Large-Scale Evidence Generation And Evaluation Across A Network Of Databases (Legend): Assessing Validity Using Hypertension As A Case Study
JOURNAL OF THE AMERICAN MEDICAL INFORMATICS ASSOCIATION(2020)
摘要
Objectives: To demonstrate the application of the Large-scale Evidence Generation and Evaluation across a Network of Databases (LEGEND) principles described in our companion article to hypertension treatments and assess internal and external validity of the generated evidence.Materials and Methods: LEGEND defines a process for high-quality observational research based on 10 guiding principles. We demonstrate how this process, here implemented through large-scale propensity score modeling, negative and positive control questions, empirical calibration, and full transparency, can be applied to compare antihypertensive drug therapies. We assess internal validity through covariate balance, confidence-interval coverage, between-database heterogeneity, and transitivity of results. We assess external validity through comparison to direct meta-analyses of randomized controlled trials (RCTs).Results: From 21.6 million unique antihypertensive new users, we generate 6 076 775 effect size estimates for 699 872 research questions on 12 946 treatment comparisons. Through propensity score matching, we achieve balance on all baseline patient characteristics for 75% of estimates, observe 95.7% coverage in our effect-estimate 95% confidence intervals, find high between-database consistency, and achieve transitivity in 84.8% of triplet hypotheses. Compared with meta-analyses of RCTs, our results are consistent with 28 of 30 comparisons while providing narrower confidence intervals.Conclusion: We find that these LEGEND results show high internal validity and are congruent with meta-analyses of RCTs. For these reasons we believe that evidence generated by LEGEND is of high quality and can inform medical decision-making where evidence is currently lacking. Subsequent publications will explore the clinical interpretations of this evidence.
更多查看译文
关键词
hypertension, treatment effects, observational studies, open science, empirical calibration
AI 理解论文
溯源树
样例
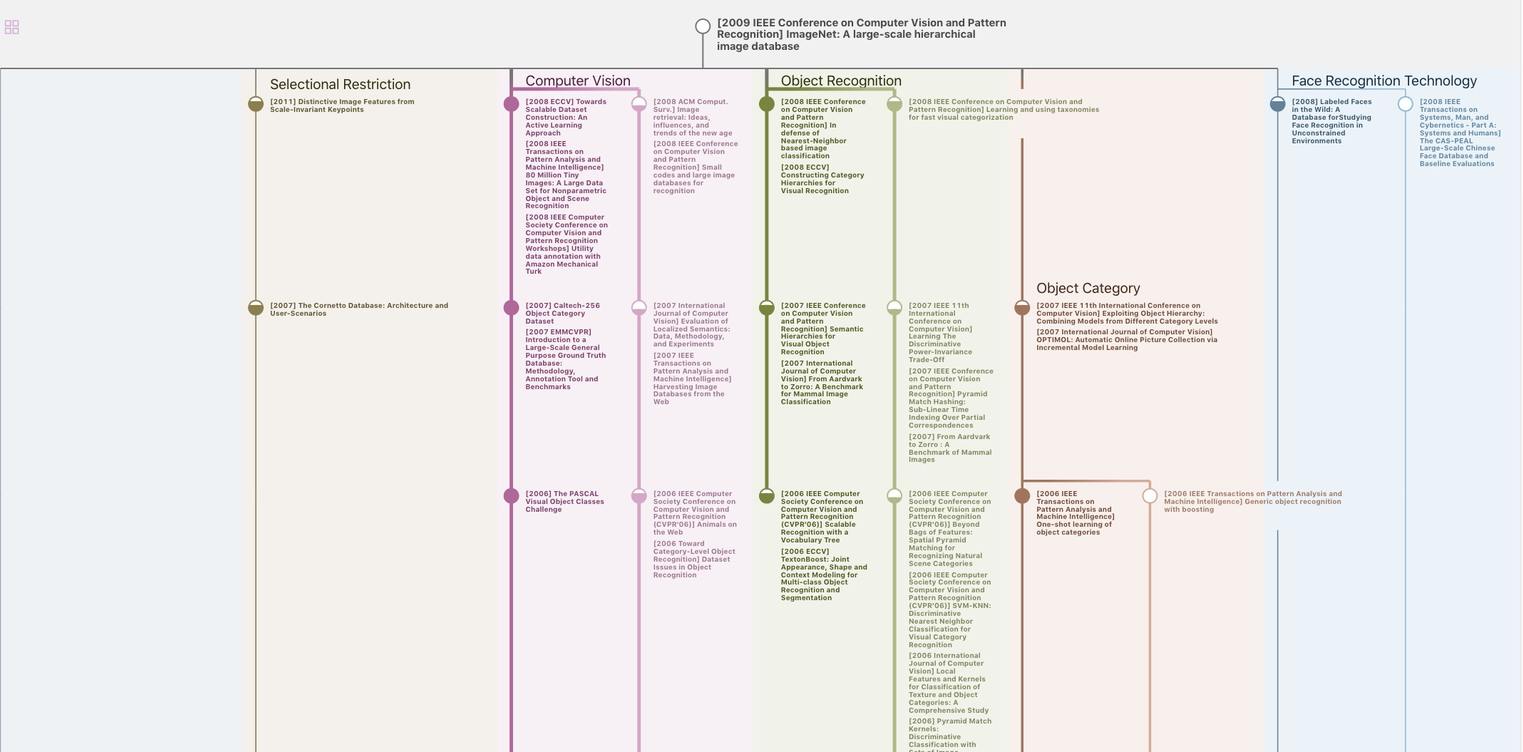
生成溯源树,研究论文发展脉络
Chat Paper
正在生成论文摘要