Self-Supervised Learning for Monocular Depth Estimation from Aerial Imagery
ISPRS Annals of the Photogrammetry, Remote Sensing and Spatial Information Sciences(2020)
Abstract
Abstract. Supervised learning based methods for monocular depth estimation usually require large amounts of extensively annotated training data. In the case of aerial imagery, this ground truth is particularly difficult to acquire. Therefore, in this paper, we present a method for self-supervised learning for monocular depth estimation from aerial imagery that does not require annotated training data. For this, we only use an image sequence from a single moving camera and learn to simultaneously estimate depth and pose information. By sharing the weights between pose and depth estimation, we achieve a relatively small model, which favors real-time application. We evaluate our approach on three diverse datasets and compare the results to conventional methods that estimate depth maps based on multi-view geometry. We achieve an accuracy δ1:25 of up to 93.5 %. In addition, we have paid particular attention to the generalization of a trained model to unknown data and the self-improving capabilities of our approach. We conclude that, even though the results of monocular depth estimation are inferior to those achieved by conventional methods, they are well suited to provide a good initialization for methods that rely on image matching or to provide estimates in regions where image matching fails, e.g. occluded or texture-less regions.
MoreTranslated text
Key words
Monocular Depth Estimation,Depth Estimation,Unsupervised Learning,Autofocusing
AI Read Science
Must-Reading Tree
Example
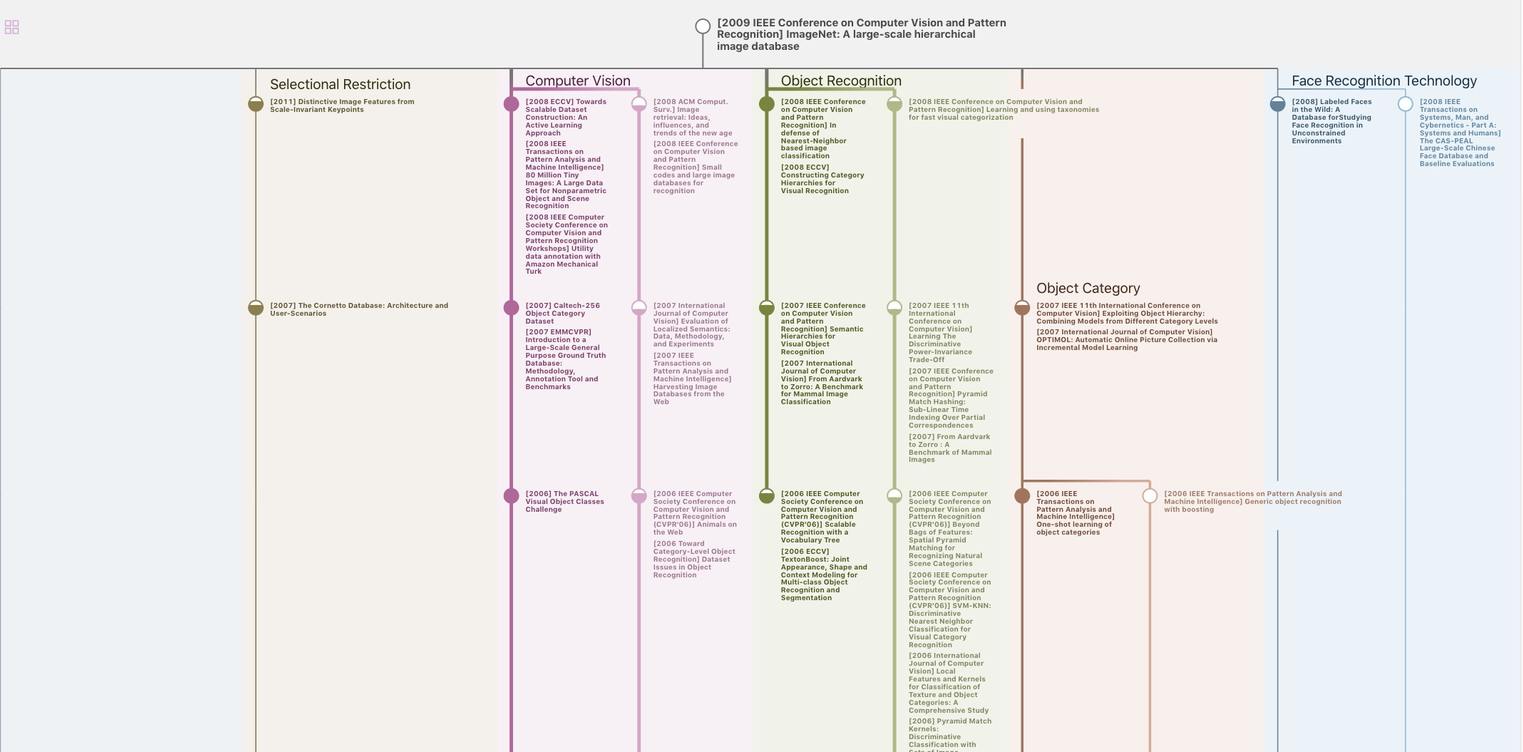
Generate MRT to find the research sequence of this paper
Chat Paper
Summary is being generated by the instructions you defined