Variable selection for Gaussian process regression through a sparse projection
IISE TRANSACTIONS(2022)
摘要
This article presents a new variable selection approach integrated with Gaussian process regression. We consider a sparse projection of input variables and a general stationary covariance model that depends on the Euclidean distance between the projected features. The sparse projection matrix is considered as an unknown parameter. We propose a forward stagewise approach with embedded gradient descent steps to co-optimize the parameter with other covariance parameters based on the maximization of a non-convex marginal likelihood function with a concave sparsity penalty, and some convergence properties of the algorithm are provided. The proposed model covers a broader class of stationary covariance functions than the existing automatic relevance determination approaches, and the solution approach is more computationally feasible than the existing Markov chain Monte Carlo sampling procedures for the automatic relevance parameter estimation with a sparsity prior. The approach is evaluated for a large number of simulated scenarios. The choice of tuning parameters and the accuracy of the parameter estimation are evaluated with the simulation study. In comparison to some chosen benchmark approaches, the proposed approach has demonstrated improved accuracy in the variable selection. It is applied to an important problem of identifying environmental factors that affect atmospheric corrosion of metal alloys.
更多查看译文
关键词
Gaussian process regression, variable selection, sparse projection, forward stagewise regression, atmospheric corrosion
AI 理解论文
溯源树
样例
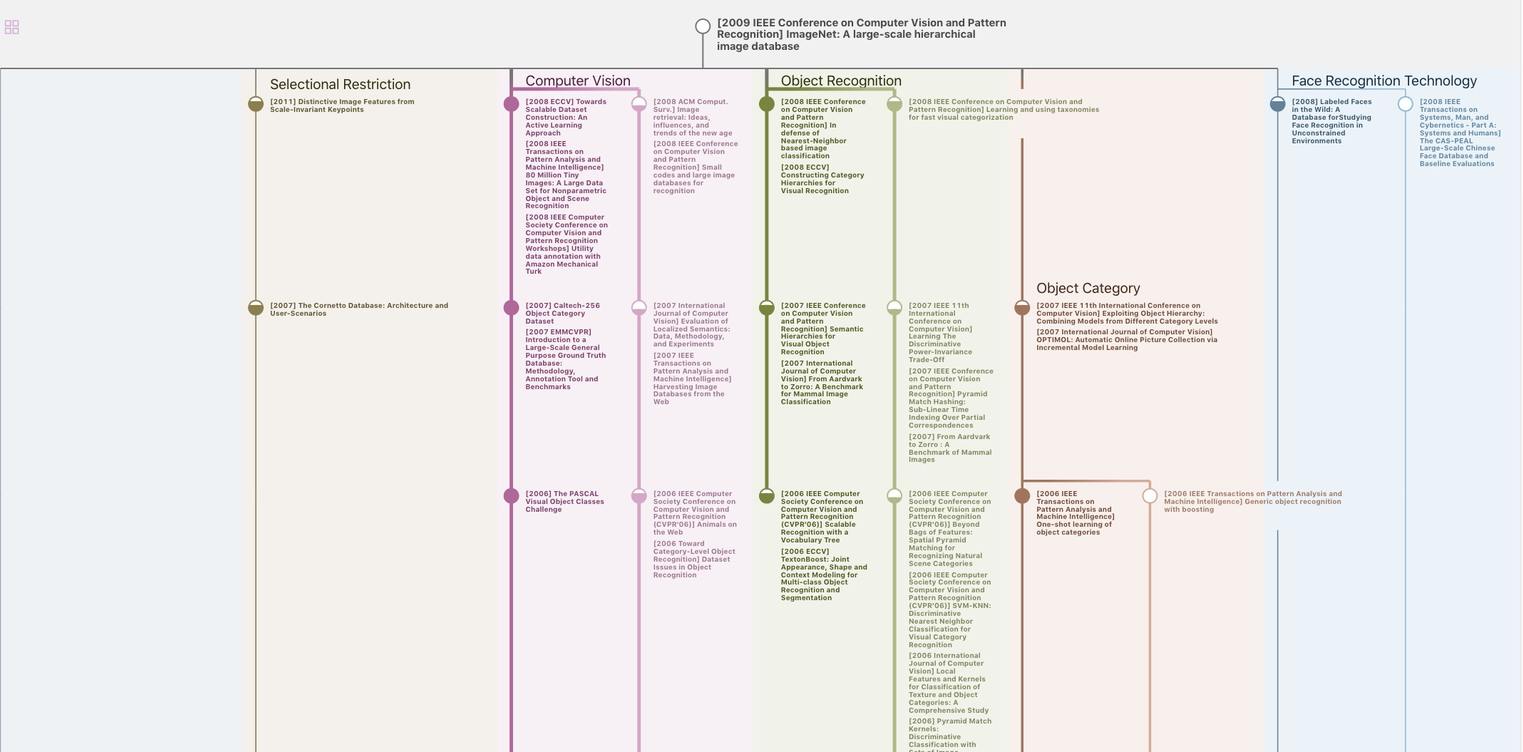
生成溯源树,研究论文发展脉络
Chat Paper
正在生成论文摘要