An adaptive multi-domain feature joint optimization framework based on composite kernels and ant colony optimization for motor imagery EEG classification
BIOMEDICAL SIGNAL PROCESSING AND CONTROL(2020)
摘要
Brain computer interface (BCI) is a novel technology that translates human intention into command to control external device. Common spatial pattern (CSP) algorithm is most frequently applied for feature engineering in motor imagery (MI) based BCI system. How to select the most suitable spatial channels, temporal & frequency parameters for different people before CSP is still a challenging issue which greatly affects the performance of MI based BCI system. In this paper, we introduce an adaptive multi-domain feature joint optimization framework. Specifically, random forest (RF) and composite kernel support vector machine (CKSVM) algorithms are used to measure the significances of different spatial channels and local temporal-frequency segments. An ant colony optimization (ACO) based scheme is proposed to search the most suitable spatial channels and temporal-frequency segments. We evaluated the effectiveness of the proposed algorithm on public BCI competition III data set IVa and two self-collected MI EEG datasets. For BCI competition III data set IVa, our method outperforms some other close related algorithms in the literature. For the two self-collected datasets, compared to the traditional manual parameter setting, the classification performance is proven to significantly improve (more than 15%) adopting our adaptive multi-domain parameters. Since our proposed method can simultaneously and automatically optimize subject-specific features in the entire spatial-temporal-frequency domains, the most discriminative CSP features can be selected and the performance of MI EEG classification is significantly improved. Thus, our research is a useful complement to the BCI field. (C) 2020 Elsevier Ltd. All rights reserved.
更多查看译文
关键词
Brain computer interface,Motor imagery,Random forest,Composite kernel support vector machine,Ant colony optimization
AI 理解论文
溯源树
样例
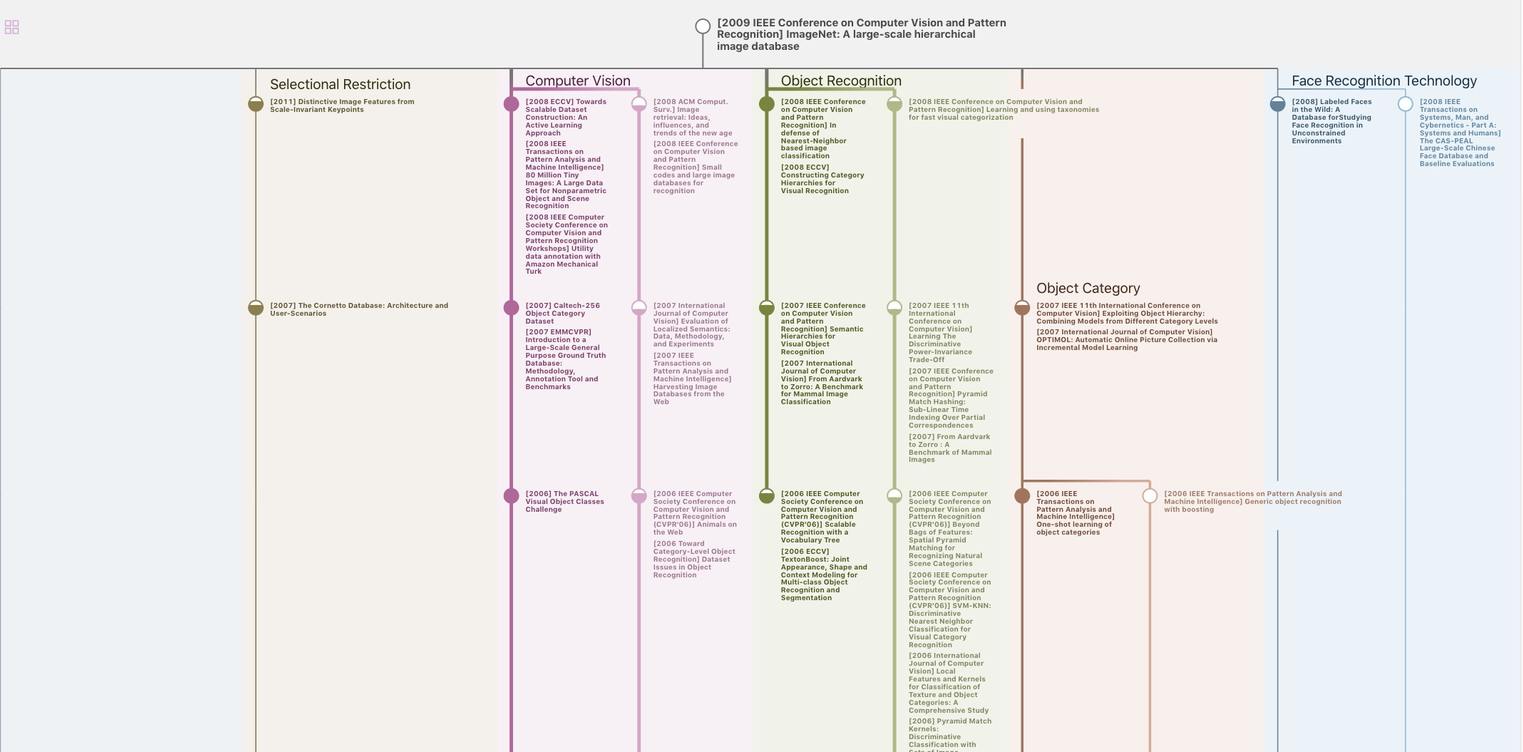
生成溯源树,研究论文发展脉络
Chat Paper
正在生成论文摘要