TransLoc: Transparent Indoor Localization with Uncertain Human Participation for Instant Delivery
MobiCom '20: The 26th Annual International Conference on Mobile Computing and Networking London United Kingdom September, 2020(2020)
摘要
Instant delivery is an important urban service in recent years because of the increasing demand. An important issue for delivery platforms is to keep updating the status of couriers especially the real-time locations, which is challenging when they are in an indoor environment. We argue the previous indoor localization techniques cannot be applied in the instant delivery scenario because they require extra deployed infrastructures and extensive labor work. In this work, we perform the couriers' indoor localization transparently in a predictive manner without extra actions of couriers by existing data from the platform including order progress reports and couriers' trajectories. Specifically, we present TransLoc to predict couriers' indoor locations by addressing two challenges including uncertain reporting behaviors and uncertain indoor mobility behaviors. Our key idea lies in two insights (i) couriers' behaviors are consistent in indoor/outdoor environments; (ii) localization, as a spatial inference problem, could be converted to a temporal inference problem. We evaluate TransLoc on 565 couriers from an instant delivery company, which improves baselines by at most 72%, and achieves a competitive result compared to a label-extensive approach. As a case study, we apply TransLoc to optimize the order dispatching strategy, which reduces the delivery time by 24%.
更多查看译文
AI 理解论文
溯源树
样例
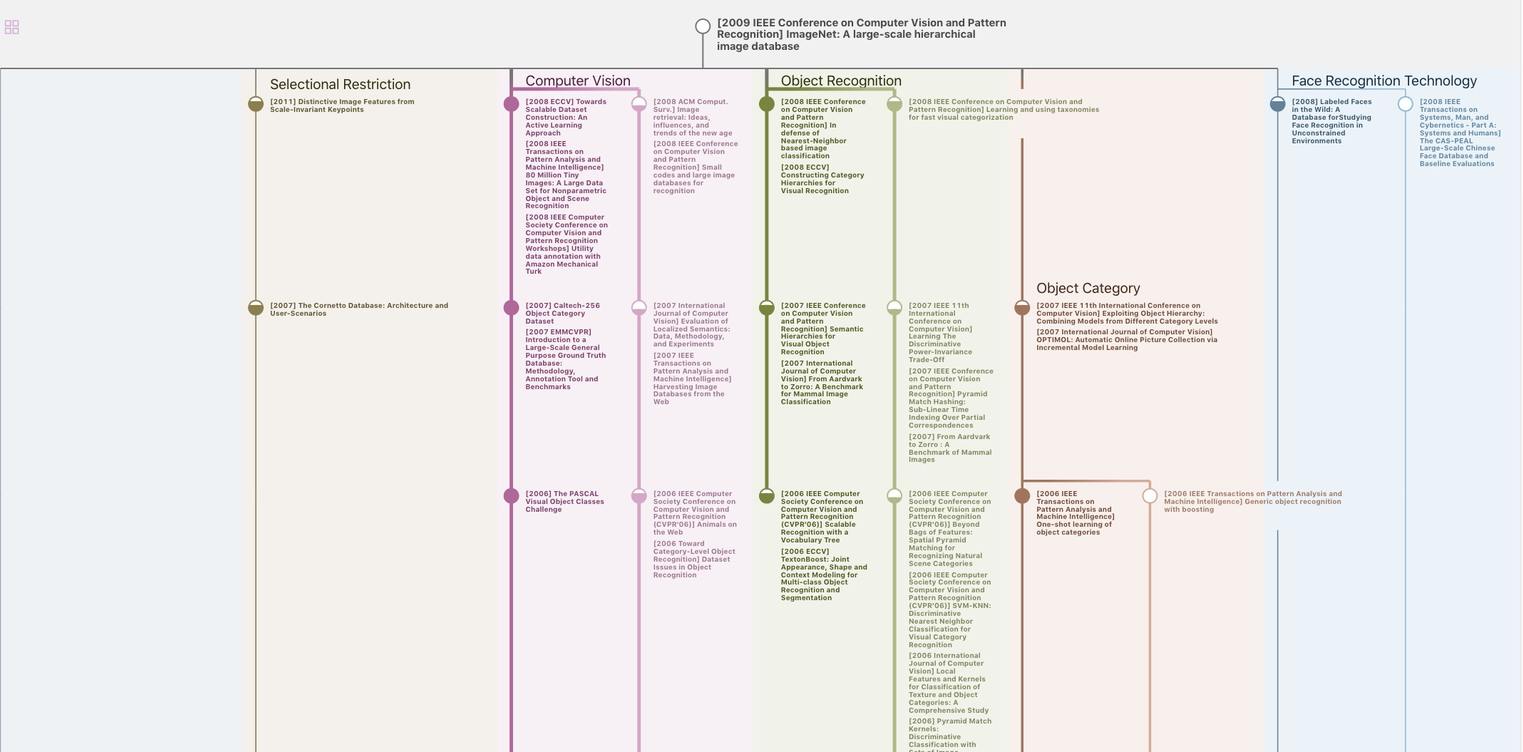
生成溯源树,研究论文发展脉络
Chat Paper
正在生成论文摘要