Data Driven Methods in Fluid Dynamics: Sparse Classification from Experimental Data
Whither Turbulence and Big Data in the 21st Century?(2017)
摘要
This work explores the use of data-driven methods, including machine learning and sparse sampling, for systems in fluid dynamics. In particular, camera images of a transitional separation bubble are used with dimensionality reduction and supervised classification techniques to discriminate between an actuated and an unactuated flow. After classification is demonstrated on full-resolution image data, similar classification performance is obtained using heavily subsampled pixels from the images. Finally, a sparse sensor optimization based on compressed sensing is used to determine optimal pixel locations for accurate classification. With 5–10 specially selected sensors, the median cross-validated classification accuracy is ≥ 97 %, as opposed to a random set of 5–10 pixels, which results in classification accuracy of 70–80 %. The methods developed here apply broadly to high-dimensional data from …
更多查看译文
AI 理解论文
溯源树
样例
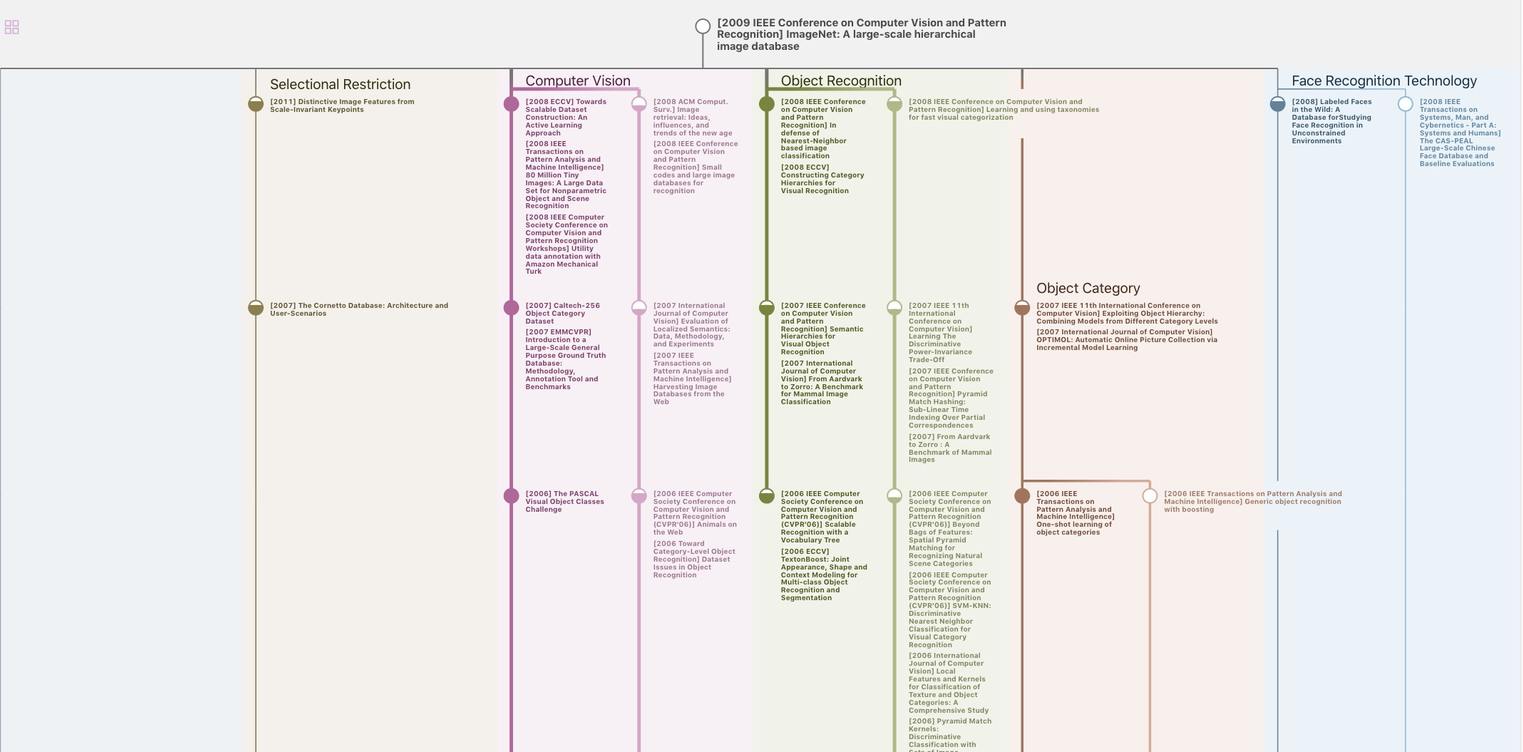
生成溯源树,研究论文发展脉络
Chat Paper
正在生成论文摘要