Shifted Convolutions for Speeding up of Convolutional networks
user-5efd71244c775ed682ed8a03(2017)
摘要
The work introduces shifted convolutions: convolutions with a kernel size of 1× 1 applied to feature maps shifted relatively to each over. Shifted convolution layer is inspired by the dilated convolution layer and has the potential to speed up semantic segmentation significantly, if properly implemented on GPU. The idea of the new layer is to enable segmenting convolutional networks to attain large receptive fields at each pixel, without blowing up the number of operations or learnable parameters. Promising initial results have been obtained for the new layer for the semantic segmentation on Stanford Backgrounds dataset. The visualizations of the effective receptive fields suggest that the layer is successful at its tasks.
更多查看译文
AI 理解论文
溯源树
样例
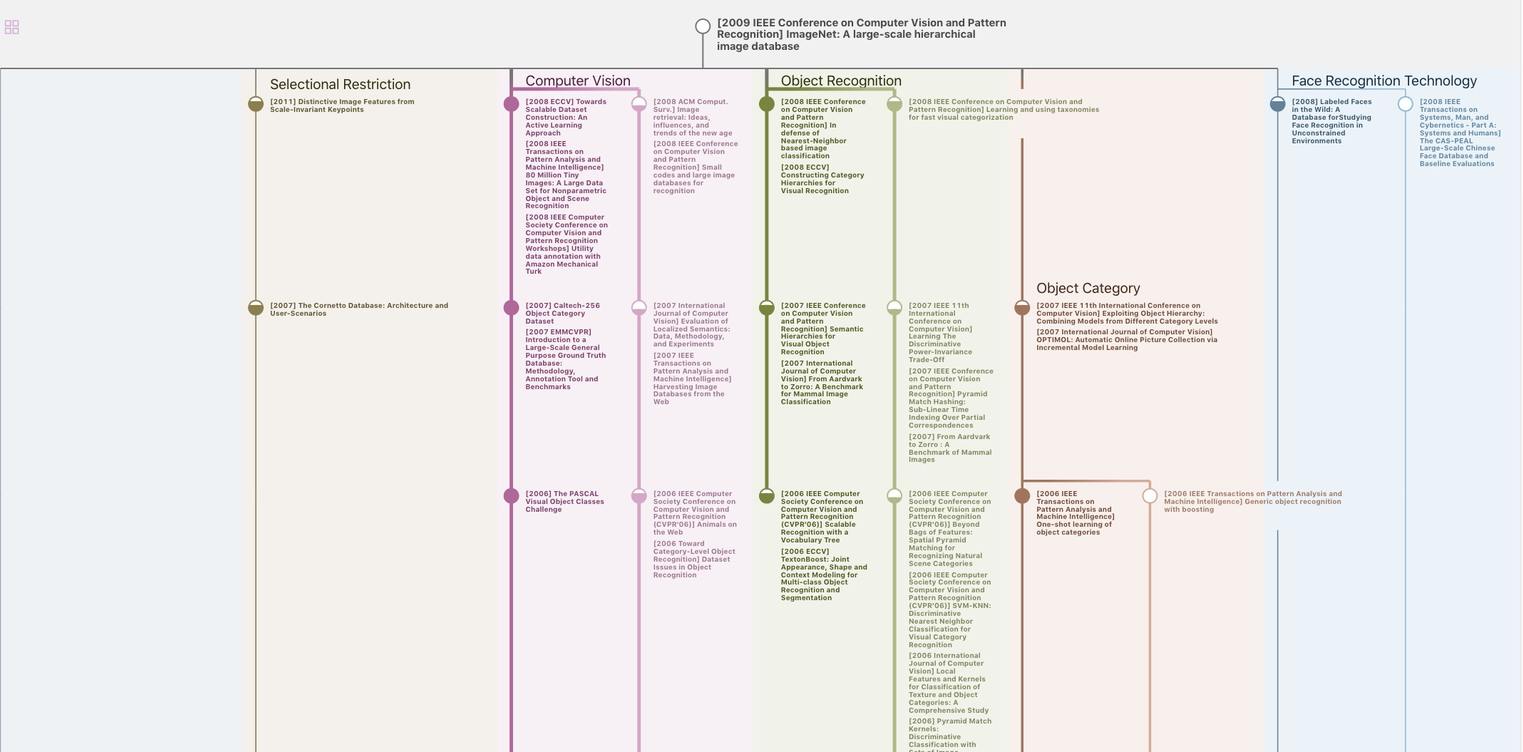
生成溯源树,研究论文发展脉络
Chat Paper
正在生成论文摘要