Predicting experimental electrophilicities from quantum and topological descriptors: A machine learning approach.
JOURNAL OF COMPUTATIONAL CHEMISTRY(2020)
摘要
In this paper, we assess the ability of various machine learning methods, either linear or non-linear, to efficiently predict Mayr's experimental scale for electrophilicity. To this aim, molecular and atomic descriptors rooted in conceptual density functional theory and in the quantum theory of atoms-in-molecules as well as topological features defined within graph theory were evaluated for a large set of molecules widely used in organic chemistry. State-of-the-art regression tools belonging to the support vector machines family and decision tree models were in particular considered and implemented. They afforded a promising predictive model, validating the use of such methodologies for the study of chemical reactivity.
更多查看译文
关键词
conceptual density functional theory,decision trees,electrophilicity,machine learning,nonlinear approaches,reactivity descriptors
AI 理解论文
溯源树
样例
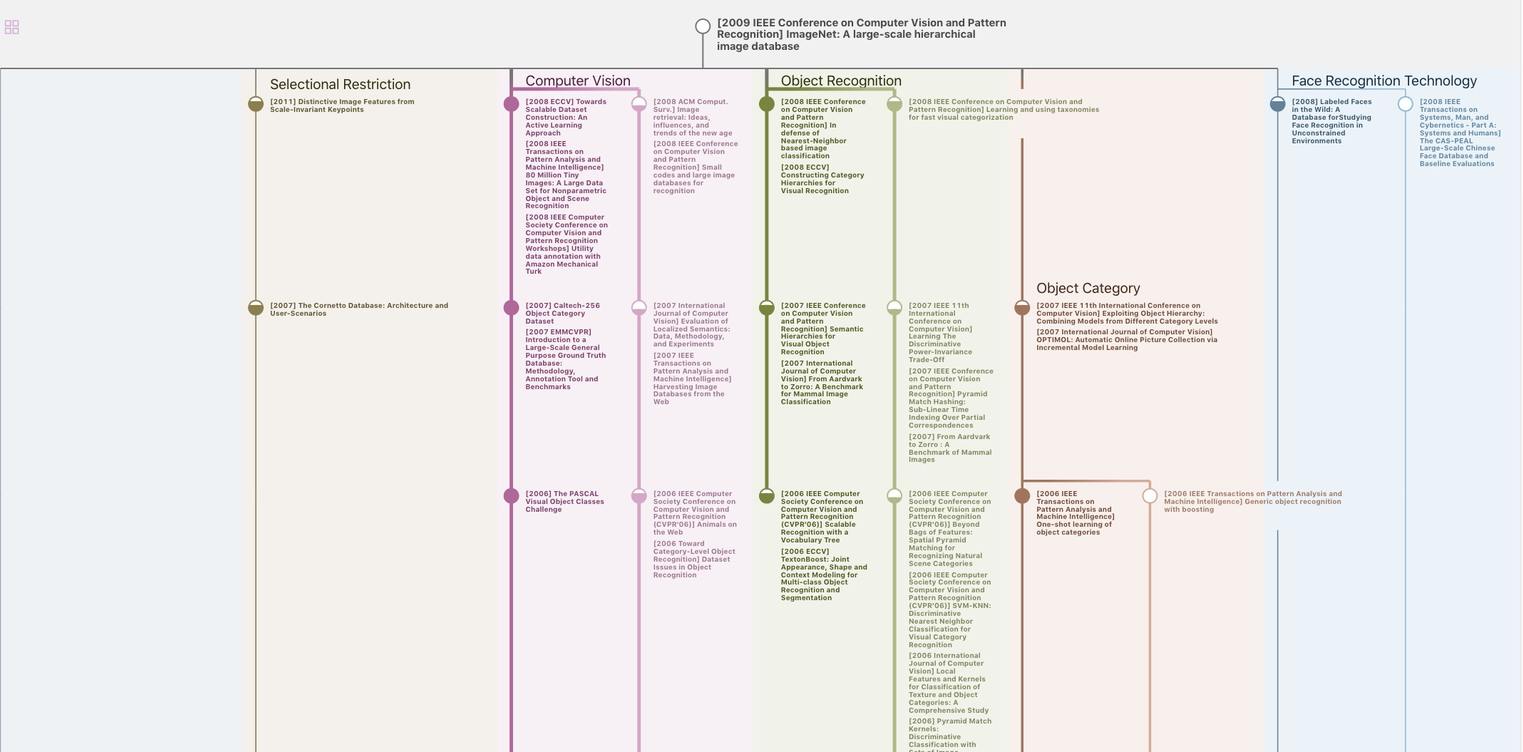
生成溯源树,研究论文发展脉络
Chat Paper
正在生成论文摘要