Graph Neural Network For 3d Classification Of Ambiguities And Optical Crosstalk In Scintillator-Based Neutrino Detectors
PHYSICAL REVIEW D(2021)
摘要
Deep-learning tools are being used extensively in high energy physics and are becoming central in the reconstruction of neutrino interactions in particle detectors. In this work, we report on the performance of a graph neural network in assisting with particle set event reconstruction. The three-dimensional reconstruction of particle tracks produced in neutrino interactions can be subject to ambiguities due to high multiplicity signatures in the detector or leakage of signal between neighboring active detector volumes. Graph neural networks potentially have the capability of identifying all these features to boost the reconstruction performance. As an example case study, we tested a graph neural network, inspired by the GraphSAGE algorithm, on a novel 3D-granular plastic-scintillator detector, that will be used to upgrade the near detector of the T2K experiment. The developed neural network has been trained and tested on diverse neutrino interaction samples, showing very promising results: the classification of particle track voxels produced in the detector can be done with efficiencies and purities of 94%-96% per event and most of the ambiguities can be identified and rejected, while being robust against systematic effects.
更多查看译文
关键词
neutrino detectors,graph neural network,3d classification,optical crosstalk,scintillator-based
AI 理解论文
溯源树
样例
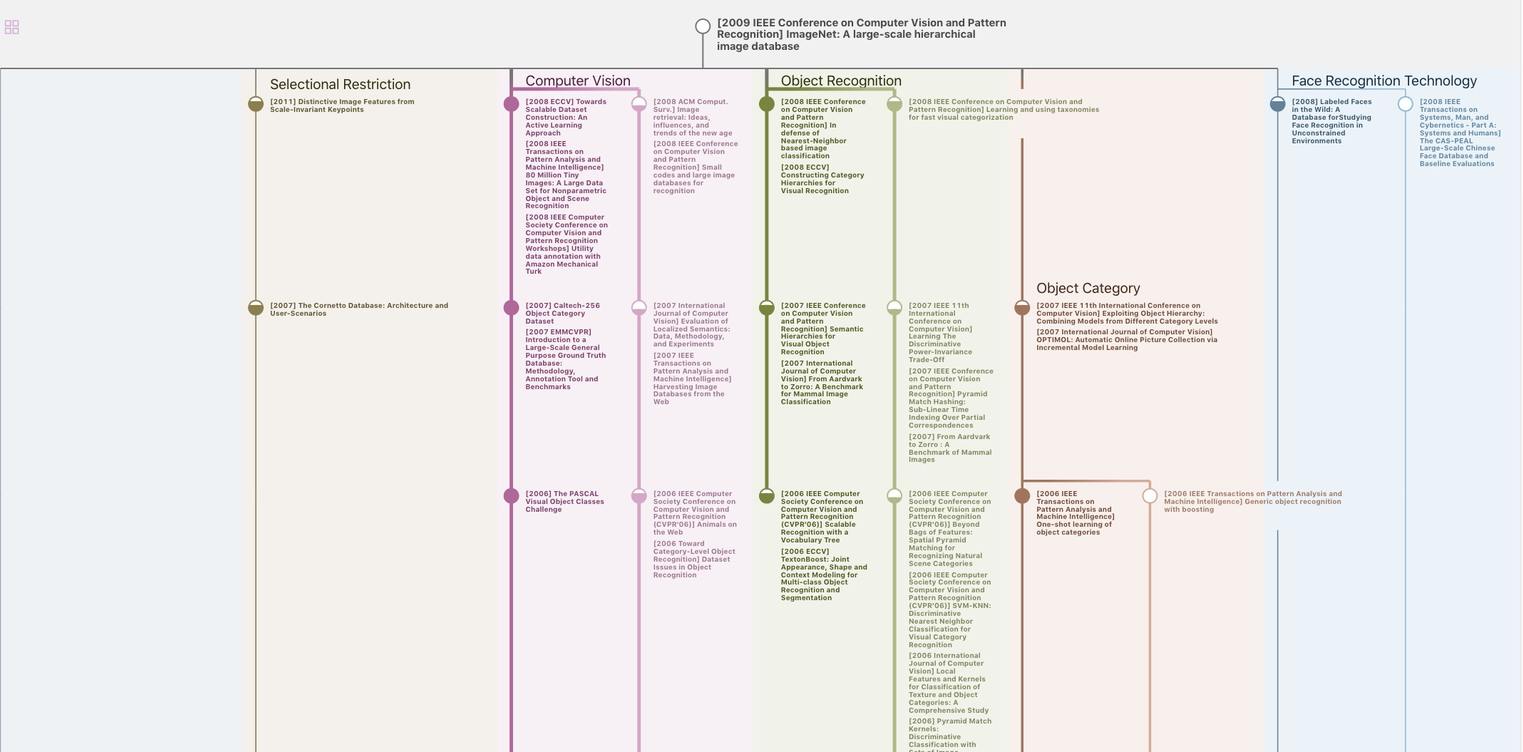
生成溯源树,研究论文发展脉络
Chat Paper
正在生成论文摘要