Sparse Logistic Regression With L 1/2 Penalty for Emotion Recognition in Electroencephalography Classification.
FRONTIERS IN NEUROINFORMATICS(2020)
摘要
Emotion recognition based on electroencephalography (EEG) signals is a current focus in brain-computer interface research. However, the classification of EEG is difficult owing to large amounts of data and high levels of noise. Therefore, it is important to determine how to effectively extract features that include important information. Regularization, one of the effective methods for EEG signal processing, can effectively extract important features from the signal and has potential applications in EEG emotion recognition. Currently, the most popular regularization technique is Lasso (L-1) and Ridge Regression (L-2). In recent years, researchers have proposed many other regularization terms. In theory,L-q-type regularization has a lowerqvalue, which means that it can be used to find solutions with better sparsity.L(1/2)regularization is ofL(q)type (0
更多
查看译文
关键词
EEG,emotion recognition,L(1)regularization,Ridge Regression,L-1,2regularization,sparse logistic regression
AI 理解论文
溯源树
样例
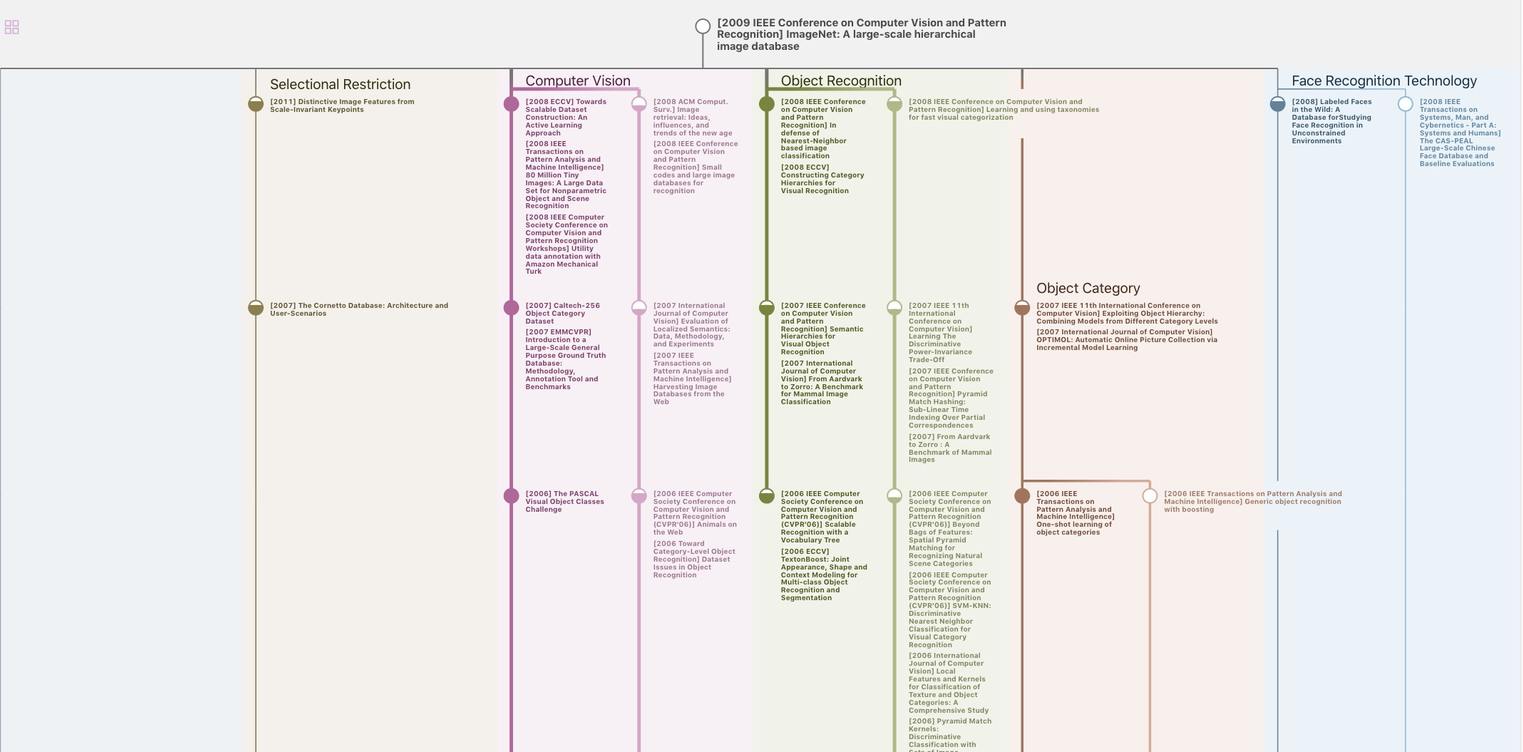
生成溯源树,研究论文发展脉络
Chat Paper
正在生成论文摘要