Direct Volume Rendering With Nonparametric Models Of Uncertainty
IEEE TRANSACTIONS ON VISUALIZATION AND COMPUTER GRAPHICS(2021)
摘要
We present a nonparametric statistical framework for the quantification, analysis, and propagation of data uncertainty in direct volume rendering (DVR). The state-of-the-art statistical DVR framework allows for preserving the transfer function (TF) of the ground truth function when visualizing uncertain data; however, the existing framework is restricted to parametric models of uncertainty. In this paper, we address the limitations of the existing DVR framework by extending the DVR framework for nonparametric distributions. We exploit the quantile interpolation technique to derive probability distributions representing uncertainty in viewing-ray sample intensities in closed form, which allows for accurate and efficient computation. We evaluate our proposed nonparametric statistical models through qualitative and quantitative comparisons with the mean-field and parametric statistical models, such as uniform and Gaussian, as well as Gaussian mixtures. In addition, we present an extension of the state-of-the-art rendering parametric framework to 2D TFs for improved DVR classifications. We show the applicability of our uncertainty quantification framework to ensemble, downsampled, and bivariate versions of scalar field datasets.
更多查看译文
关键词
Uncertainty, Data visualization, Interpolation, Probability density function, Rendering (computer graphics), Two dimensional displays, Pipelines, Volumes, uncertainty, nonparametric, 2D transfer function
AI 理解论文
溯源树
样例
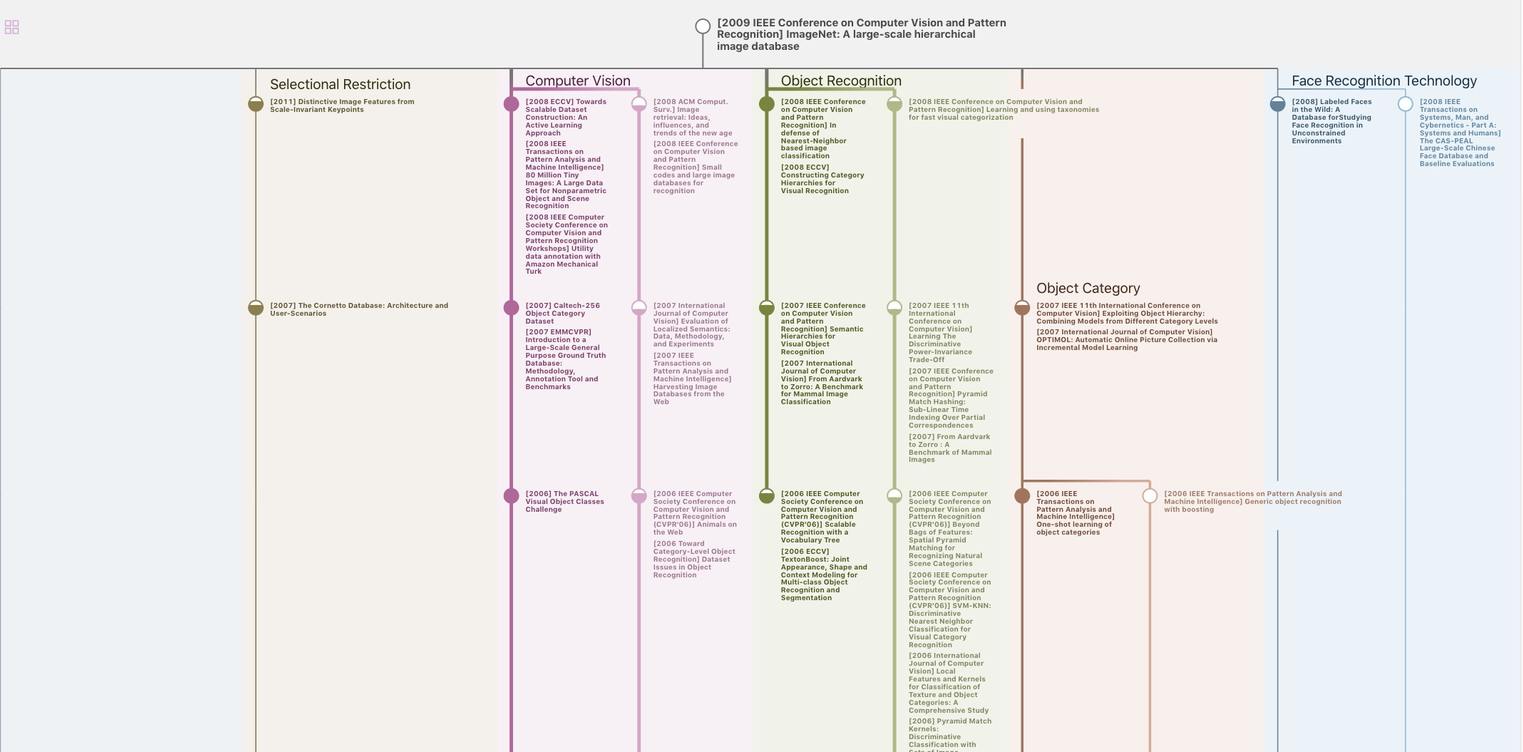
生成溯源树,研究论文发展脉络
Chat Paper
正在生成论文摘要