Deep kernel learning approach to engine emissions modeling
Data-Centric Engineering(2020)
Abstract
We apply deep kernel learning (DKL), which can be viewed as a combination of a Gaussian process (GP) and a deep neural network (DNN), to compression ignition engine emissions and compare its performance to a selection of other surrogate models on the same dataset. Surrogate models are a class of computationally cheaper alternatives to physics-based models. High-dimensional model representation (HDMR) is also briefly discussed and acts as a benchmark model for comparison. We apply the considered methods to a dataset, which was obtained from a compression ignition engine and includes as outputs soot and NOx emissions as functions of 14 engine operating condition variables. We combine a quasi-random global search with a conventional grid-optimization method in order to identify suitable values for several DKL hyperparameters, which include network architecture, kernel, and learning …
MoreTranslated text
Key words
Deep kernel learning, emissions, surrogate models, Gaussian processes, internal combustion engines
AI Read Science
Must-Reading Tree
Example
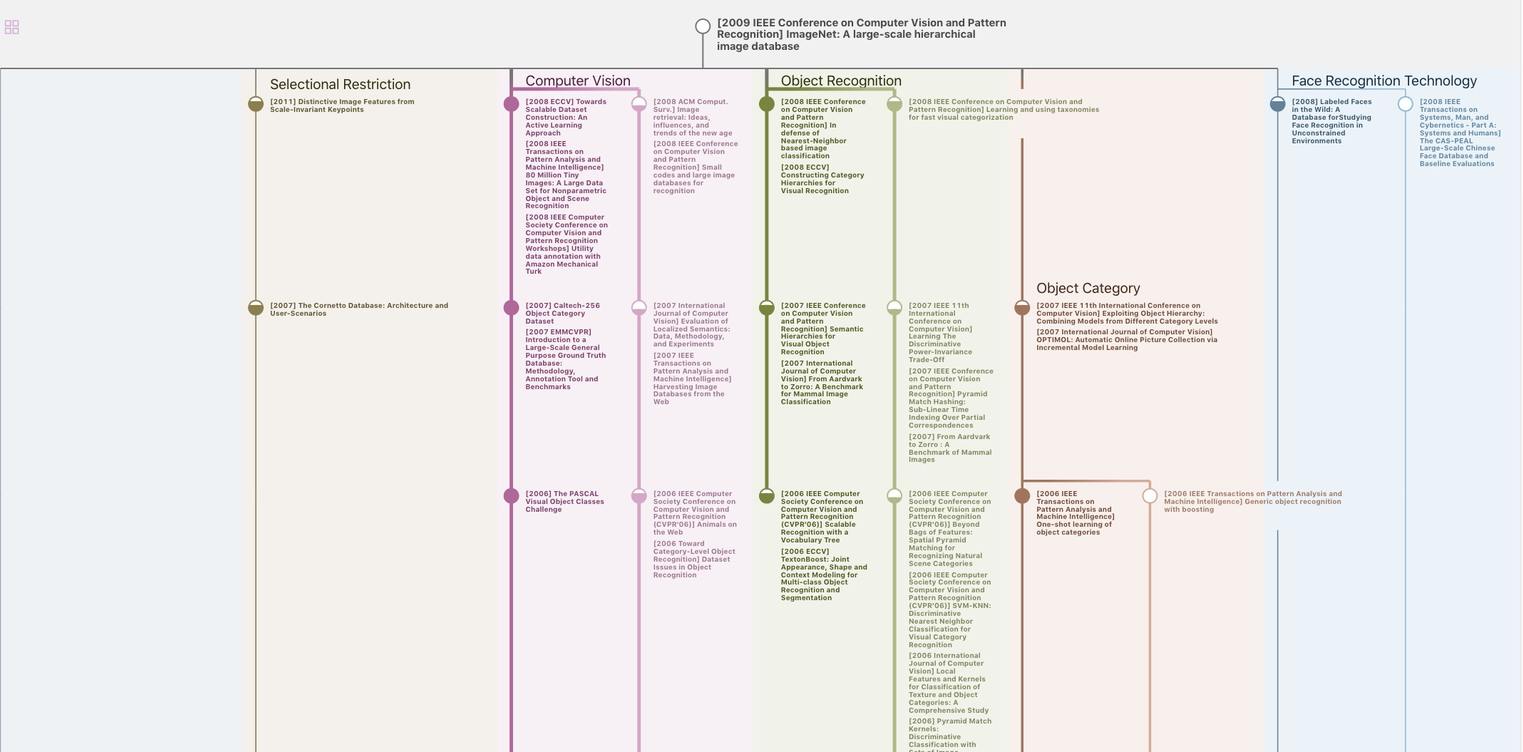
Generate MRT to find the research sequence of this paper
Chat Paper
Summary is being generated by the instructions you defined