Estimating crowd-worker's reliability with interval-valued labels to improve the quality of crowdsourced work.
SSCI(2021)
摘要
With inputs from human crowds, usually through the Internet, crowdsourcing has become a promising methodology in AI and machine learning for applications that require human knowledge. Researchers have recently proposed interval-valued labels (IVLs), instead of commonly used binary-valued ones, to manage uncertainty in crowdsourcing [19]. However, that work has not yet taken the crowd worker's reliability into consideration. Crowd workers usually come with various social and economic backgrounds, and have different levels of reliability. To further improve the overall quality of crowdsourcing with IVLs, this work presents practical methods that quantitatively estimate worker's reliability in terms of his/her correctness, confidence, stability, and predictability from his/her IVLs. With worker's reliability, this paper proposes two learning schemes: weighted interval majority voting (WIMV) and weighted preferred matching probability (WPMP). Computational experiments on sample datasets demonstrate that both WIMV and WPMP can significantly improve learning results in terms of higher precision, accuracy, and F-1-score than other methods.
更多查看译文
关键词
crowdsourcing,interval-valued label,worker's reliability,correctness,confidence,stability,predictability
AI 理解论文
溯源树
样例
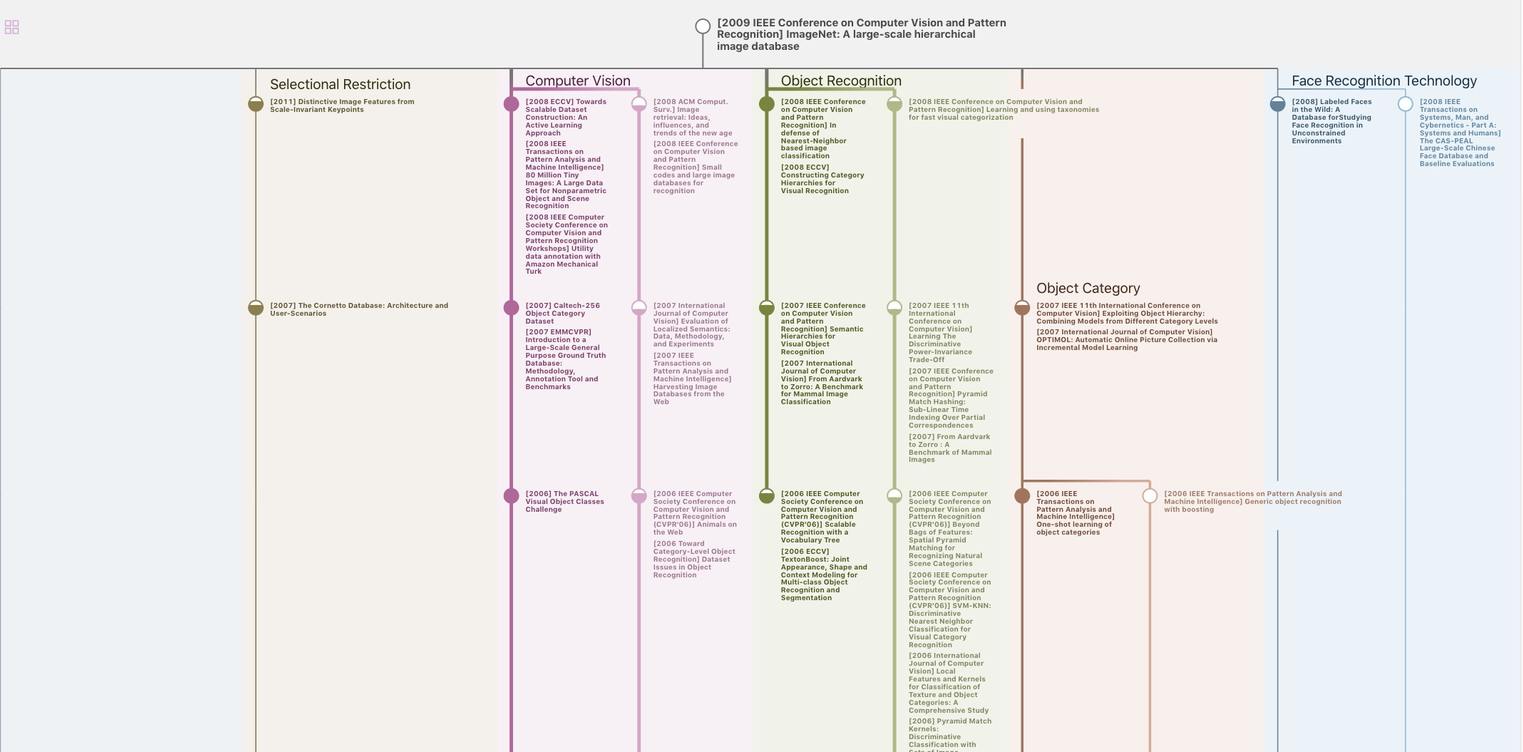
生成溯源树,研究论文发展脉络
Chat Paper
正在生成论文摘要