I-Aid: Identifying Actionable Information From Disaster-Related Tweets
IEEE ACCESS(2021)
摘要
Social media plays a significant role in disaster management by providing valuable data about affected people, donations, and help requests. Recent studies highlight the need to filter information on social media into fine-grained content labels. However, identifying useful information from massive amounts of social media posts during a crisis is a challenging task. In this paper, we propose I-AID, a multimodel approach to automatically categorize tweets into multi-label information types and filter critical information from the enormous volume of social media data. I-AID incorporates three main components: i) a BERT-based encoder to capture the semantics of a tweet and represent as a low-dimensional vector, ii) a graph attention network (GAT) to apprehend correlations between tweets' words/entities and the corresponding information types, and iii) a Relation Network as a learnable distance metric to compute the similarity between tweets and their corresponding information types in a supervised way. We conducted several experiments on two real publicly-available datasets. Our results indicate that I-AID outperforms state-of-the-art approaches in terms of weighted average F1 score by +6% and +4% on the TREC-IS dataset and COVID-19 Tweets, respectively.
更多查看译文
关键词
Measurement, Bit error rate, Social networking (online), Task analysis, Correlation, Vocabulary, Semantics, Crisis information, contextualized text embedding, social media analysis, graph attention network, meta-learning
AI 理解论文
溯源树
样例
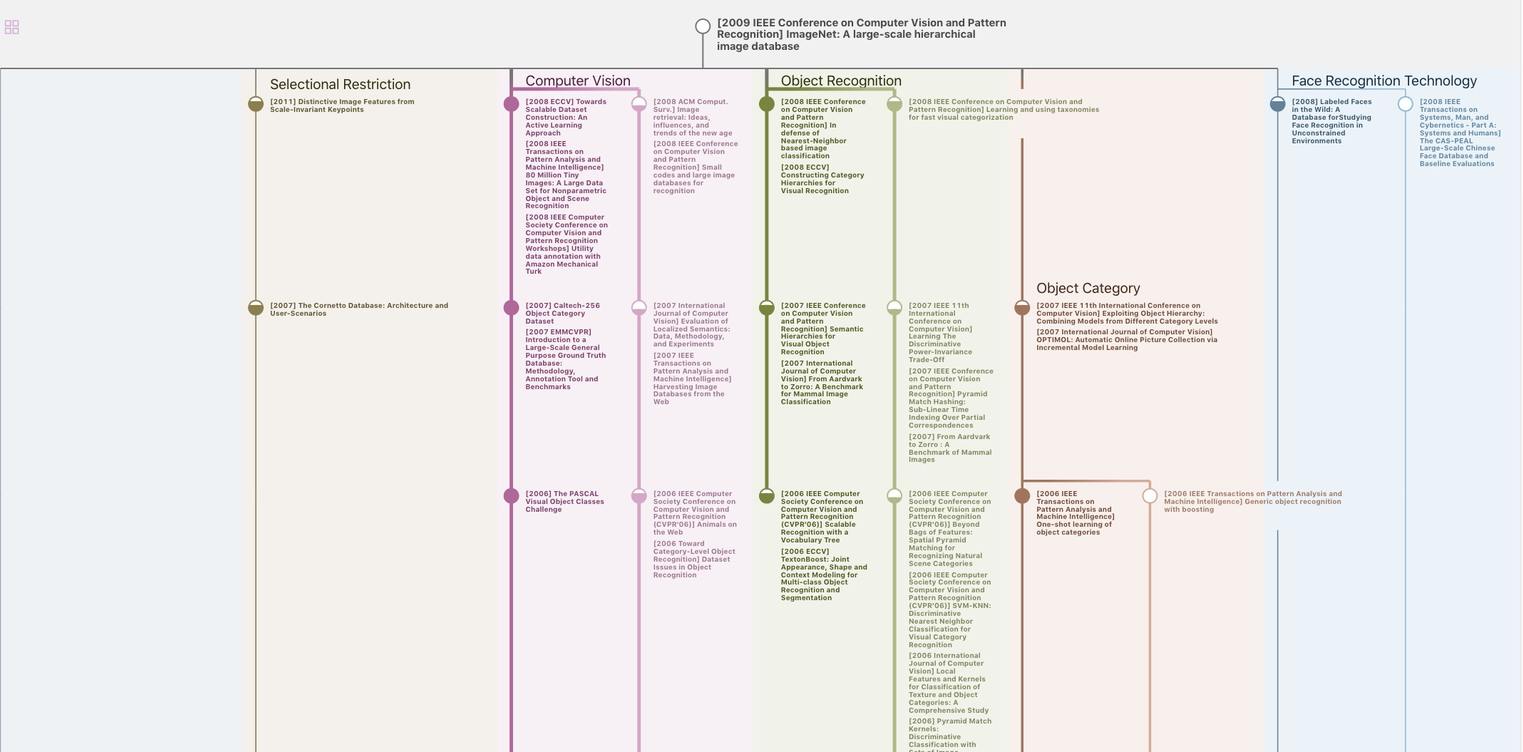
生成溯源树,研究论文发展脉络
Chat Paper
正在生成论文摘要