Local differential privacy for human-centered computing
EURASIP Journal on Wireless Communications and Networking(2020)
摘要
Human-centered computing in cloud, edge, and fog is one of the most concerning issues. Edge and fog nodes generate huge amounts of data continuously, and the analysis of these data provides valuable information. But they also increase privacy risks. The personal sensitive data may be disclosed by untrusted third-party service providers, and the current solutions to privacy protection are inefficient, costly. It is difficult to obtain available statistics. To solve these problems, we propose a local differential privacy sensitive data collection protocol in human-centered computing. Firstly, to maintain high data utility, the selection of the optimal number of hash functions and the mapping length is based on the size of the collected data. Secondly, we hash the sensitive data, add the appropriate Laplace noise to the client side, and send the reports to the server side. Thirdly, we construct the count sketch matrix to obtain privacy statistics on the server side. Finally, the utility of the proposed protocol is verified by synthetic datasets and a real dataset. The experimental results demonstrate that the protocol can achieve a balance between data utility and privacy protection.
更多查看译文
关键词
Human-centered computing, Local differential privacy, Laplace noise, Count sketch
AI 理解论文
溯源树
样例
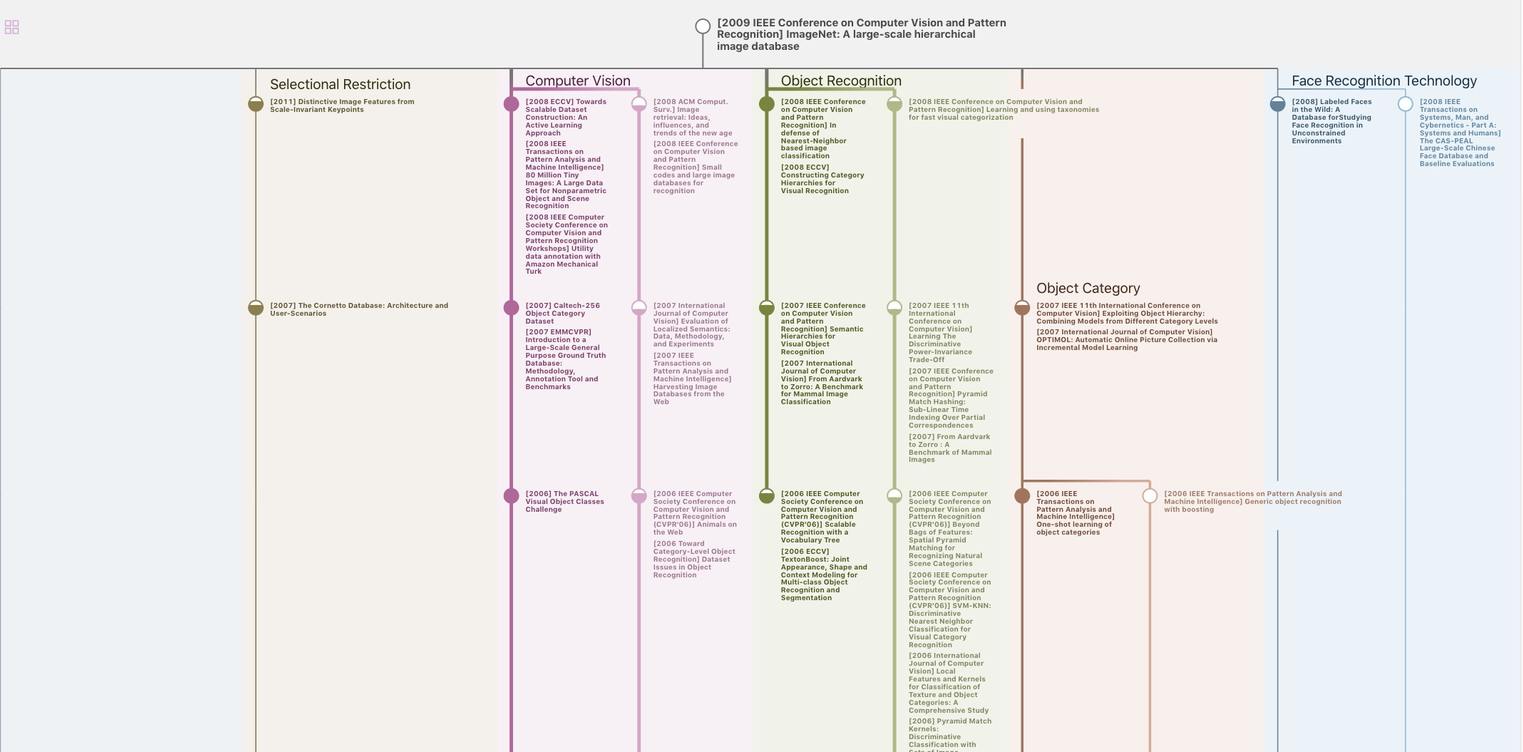
生成溯源树,研究论文发展脉络
Chat Paper
正在生成论文摘要