A new approach for microscopic diagnosis of malaria parasites in thick blood smears using pre-trained deep learning models
SN Applied Sciences(2020)
摘要
One of the deadly endemic diseases in sub-Saharan Africa is malaria. Its prevalence is promoted by lack of sufficient expertise to carry out accurate and timely diagnosis using the standard microscopy method. Where lab technicians are available, the results are usually subjective due to variations in expert judgement. To address this challenge, prompt interventions to improve disease control are needed. The emerging technologies of machine learning that can learn complex image patterns have accelerated research in medical image analysis. In this study, on a dataset of thick blood smear images, we evaluate and compare performance of three pre-trained deep learning architectures namely; faster regional convolutional neural network (faster R-CNN), single-shot multi-box detector (SSD) and RetinaNet through a Tensorflow object detection API. Data augmentation method was applied to optimise performance of the meta architectures. The possibility for mobile phone detector deployment was also investigated. The results revealed that faster R-CNN was the best trained model with a mean average precision of over 0.94 and SSD, was the best model for mobile deployment. We therefore deduce that faster R-CNN is best suited for obtaining high rates of accuracy in malaria detection while SDD is best suited for mobile deployment.
更多查看译文
关键词
Deep learning, Malaria detection, Thick blood smear, Mobile detector
AI 理解论文
溯源树
样例
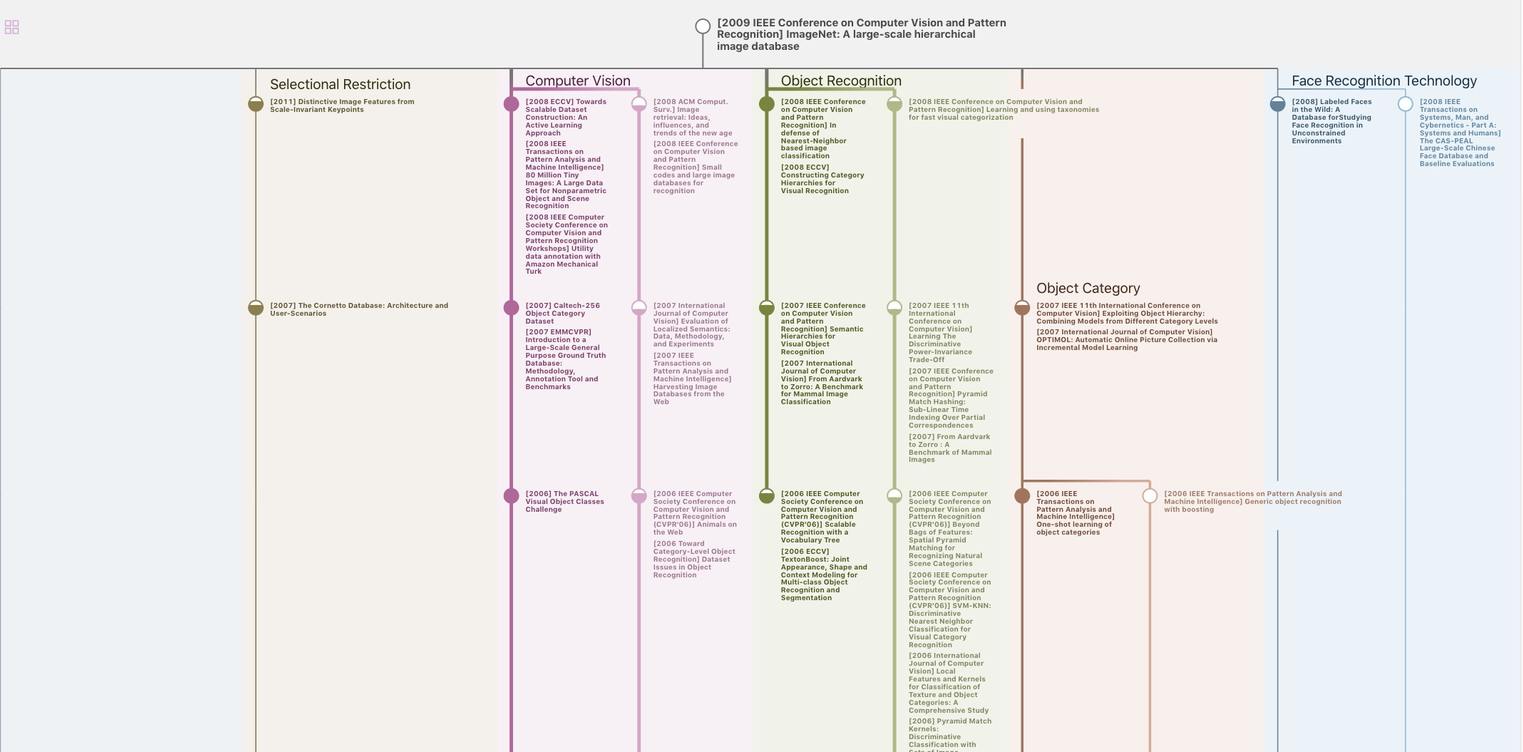
生成溯源树,研究论文发展脉络
Chat Paper
正在生成论文摘要