Search Result Diversification with Guarantee of Topic Proportionality
ICTIR '20: The 2020 ACM SIGIR International Conference on the Theory of Information Retrieval Virtual Event Norway September, 2020(2020)
摘要
Search result diversification based on topic proportionality considers a document as a bag of weighted topics and aims to reorder or down-sample a ranked list in a way that maintains topic proportionality. The goal is to show the topic distribution from an ambiguous query at all points in the revised list, hoping to satisfy all users in expectation. One effective approach, PM-2, greedily selects the best topic that maintains proportionality at each ranking position and then selects the document that best represents that topic. From a theoretical perspective, this approach does not provide any guarantee that topic proportionality holds in the small ranked list. Moreover, this approach does not take query-document relevance into account. We propose a Linear Programming (LP) formulation, LP-QL, that maintains topic proportionality and simultaneously maximizes relevance. We show that this approach satisfies topic proportionality constraints in expectation. Empirically, it achieves a 5.5% performance gain (significant) in terms of alpha-NDCG compared to PM-2 when we use LDA as the topic modelling approach. Furthermore, we propose LP-PM-2 that integrates the solution of LP-QL with PM-2. LP-PM-2 achieves 3.2% performance gain (significant) over PM-2 in terms of alpha-NDCG with term based topic modeling approach. All of our experiments are based on a popular web document collection, ClueWeb09 Category B, and the queries are taken from TREC Web Track's diversity task.
更多查看译文
AI 理解论文
溯源树
样例
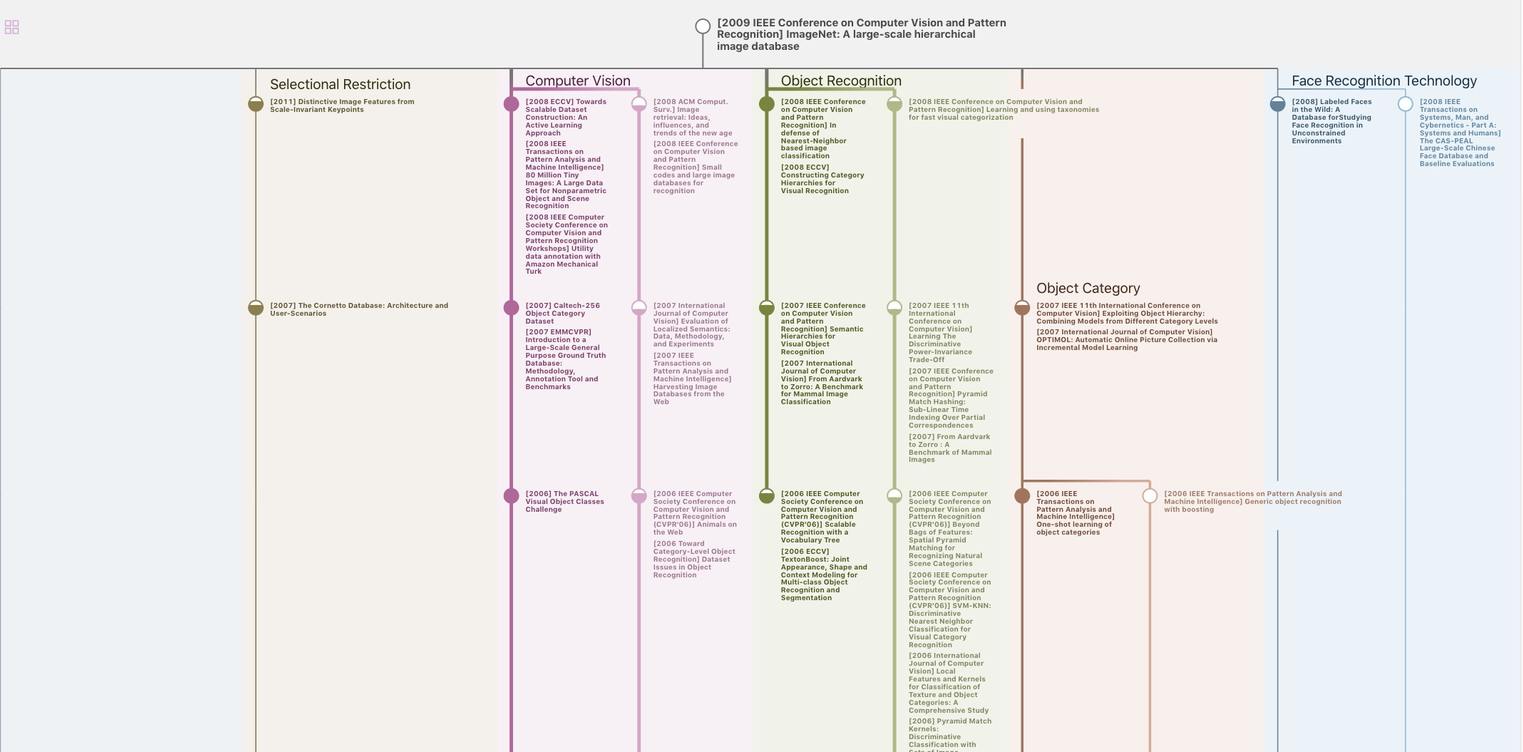
生成溯源树,研究论文发展脉络
Chat Paper
正在生成论文摘要