Hyperspectral image restoration via CNN denoiser prior regularized low-rank tensor recovery
COMPUTER VISION AND IMAGE UNDERSTANDING(2020)
摘要
Hyperspectral images (HSIs) are widely used in various tasks such as mineral detection and food safety. However, during the imaging process, they are often contaminated by various noises. In this paper for HSIs restoration tasks, we firstly investigate the advantages of traditional physical restoration models and the denoising convolutional neural networks (CNN). For the physical prior of HSIs, a Tucker decomposition based low-rank tensor approximation can fully explore the global correlations in both the spatial and spectral domains. And for the implicit prior, a CNN based method can represent the prior which cannot be designed by mathematical theory tools. Then, we combine the advantages of the two methods to introduce the HSI restoration CNN with the low-rank tensor approximation based regularization in the flexible and extensible plug-and-play framework. The proposed model can be quickly solved using the alternating direction method of multipliers method. Experiments with simulated data and real data show that, compared with competitive methods, the proposed method achieves better HSI restoration results in various quantitative evaluation indicators.
更多查看译文
关键词
Hyperspectral image (HSI),Low-rank tensor decomposition,Plug-and-play,Deep prior,Restoration
AI 理解论文
溯源树
样例
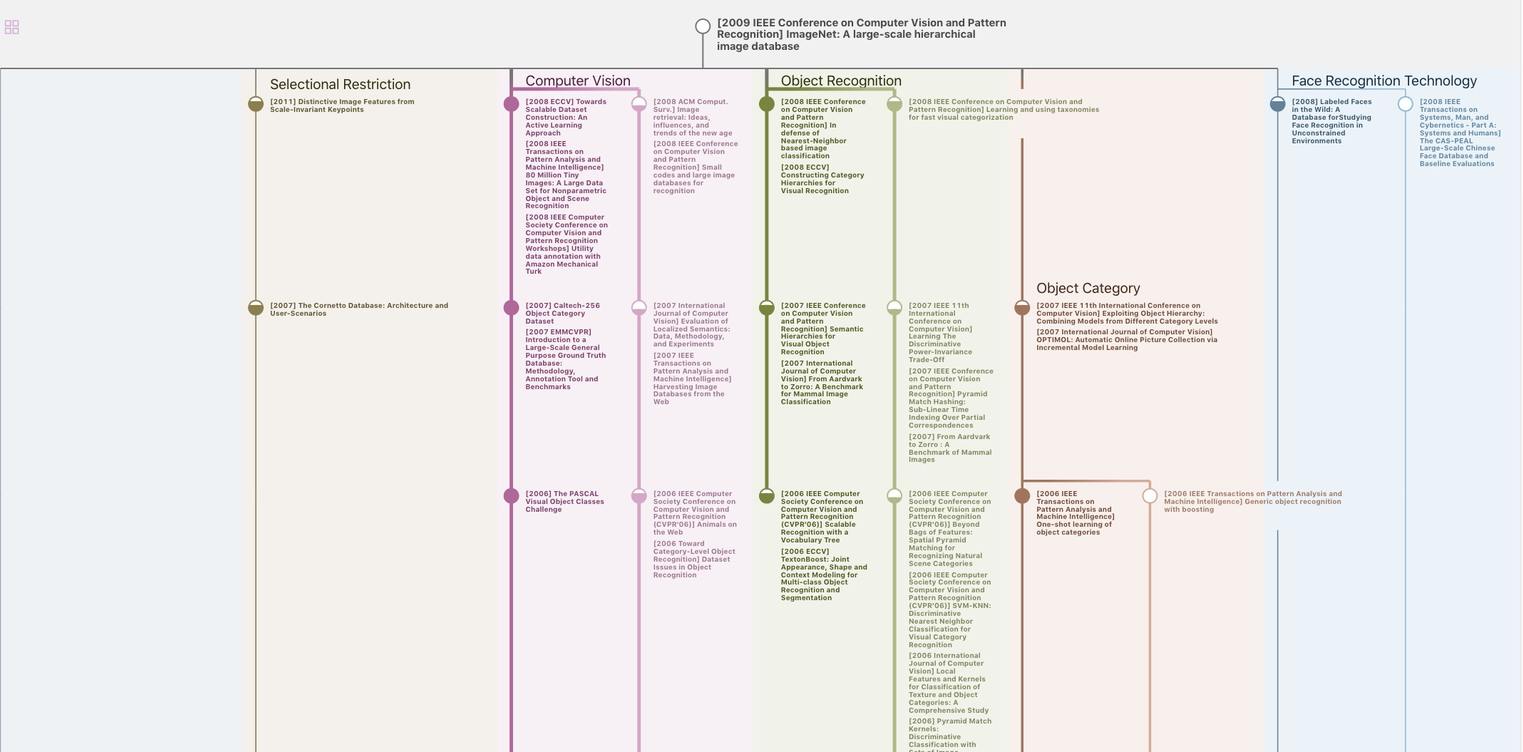
生成溯源树,研究论文发展脉络
Chat Paper
正在生成论文摘要