DIRECT-Net: a unified mutual-domain material decomposition network for quantitative dual-energy CT imaging
arxiv(2020)
摘要
By acquiring two sets of tomographic measurements at distinct X-ray spectra, the dual-energy CT (DECT) enables quantitative material-specific imaging. However, the conventionally decomposed material basis images may encounter severe image noise amplification and artifacts, resulting in degraded image quality and decreased quantitative accuracy. Iterative DECT image reconstruction algorithms incorporating either the sinogram or the CT image prior information have shown potential advantages in noise and artifact suppression, but with the expense of large computational resource, prolonged reconstruction time, and tedious manual selections of algorithm parameters. To partially overcome these limitations, we develop a domain-transformation enabled end-to-end deep convolutional neural network (DIRECT-Net) to perform high quality DECT material decomposition. Specifically, the proposed DIRECT-Net has immediate accesses to mutual-domain data, and utilizes stacked convolution neural network (CNN) layers for noise reduction and material decomposition. The training data are numerically simulated based on the underlying physics of DECT imaging.The XCAT digital phantom, iodine solutions phantom, and biological specimen are used to validate the performance of DIRECT-Net. The qualitative and quantitative results demonstrate that this newly developed DIRECT-Net is promising in suppressing noise, improving image accuracy, and reducing computation time for future DECT imaging.
更多查看译文
关键词
CNN, deep learning, dual-energy CT, end-to-end, material decomposition, quantitative imaging
AI 理解论文
溯源树
样例
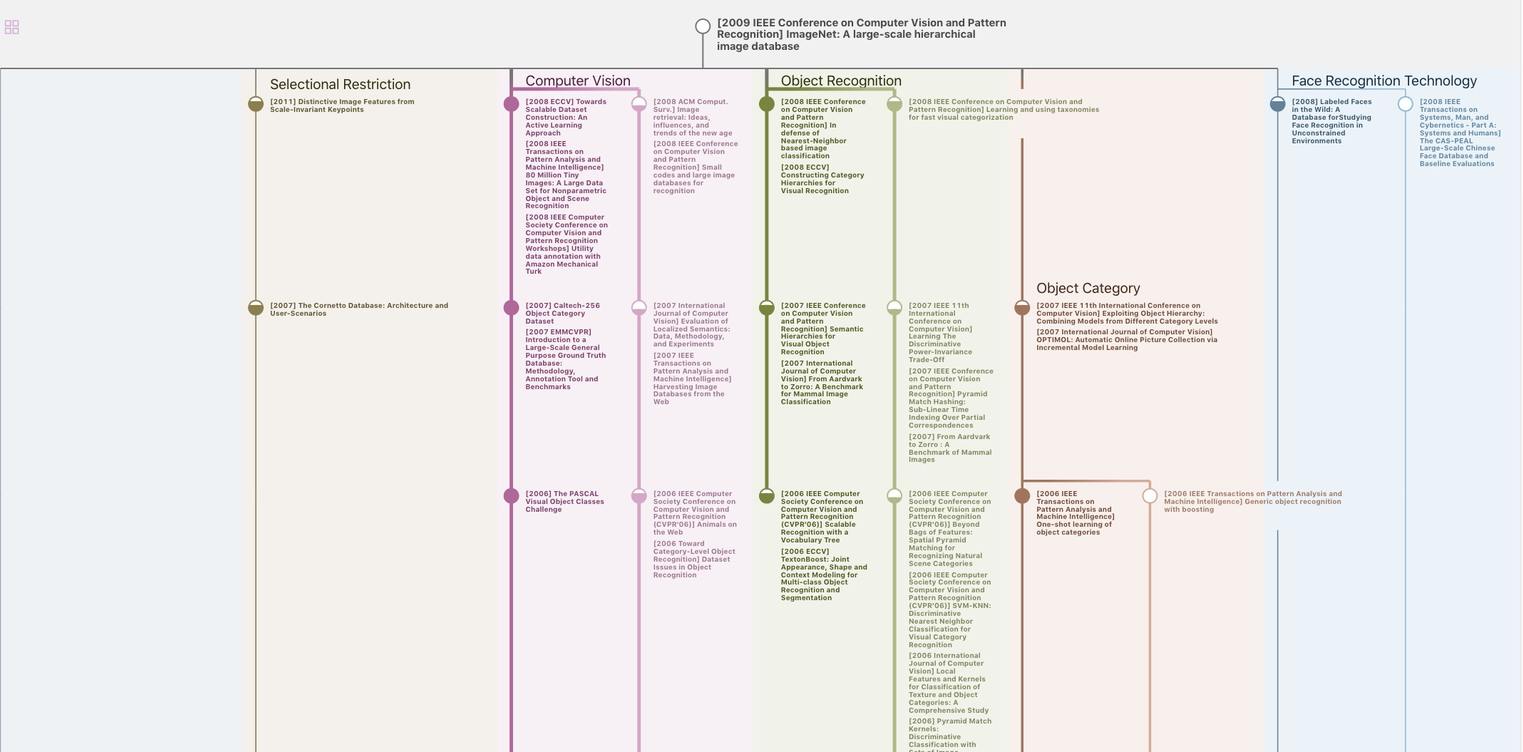
生成溯源树,研究论文发展脉络
Chat Paper
正在生成论文摘要