Real Image Super Resolution via Heterogeneous Model Ensemble Using GP-NAS.
arxiv(2020)
摘要
With advancement in deep neural network (DNN), recent state-of-the-art (SOTA) image superresolution (SR) methods have achieved impressive performance using deep residual network with dense skip connections. While these models perform well on benchmark dataset where low-resolution (LR) images are constructed from high-resolution (HR) references with known blur kernel, real image SR is more challenging when both images in the LR-HR pair are collected from real cameras. Based on existing dense residual networks, a Gaussian process based neural architecture search (GP-NAS) scheme is utilized to find candidate network architectures using a large search space by varying the number of dense residual blocks, the block size and the number of features. A suite of heterogeneous models with diverse network structure and hyperparameter are selected for model-ensemble to achieve outstanding performance in real image SR. The proposed method won the first place in all three tracks of the AIM 2020 Real Image Super-Resolution Challenge.
更多查看译文
关键词
Single image super resolution, Dense residual network, Neural architecture search
AI 理解论文
溯源树
样例
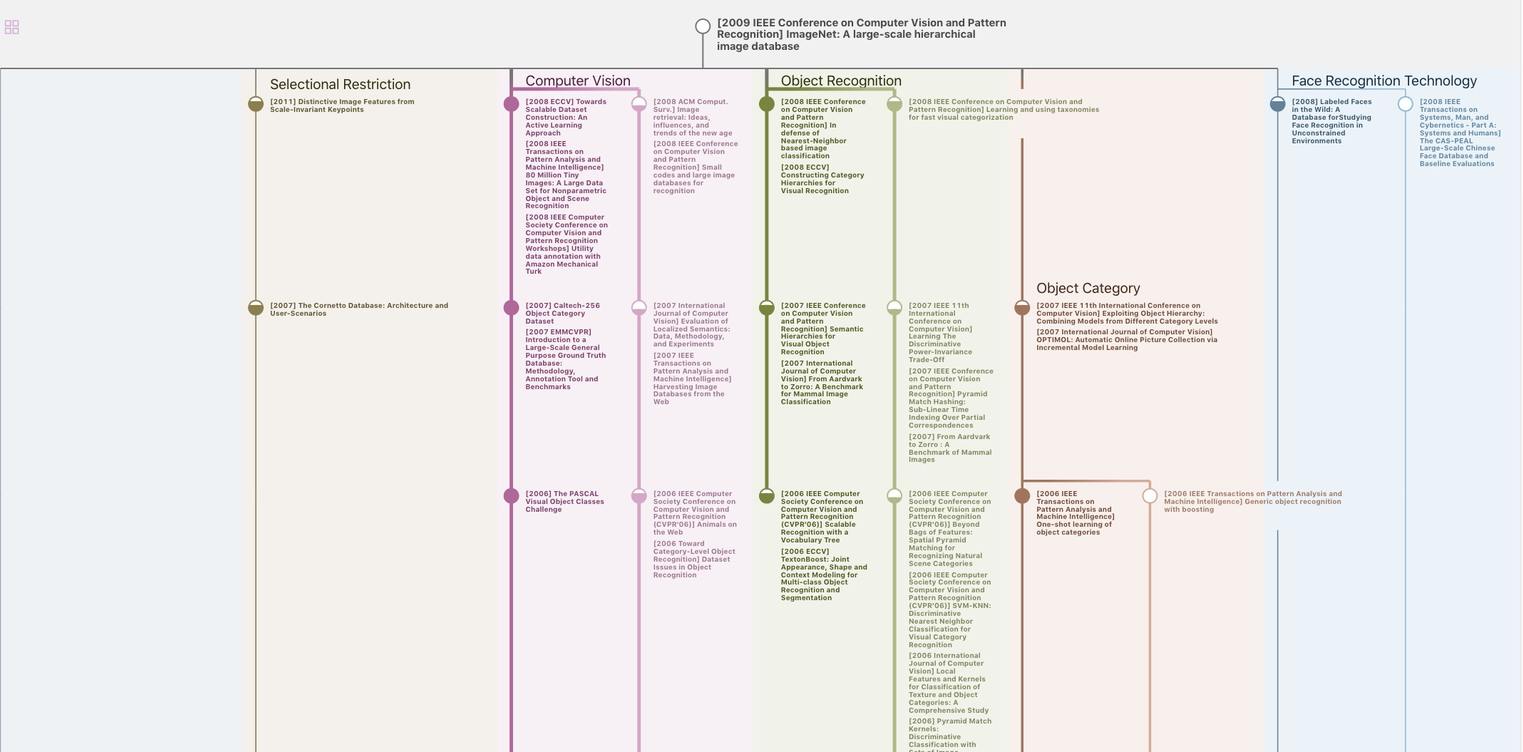
生成溯源树,研究论文发展脉络
Chat Paper
正在生成论文摘要