Hierarchical fusion of common sense knowledge and classifier decisions for answer selection in community question answering
Neural Networks(2020)
摘要
The goal of answer selection is to select the most applicable answers from an answer candidate pool. It plays an essential role in numerous applications in information retrieval (IR) and natural language processing (NLP). In this paper, we introduce a novel Knowledge-enhanced Hierarchical Attention mechanism for Answer Selection (KHAAS), which fully exploits the common sense knowledge from knowledge bases (KBs) and input textual information. Specifically, we first devise a three-stage knowledge-enhanced hierarchical attention mechanism, including the word-level attention, the phrase-level attention, and the document-level attention to learn the fact-aware intra-document features within questions and answers by fusing the knowledge from both the question/answer and KB. Hence, we can leverage the semantic compositionality of the question/answer and learn more holistic knowledge-enhanced intra-document features of the question/answer at three levels of granularity. Second, after obtaining the knowledge-enhanced question and answer representations, we employ a multi-perspective co-attention network to learn the complex inter-document relationships between the question and answer representations from different representation subspaces, which can capture the interactive semantics of the question and answer representations at three levels. Finally, we propose an adaptive decision fusion method to learn a more effective and robust ensemble answer selection model by adaptively combining multiple classifiers learned with different levels of features. Experimental results on three large-scale answer selection datasets demonstrate that KHAAS consistently outperforms the compared methods.
更多查看译文
关键词
Answer selection,Common sense knowledge,Hierarchical attention,Semantic compositionality
AI 理解论文
溯源树
样例
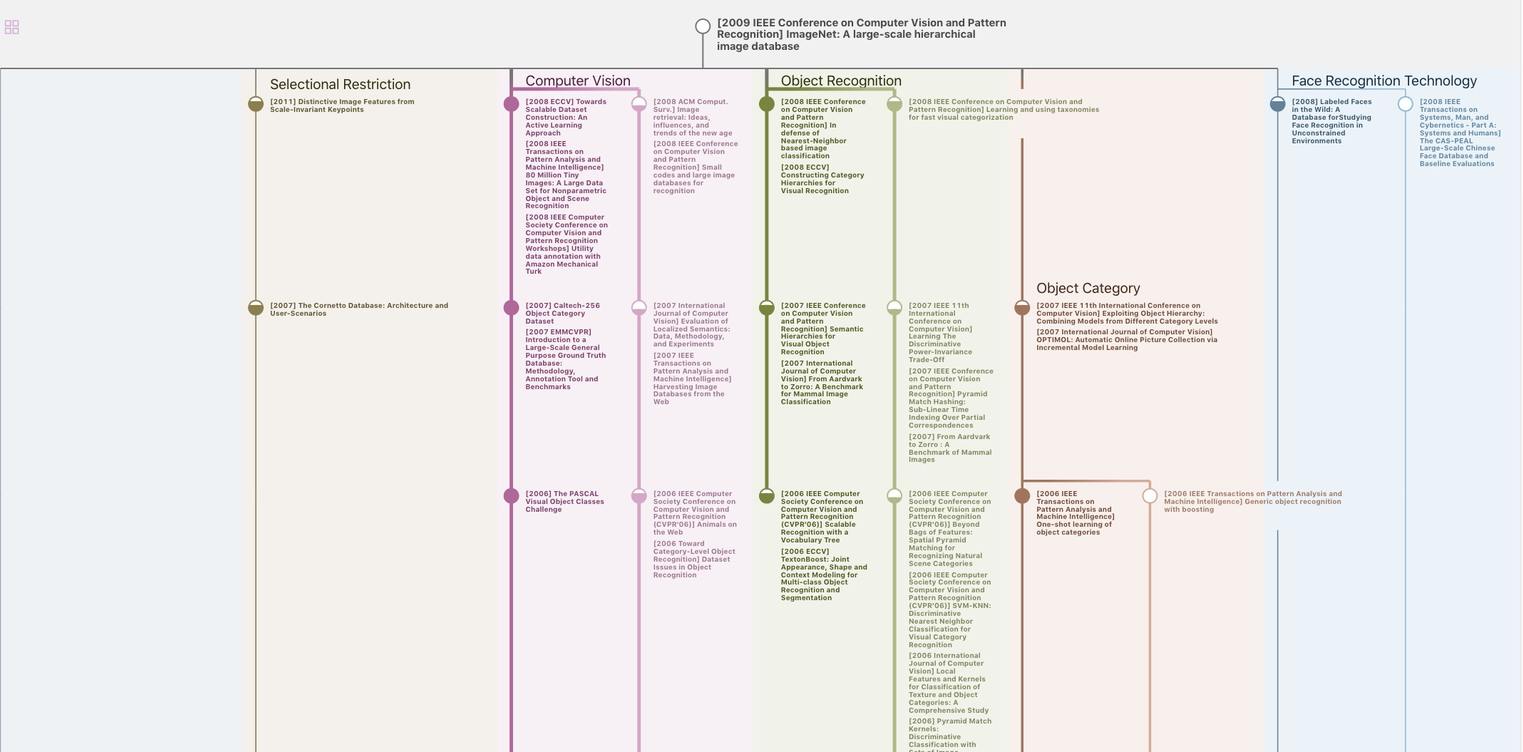
生成溯源树,研究论文发展脉络
Chat Paper
正在生成论文摘要