Composite Machine Learning Algorithm for Material Sourcing .
JOURNAL OF FORENSIC SCIENCES(2020)
Abstract
This study developed a composite machine learning algorithm for attribution of materials of forensic interest (like ammonium nitrate) to original sources. k-nearest neighbor and random forest models were used for source elimination and classification, respectively, in a two-step, composite algorithm based on particle color, size/shape, and trace element concentration features. Novel approaches for simulation to supplement within-source reference features based on empirically measured multi-lot analyses, an improved hold-one-lot-out method for cross-validation, an assessment of the likelihood of the presence of a reference sample, fusion of the source probabilities from the respective classification models, and the calculation of metrics for assessing ensemble sourcing performance are described. Excellent sourcing predictions were obtained; the sourcing algorithm identified the correct source as the top choice 89% of the time, and the correct source was identified to be an average of 2.7 times more likely than the most likely incorrect source.
MoreTranslated text
Key words
forensic science,source attribution,k-nearest neighbor,multinomial logistic regression,random forest
AI Read Science
Must-Reading Tree
Example
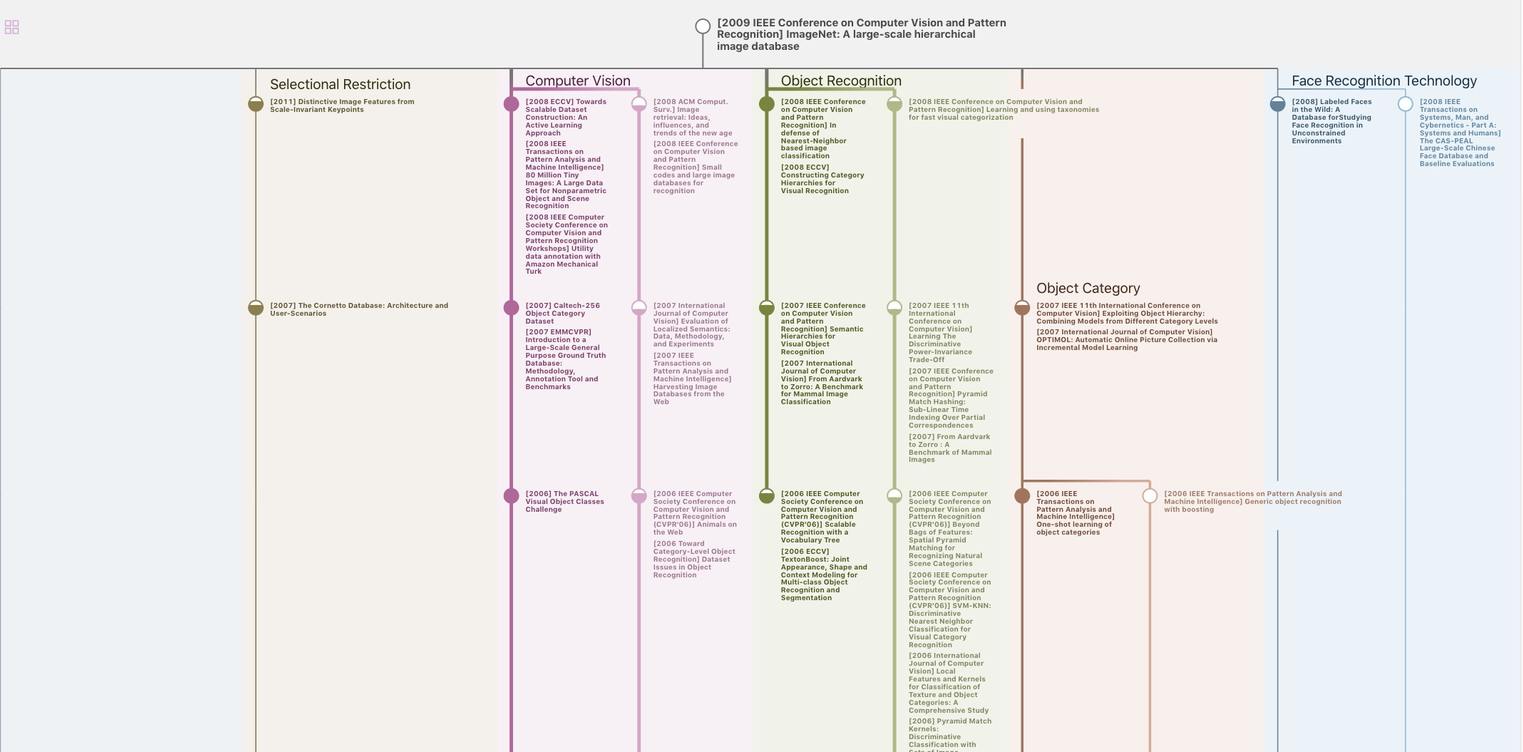
Generate MRT to find the research sequence of this paper
Chat Paper
Summary is being generated by the instructions you defined