What Makes Fake Images Detectable? Understanding Properties that Generalize
European Conference on Computer Vision(2020)
摘要
The quality of image generation and manipulation is reaching impressive levels, making it increasingly difficult for a human to distinguish between what is real and what is fake. However, deep networks can still pick up on the subtle artifacts in these doctored images. We seek to understand what properties of fake images make them detectable and identify what generalizes across different model architectures, datasets, and variations in training. We use a patch-based classifier with limited receptive fields to visualize which regions of fake images are more easily detectable. We further show a technique to exaggerate these detectable properties and demonstrate that, even when the image generator is adversarially finetuned against a fake image classifier, it is still imperfect and leaves detectable artifacts in certain image patches. Code is available at https://github.com/chail/patch-forensics.
更多查看译文
关键词
Image forensics,Generative models,Image manipulation,Visualization,Generalization
AI 理解论文
溯源树
样例
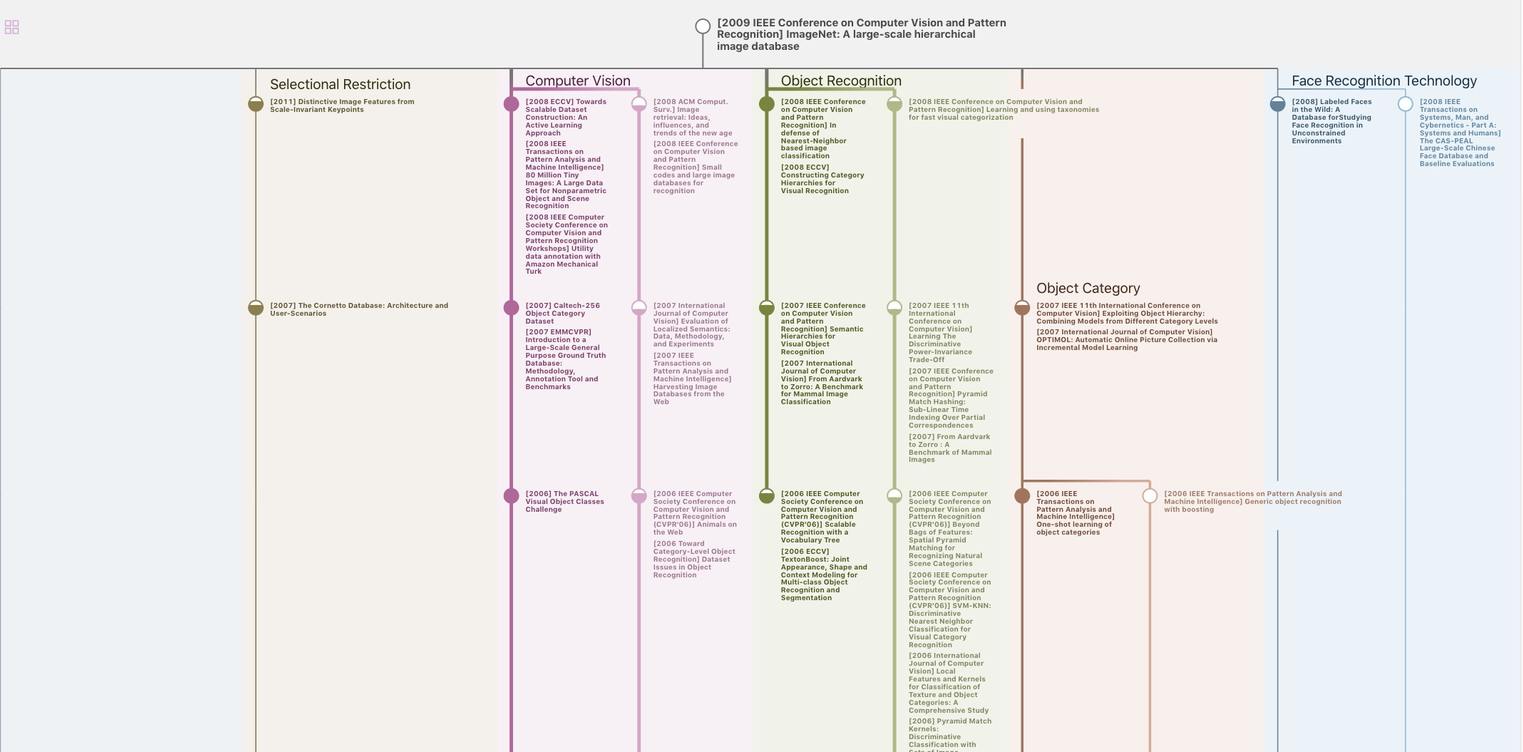
生成溯源树,研究论文发展脉络
Chat Paper
正在生成论文摘要