Data-driven adaptive modeling method for industrial processes and its application in flotation reagent control
ISA Transactions(2021)
摘要
In real industrial processes, new process “excitation” patterns that largely deviate from previously collected training data will appear due to disturbances caused by process inputs. To reduce model mismatch, it is important for a data-driven process model to adapt to new process “excitation” patterns. Although efforts have been devoted to developing adaptive process models to deal with this problem, few studies have attempted to develop an adaptive process model that can incrementally learn new process “excitation” patterns without performance degradation on old patterns. In this study, efforts are devoted to enabling data-driven process models with incremental learning ability. First, a novel incremental learning method is proposed for process model updating. Second, an adaptive neural network process model is developed based on the novel incremental learning method. Third, a nonlinear model predictive control based on the adaptive process model is implemented and applied for flotation reagent control. Experiments based on historical data provide evidence that the newly developed adaptive process model can accommodate new process “excitation” patterns and preserve its performance on old patterns. Furthermore, industry experiments carried out in a real-world lead–zinc froth flotation plant provide industrial evidence and show that the newly designed controller is promising for practical flotation reagent control.
更多查看译文
关键词
Process modeling,Neural network,Incremental learning,Model mismatch,Froth flotation
AI 理解论文
溯源树
样例
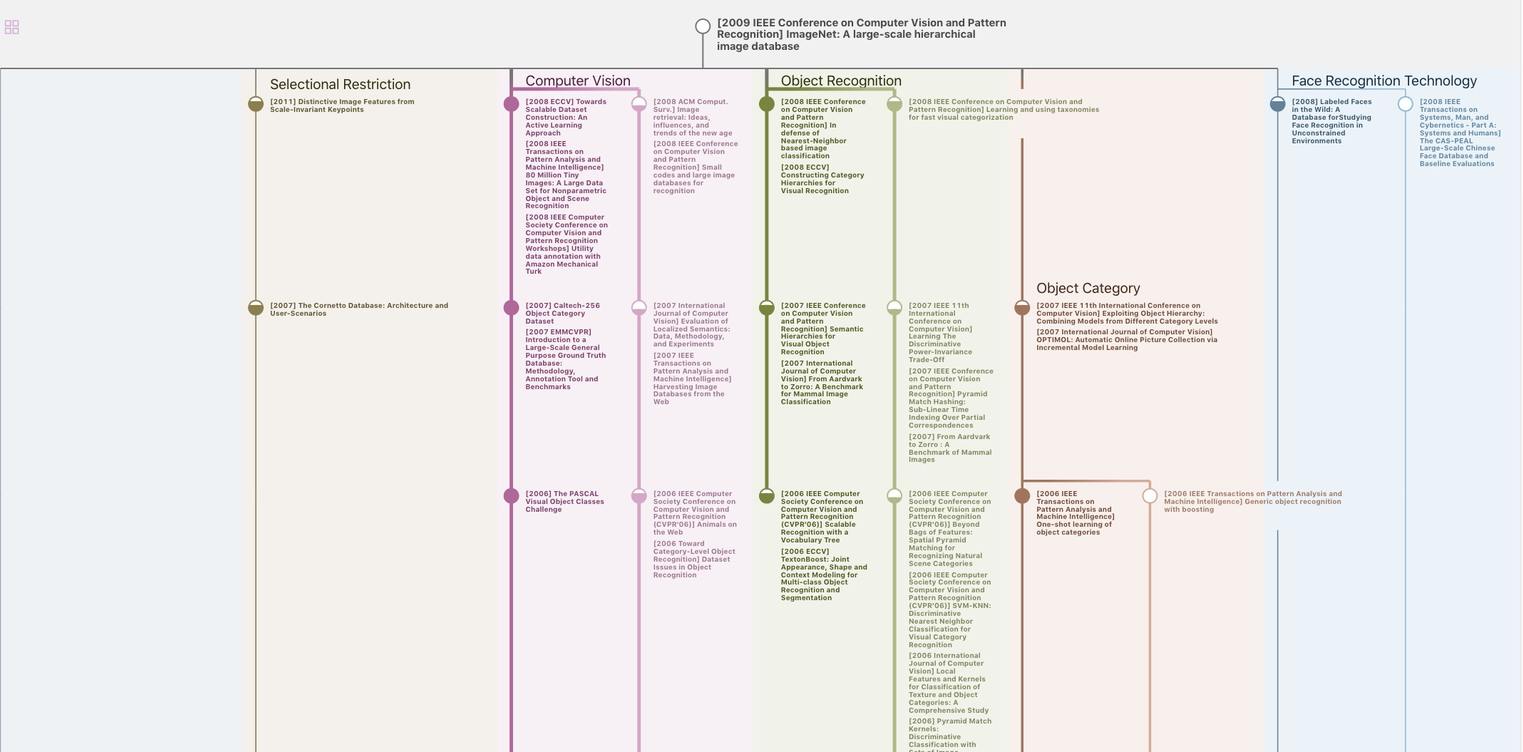
生成溯源树,研究论文发展脉络
Chat Paper
正在生成论文摘要