A Geometry-Aware Hidden Markov Model for Indoor Positioning
2020 IEEE/ASME International Conference on Advanced Intelligent Mechatronics (AIM)(2020)
摘要
Position estimation in localization and tracking is often approached with Bayesian filtering. In combination with Gaussian assumptions about state distributions this approach results in efficient algorithms such as the Kalman filter. Indoors, however, the location state space is naturally constrained by walls and other obstacles that cannot be taken into account with Kalman or other Gaussian filters. More general Hidden Markov Models (HMMs) on the other hand do not make restrictive assumptions and thus allow for extensive explicit modelling. In particular for indoor positioning, we show how to inject geometric apriori knowledge, i.e. a given floor plan, into the parameters of a discrete-state HMM. In contrast to classical position estimation, we use an adapted Viterbi-algorithm for decoding the maximum aposteriori (MAP) trajectory estimate based on the geometry-aware HMM. Results and advantages of the presented method are discussed for the localization of a mobile sensor node in a simulated measurement setup.
更多查看译文
关键词
geometry-aware Hidden Markov model,indoor positioning,Bayesian filtering,Gaussian assumptions,state distributions this approach,Kalman filter,location state space,Gaussian filters,general Hidden Markov Models,restrictive assumptions,extensive explicit modelling,geometric apriori knowledge,given floor plan,discrete-state HMM,classical position estimation,adapted Viterbi-algorithm,maximum aposteriori trajectory estimate,geometry-aware HMM
AI 理解论文
溯源树
样例
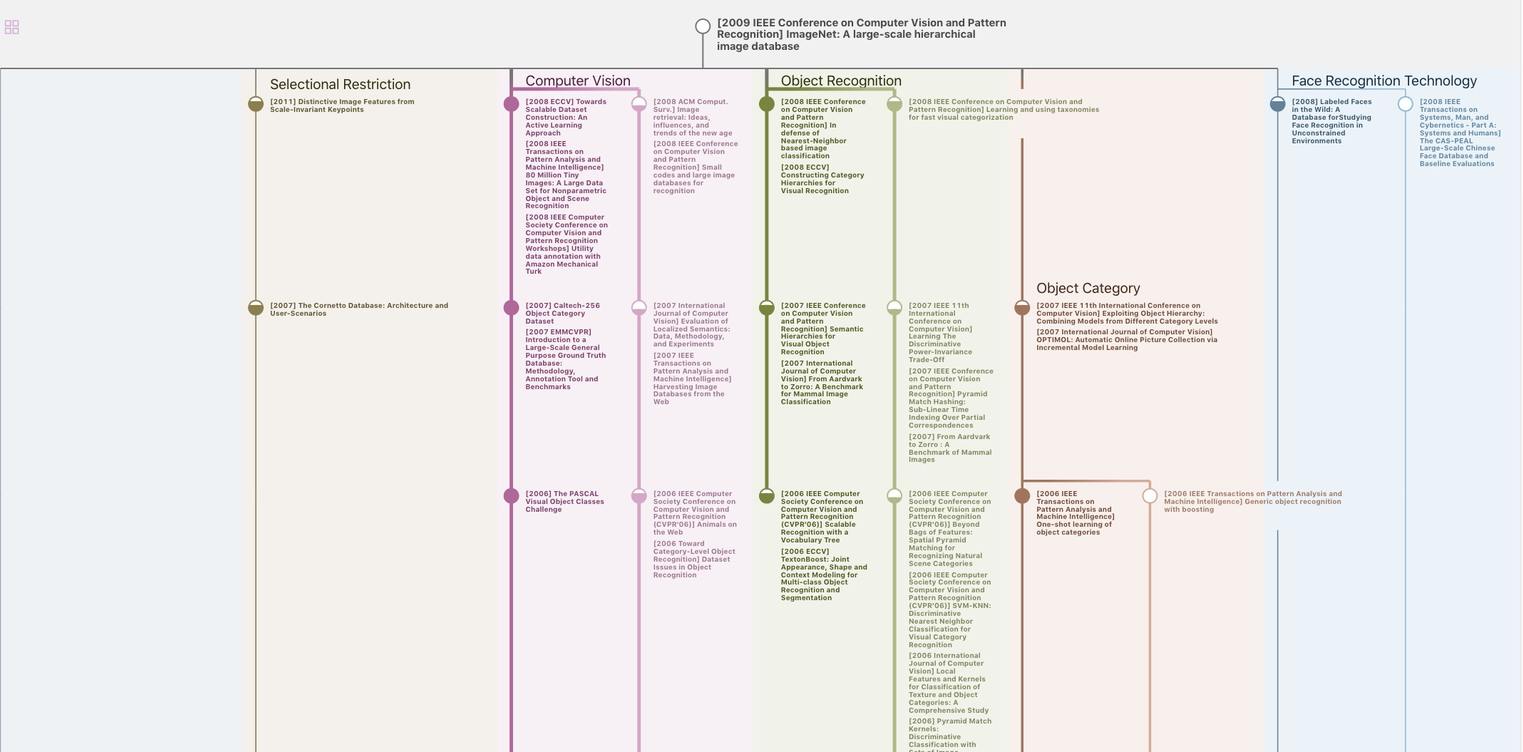
生成溯源树,研究论文发展脉络
Chat Paper
正在生成论文摘要