Learning Implicit Representations of 3D Object Orientations from RGB
ICRA Workshop: Representing a Complex World(2018)
摘要
This work presents a fast and robust algorithm for object orientation estimation that is solely trained on synthetic views rendered from a 3D model. We introduce a dense encoderdecoder architecture that learns implicit representations of 3D object orientations. Since our training is self-supervised, we avoid the necessity of real, pose-annotated training data. Furthermore, it prevents issues related to ambiguous object views. To encode latent representations that are robust against occlusions, clutter and the differences between synthetic and real data, a new domain randomization strategy is proposed. We motivate our approach by experiments on abstract 2D shapes and evaluate it on the challenging T-LESS dataset. In addition to the results in this paper, we provide a live presentation of the system during the workshop, on a Nvidia Jetson TX2 board.
更多查看译文
AI 理解论文
溯源树
样例
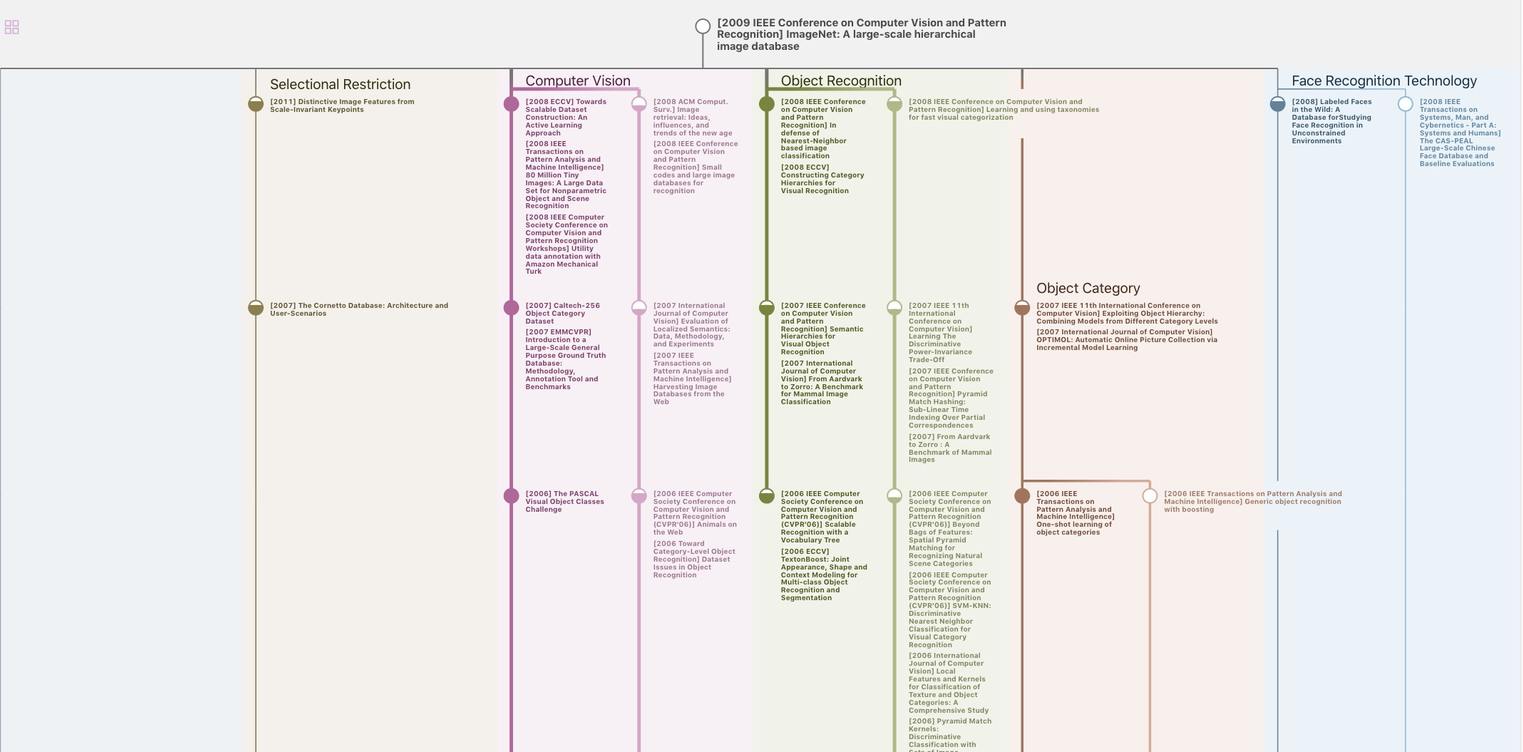
生成溯源树,研究论文发展脉络
Chat Paper
正在生成论文摘要