Reinforcement Learning For Robust Trajectory Design Of Interplanetary Missions
JOURNAL OF GUIDANCE CONTROL AND DYNAMICS(2021)
Abstract
This paper investigates the use of reinforcement learning for the robust design of low-thrust interplanetary trajectories in presence of severe uncertainties and disturbances, alternately modeled as Gaussian additive process noise, observation noise, and random errors in the actuation of the thrust control, including the occurrence of a missed thrust event. The stochastic optimal control problem is recast as a time-discrete Markov decision process to comply with the standard formulation of reinforcement learning. An open-source implementation of the state-of-the-art algorithm Proximal Policy Optimization is adopted to carry out the training process of a deep neural network, used to map the spacecraft (observed) states to the optimal control policy. The resulting guidance and control network provides both a robust nominal trajectory and the associated closed-loop guidance law. Numerical results are presented for a typical Earth-Mars mission. To validate the proposed approach, the solution found in a (deterministic) unperturbed scenario is first compared with the optimal one provided by an indirect technique. The robustness and optimality of the obtained closed-loop guidance laws is then assessed by means of Monte Carlo campaigns performed in the considered uncertain scenarios.
MoreTranslated text
Key words
interplanetary missions,reinforcement,low-thrust
AI Read Science
Must-Reading Tree
Example
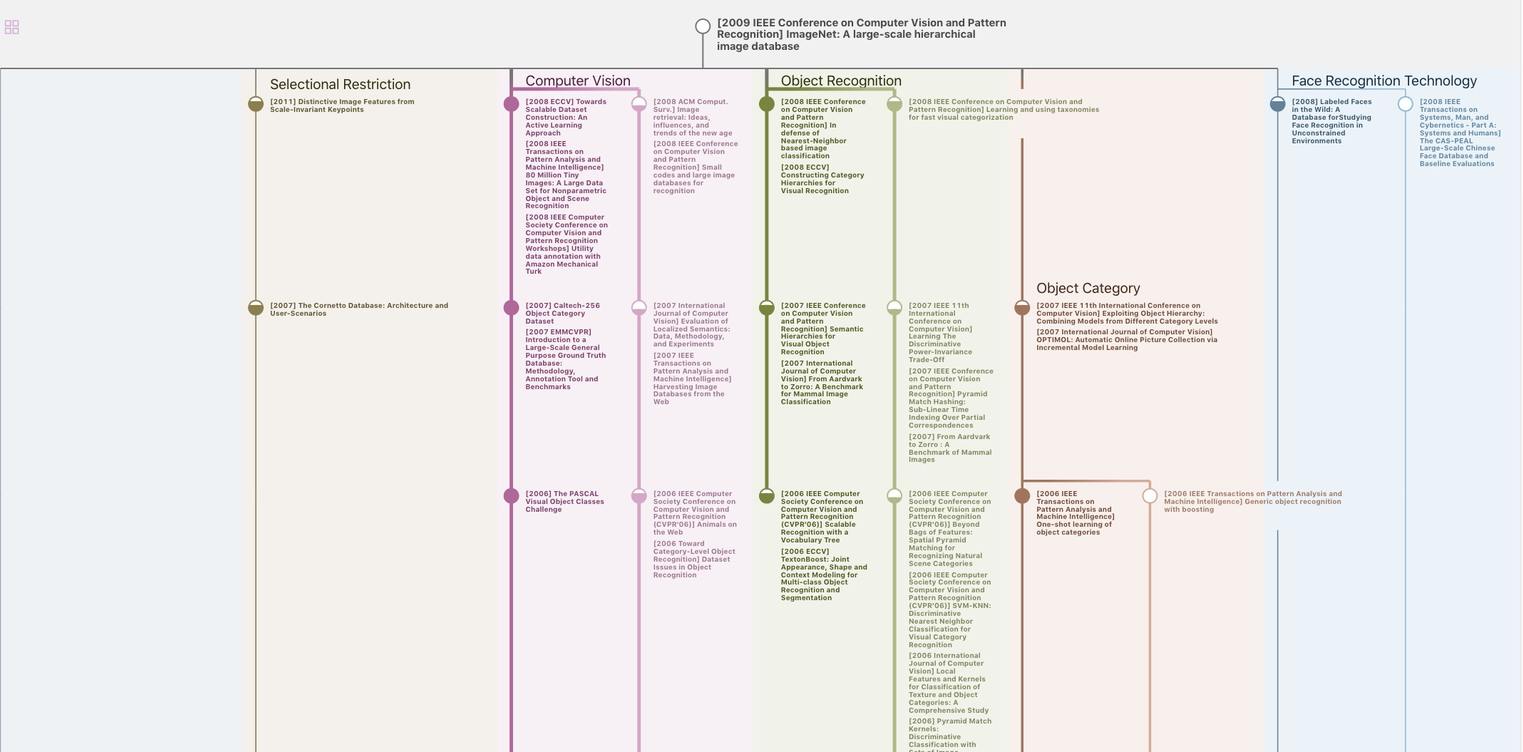
Generate MRT to find the research sequence of this paper
Chat Paper
Summary is being generated by the instructions you defined