Synergy in fertility forecasting: improving forecast accuracy through model averaging
Genus(2020)
摘要
Accuracy in fertility forecasting has proved challenging and warrants renewed attention. One way to improve accuracy is to combine the strengths of a set of existing models through model averaging. The model-averaged forecast is derived using empirical model weights that optimise forecast accuracy at each forecast horizon based on historical data. We apply model averaging to fertility forecasting for the first time, using data for 17 countries and six models. Four model-averaging methods are compared: frequentist, Bayesian, model confidence set, and equal weights. We compute individual-model and model-averaged point and interval forecasts at horizons of one to 20 years. We demonstrate gains in average accuracy of 4–23% for point forecasts and 3–24% for interval forecasts, with greater gains from the frequentist and equal weights approaches at longer horizons. Data for England and Wales are used to illustrate model averaging in forecasting age-specific fertility to 2036. The advantages and further potential of model averaging for fertility forecasting are discussed. As the accuracy of model-averaged forecasts depends on the accuracy of the individual models, there is ongoing need to develop better models of fertility for use in forecasting and model averaging. We conclude that model averaging holds considerable promise for the improvement of fertility forecasting in a systematic way using existing models and warrants further investigation.
更多查看译文
关键词
Fertility forecasting,Age-specific fertility,Forecast accuracy,Model averaging,Frequentist,Bayesian,Model confidence set,Computational methods,Functional time-series model,Univariate time-series model
AI 理解论文
溯源树
样例
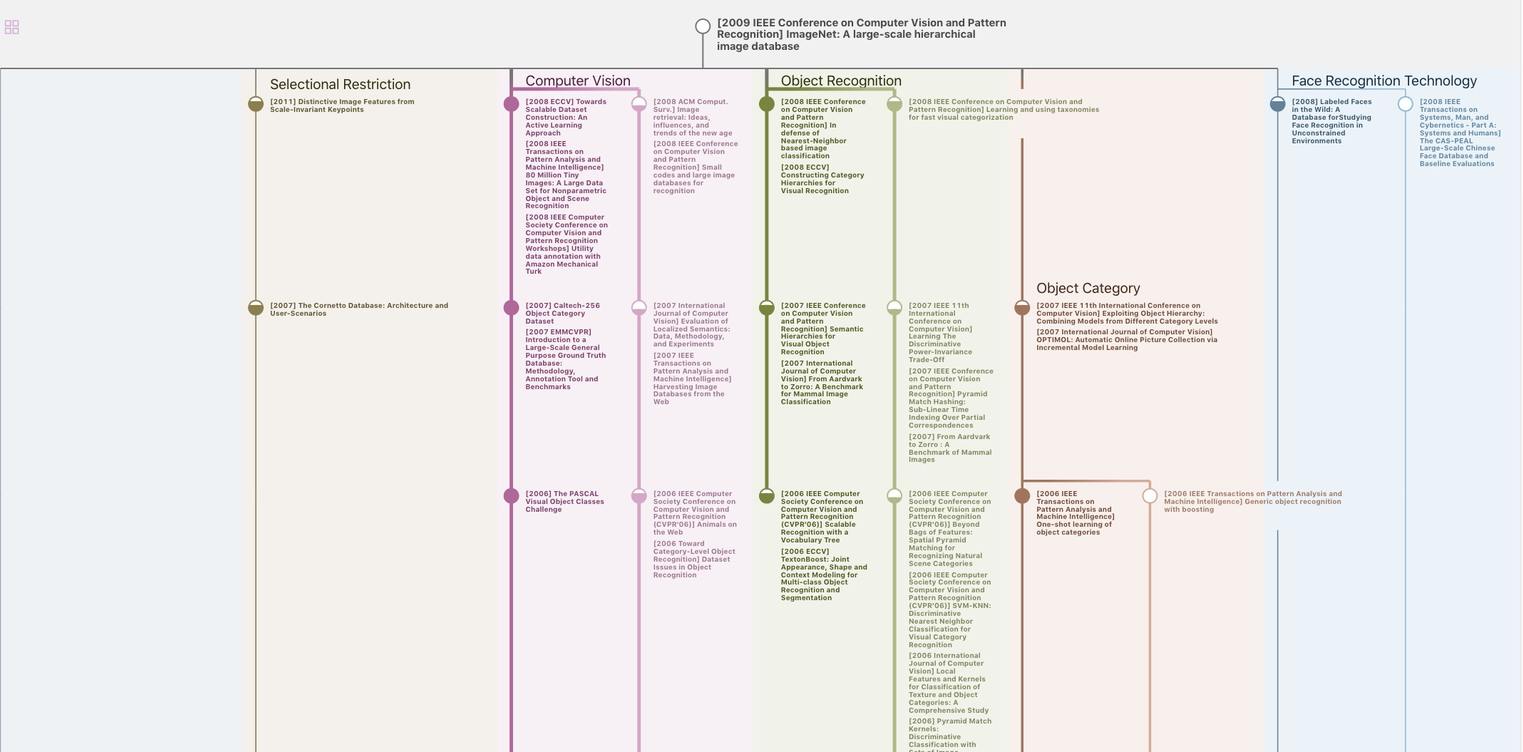
生成溯源树,研究论文发展脉络
Chat Paper
正在生成论文摘要