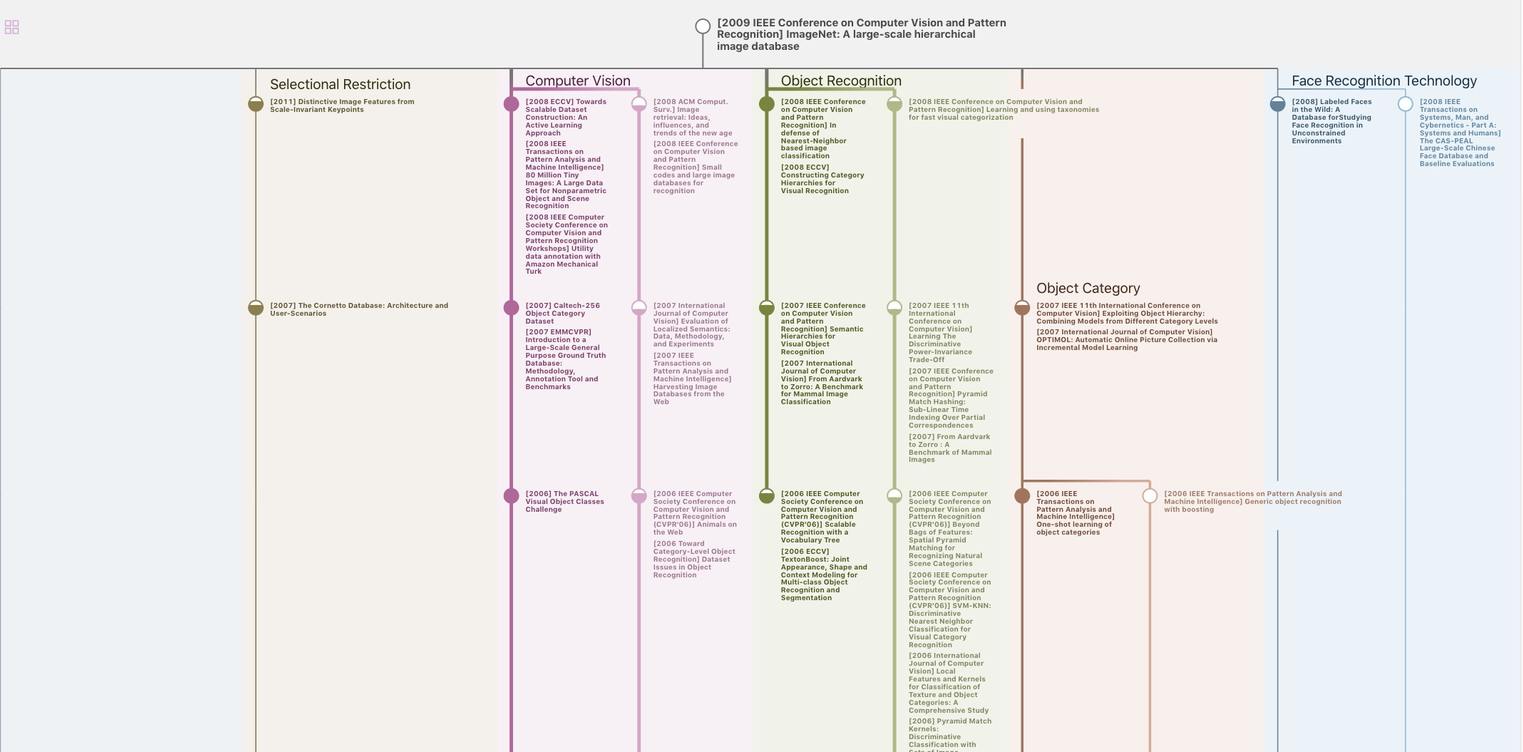
Generate MRT to find the research sequence of this paper
Chat Paper
Summary is being generated by the instructions you defined