Learning-Based Gravity Estimation For Robot Manipulator Using Krr And Svr
2020 IEEE/ASME INTERNATIONAL CONFERENCE ON ADVANCED INTELLIGENT MECHATRONICS (AIM)(2020)
摘要
In this paper, a learning-based method for estimating the parameters of the gravity term of a manipulator with the kernel trick approach is presented. This method extracts the mapping equation from the analytical form of the dynamic equation. Based only on the configuration and sampling data of the robotic arm, Kernel ridge regression (KRR) and Support vector regression (SVR) algorithms are introduced to estimate the position parameters and provide a comparison between different learning regression techniques. The novelty of this work is the time-efficient estimation of robot gravity through randomly located joint sampling data using the kernel trick. The optimal solution to the optimal trade-off curve is proposed and discussed. Theoretical analysis shows that the joint angle and driving torque can be used to estimate the relationship between the center of gravity of the manipulator links and the mass of the connecting rod to obtain an accurate dynamic gravity model.
更多查看译文
关键词
gravity term,kernel trick approach,mapping equation,dynamic equation,robotic arm,KRR,SVR,position parameters,different learning regression techniques,time-efficient estimation,robot gravity,randomly located joint sampling data,joint angle,driving torque,manipulator links,accurate dynamic gravity model,gravity estimation,robot manipulator,learning-based method
AI 理解论文
溯源树
样例
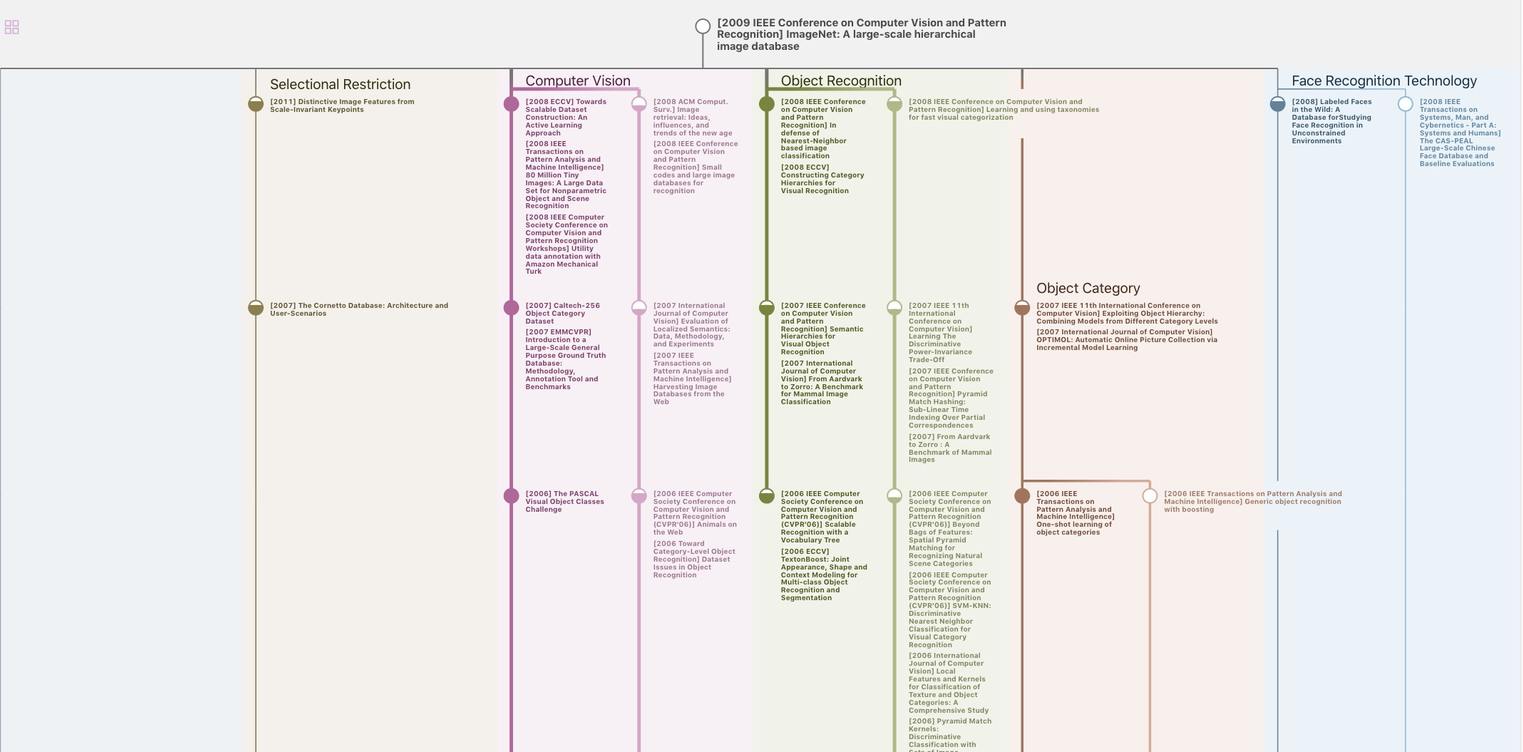
生成溯源树,研究论文发展脉络
Chat Paper
正在生成论文摘要