Classification Of Probable Online Social Networking Addiction: A Latent Profile Analysis From A Large-Scale Survey Among Chinese Adolescents
JOURNAL OF BEHAVIORAL ADDICTIONS(2020)
摘要
Background and aims: Problematic online social networking use is prevalent among adolescents, but consensus about the instruments and their optimal cut-off points is lacking. This study derived an optimal cut-off point for the validated Online Social Networking Addiction (OSNA) scale to identify probable OSNA cases among Chinese adolescents. Methods: A survey recruited 4,951 adolescent online social networking users. Latent profile analysis (LPA) and receiver operating characteristic curve (ROC) analyses were applied to the validated 8-item OSNA scale to determine its optimal cut-off point. Results: The 3-class model was selected by multiple criteria, and validated in a randomly split-half subsample. Accordingly, participants were categorized into the low risk (36.4%), average risk (50.4%), and high risk (13.2%) groups. The highest risk group was regarded as "cases" and the rest as "non-cases", serving as the reference standard in ROC analysis, which identified an optimal cut-off point of 23 (sensitivity: 97.2%, specificity: 95.2%). The cut-off point was used to classify participants into positive (probable case: 17:0%) and negative groups according to their OSNA scores. The positive group (probable cases) reported significantly longer duration and higher intensity of online social networking use, and higher prevalence of Internet addiction than the negative group. Conclusions: The classification strategy and results are potentially useful for future research that measure problematic online social networking use and its impact on health among adolescents. The approach can facilitate research that requires cut-off points of screening tools but gold standards are unavailable.
更多查看译文
关键词
online social networking addiction, classification, latent profile analysis, adolescents
AI 理解论文
溯源树
样例
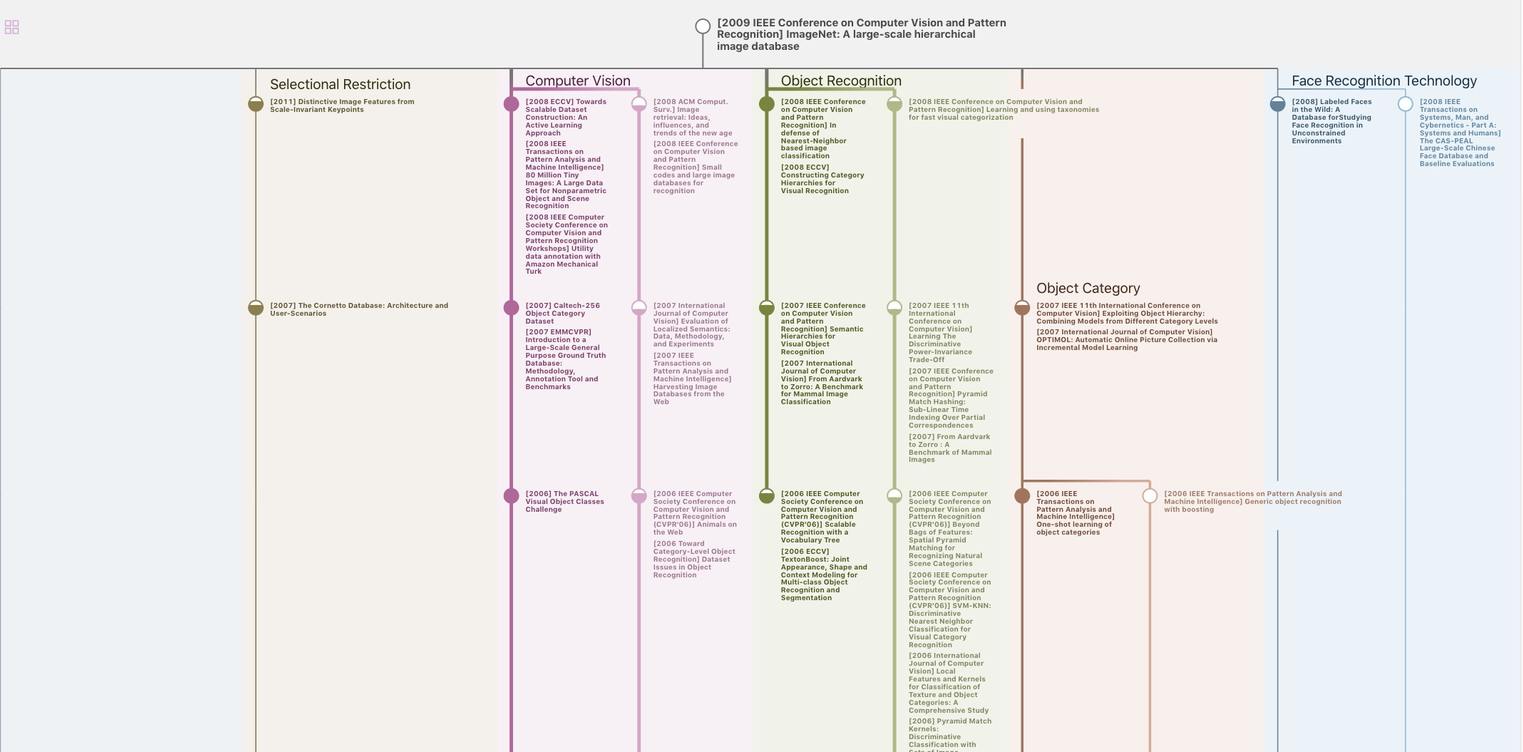
生成溯源树,研究论文发展脉络
Chat Paper
正在生成论文摘要