Training Data Optimization for Pairwise Learning to Rank
ICTIR '20: The 2020 ACM SIGIR International Conference on the Theory of Information Retrieval Virtual Event Norway September, 2020(2020)
摘要
This paper studies data optimization for Learning to Rank (LtR), by dropping training labels to increase ranking accuracy. Our work is inspired by data dropout, showing some training data do not positively influence learning and are better dropped out, despite a common belief that a larger training dataset is beneficial. Our main contribution is to extend this intuition for noisy- and semi- supervised LtR scenarios: some human annotations can be noisy or out-of-date, and so are machine-generated pseudo-labels in semi- supervised scenarios. Dropping out such unreliable labels would contribute to both scenarios. State-of-the-arts propose Influence Function (IF) for estimating how each training instance affects learn- ing, and we identify and overcome two challenges specific to LtR. 1) Non-convex ranking functions violate the assumptions required for the robustness of IF estimation. 2) The pairwise learning of LtR incurs quadratic estimation overhead. Our technical contributions are addressing these challenges: First, we revise estimation and data optimization to accommodate reduced reliability; Second, we devise a group-wise estimation, reducing cost yet keeping accuracy high. We validate the effectiveness of our approach in a wide range of ad-hoc information retrieval benchmarks and real-life search engine datasets in both noisy- and semi-supervised scenarios.
更多查看译文
AI 理解论文
溯源树
样例
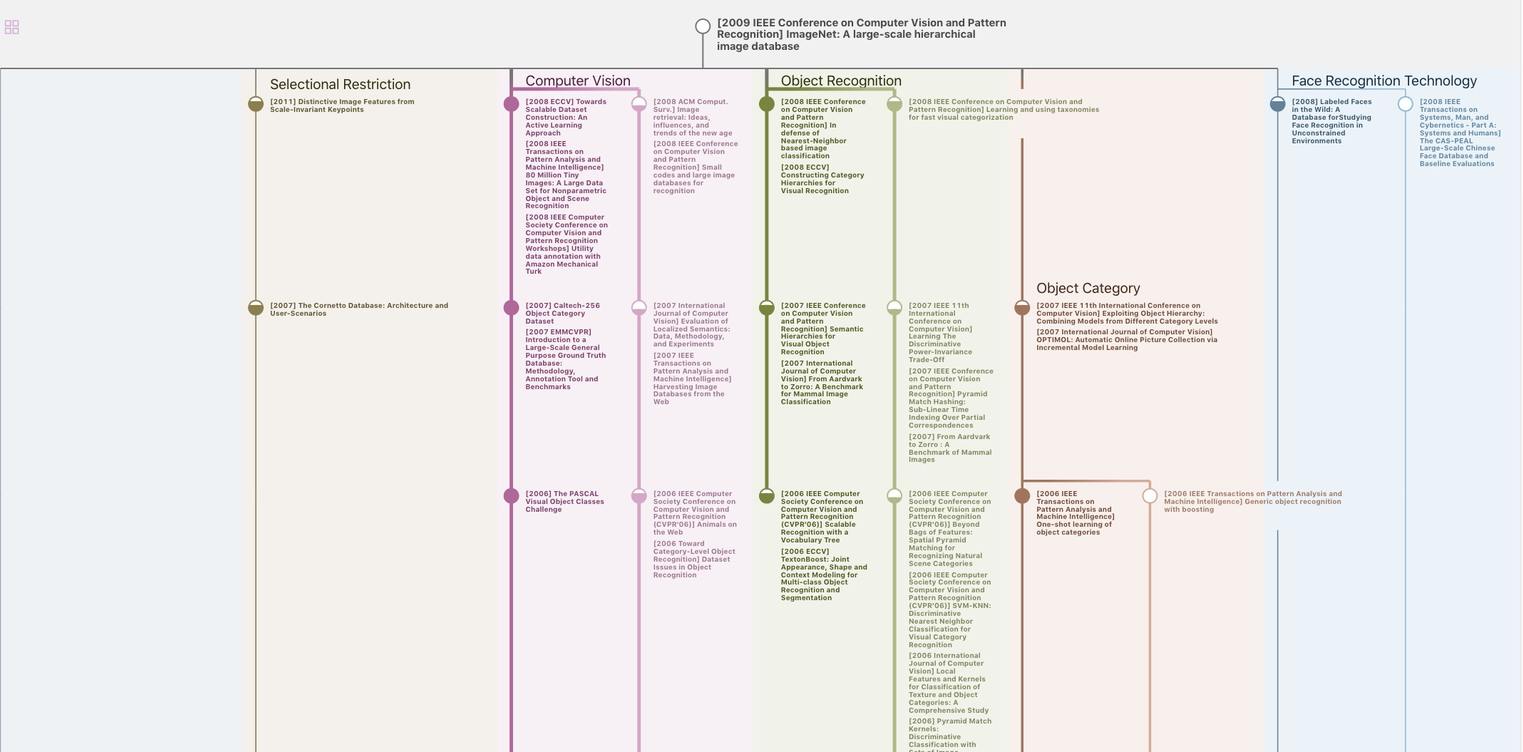
生成溯源树,研究论文发展脉络
Chat Paper
正在生成论文摘要