A Robust Score-Driven Filter for Multivariate Time Series
arxiv(2022)
摘要
A multivariate score-driven filter is developed to extract signals from noisy vector processes. By assuming that the conditional location vector from a multivariate Student's t distribution changes over time, we construct a robust filter which is able to overcome several issues that naturally arise when modeling heavy-tailed phenomena and, more in general, vectors of dependent non-Gaussian time series. We derive conditions for stationarity and invertibility and estimate the unknown parameters by maximum likelihood (ML). Strong consistency and asymptotic normality of the estimator are proved and the finite sample properties are illustrated by a Monte-Carlo study. From a computational point of view, analytical formulae are derived, which consent to develop estimation procedures based on the Fisher scoring method. The theory is supported by a novel empirical illustration that shows how the model can be effectively applied to estimate consumer prices from home scanner data.
更多查看译文
关键词
multivariate time series,time series,filter,score-driven
AI 理解论文
溯源树
样例
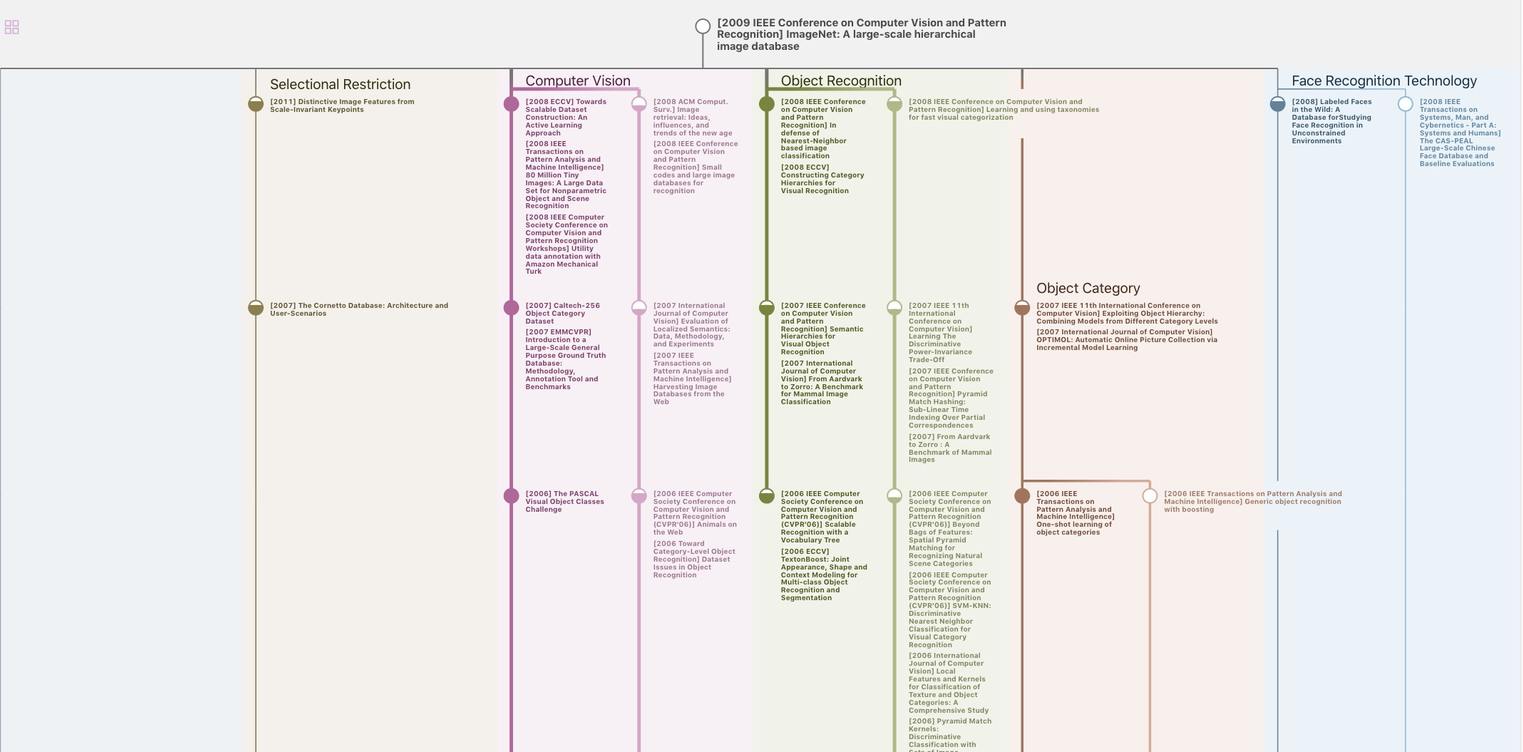
生成溯源树,研究论文发展脉络
Chat Paper
正在生成论文摘要