Unfolding-Model-Based Visualization: Theory, Method And Applications
JOURNAL OF MACHINE LEARNING RESEARCH(2021)
摘要
Multidimensional unfolding methods are widely used for visualizing item response data. Such methods project respondents and items simultaneously onto a low-dimensional Euclidian space, in which respondents and items are represented by ideal points, with person-person, item-item, and person-item similarities being captured by the Euclidian distances between the points. In this paper, we study the visualization of multidimensional unfolding from a statistical perspective. We cast multidimensional unfolding into an estimation problem, where the respondent and item ideal points are treated as parameters to be estimated. An estimator is then proposed for the simultaneous estimation of these parameters. Asymptotic theory is provided for the recovery of the ideal points, shedding lights on the validity of model-based visualization. An alternating projected gradient descent algorithm is proposed for the parameter estimation. We provide two illustrative examples, one on users' movie rating and the other on senate roll call voting.
更多查看译文
关键词
Multidimensional Unfolding, Data Visualization, Distance Matrix Completion, Item Response Data, Embedding
AI 理解论文
溯源树
样例
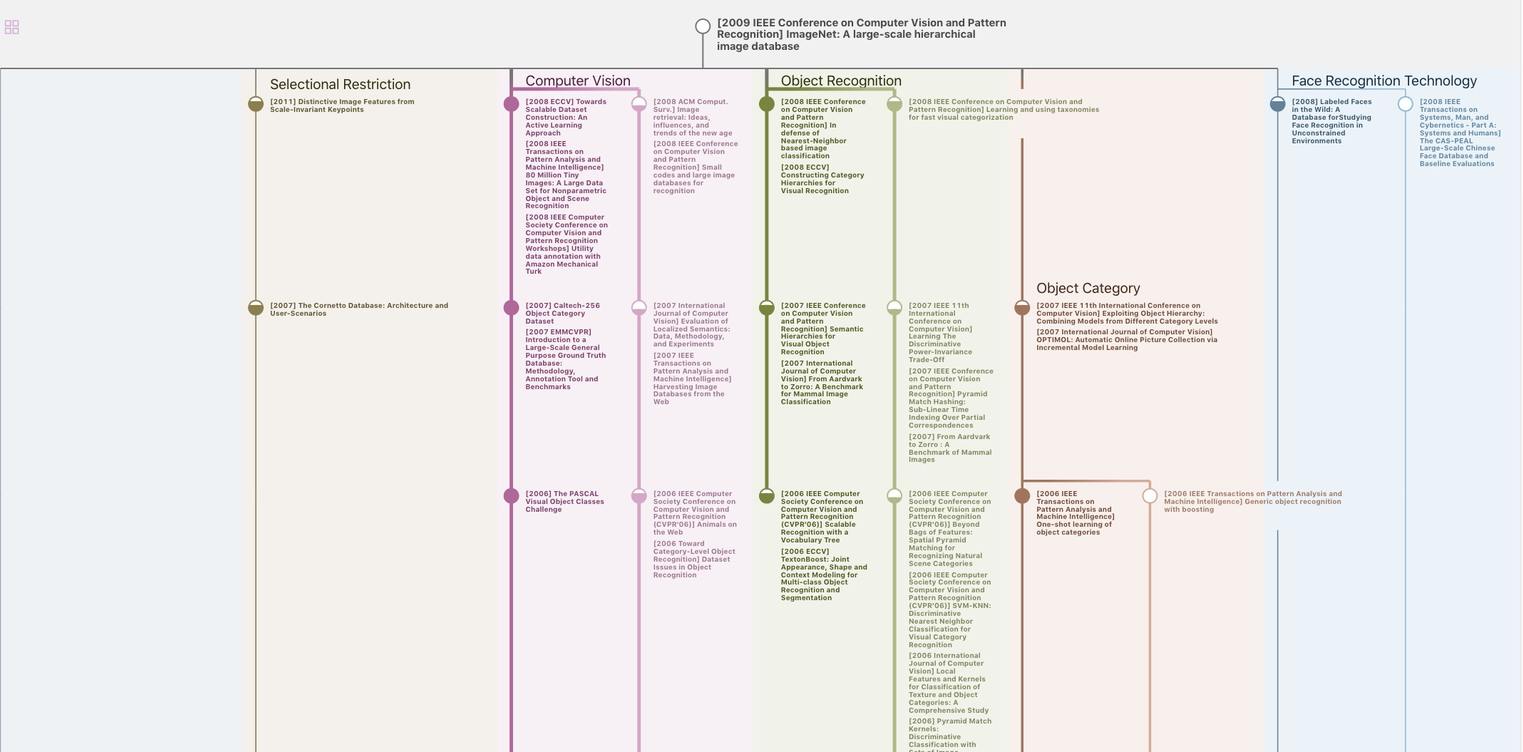
生成溯源树,研究论文发展脉络
Chat Paper
正在生成论文摘要