Evaluating Nonlinear Decision Trees for Binary Classification Tasks with Other Existing Methods
SSCI(2020)
摘要
Classification of datasets into two or more distinct classes is an important machine learning task. Many methods are able to classify binary classification tasks with a very high accuracy on test data, but cannot provide any easily interpretable explanation for users to have a deeper understanding of reasons for the split of data into two classes. In this paper, we highlight and evaluate a recently proposed nonlinear decision tree approach with a number of commonly used classification methods on a number of datasets involving a few to a large number of features. The study reveals key issues such as effect of classification on the method's parameter values, complexity of the classifier versus achieved accuracy, and interpretability of resulting classifiers.
更多查看译文
关键词
Interpretable AI, Classification, Genetic programming, Nonlinear decision trees, Generalized additive method
AI 理解论文
溯源树
样例
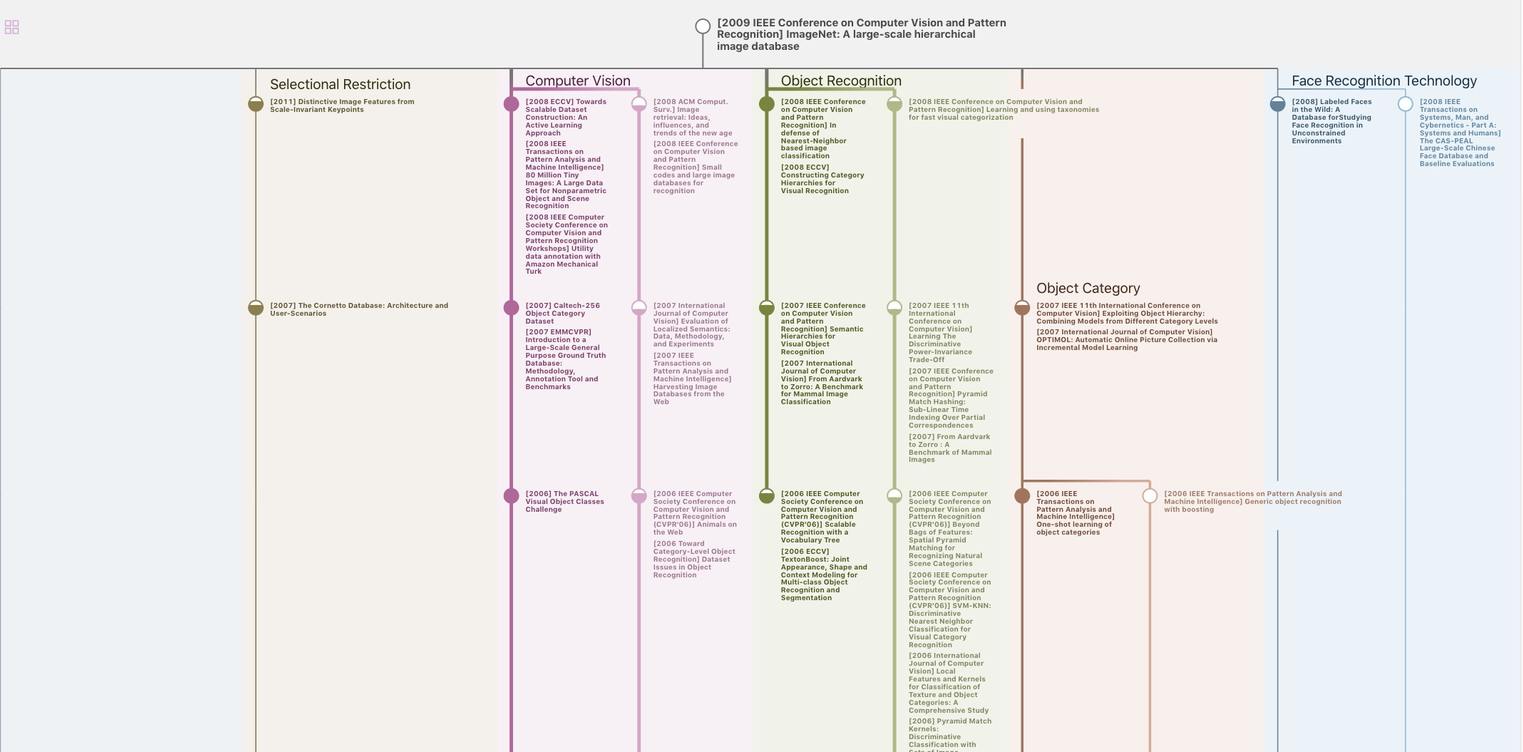
生成溯源树,研究论文发展脉络
Chat Paper
正在生成论文摘要