Stochastic Sequential Modeling: Toward Improved Prostate Cancer Diagnosis Through Temporal-Ultrasound
ANNALS OF BIOMEDICAL ENGINEERING(2020)
摘要
Prostate cancer (PCa) is a common, serious form of cancer in men that is still prevalent despite ongoing developments in diagnostic oncology. Current detection methods lead to high rates of inaccurate diagnosis. We present a method to directly model and exploit temporal aspects of temporal enhanced ultrasound (TeUS) for tissue characterization, which improves malignancy prediction. We employ a probabilistic-temporal framework, namely, hidden Markov models (HMMs), for modeling TeUS data obtained from PCa patients. We distinguish malignant from benign tissue by comparing the respective log-likelihood estimates generated by the HMMs. We analyze 1100 TeUS signals acquired from 12 patients. Our results show improved malignancy identification compared to previous results, demonstrating over 85% accuracy and AUC of 0.95. Incorporating temporal information directly into the models leads to improved tissue differentiation in PCa. We expect our method to generalize and be applied to other types of cancer in which temporal-ultrasound can be recorded.
更多查看译文
关键词
Image guided diagnosis, Hidden Markov models, Time-domain analysis, TRUS-guided biopsies, Tissue characterization
AI 理解论文
溯源树
样例
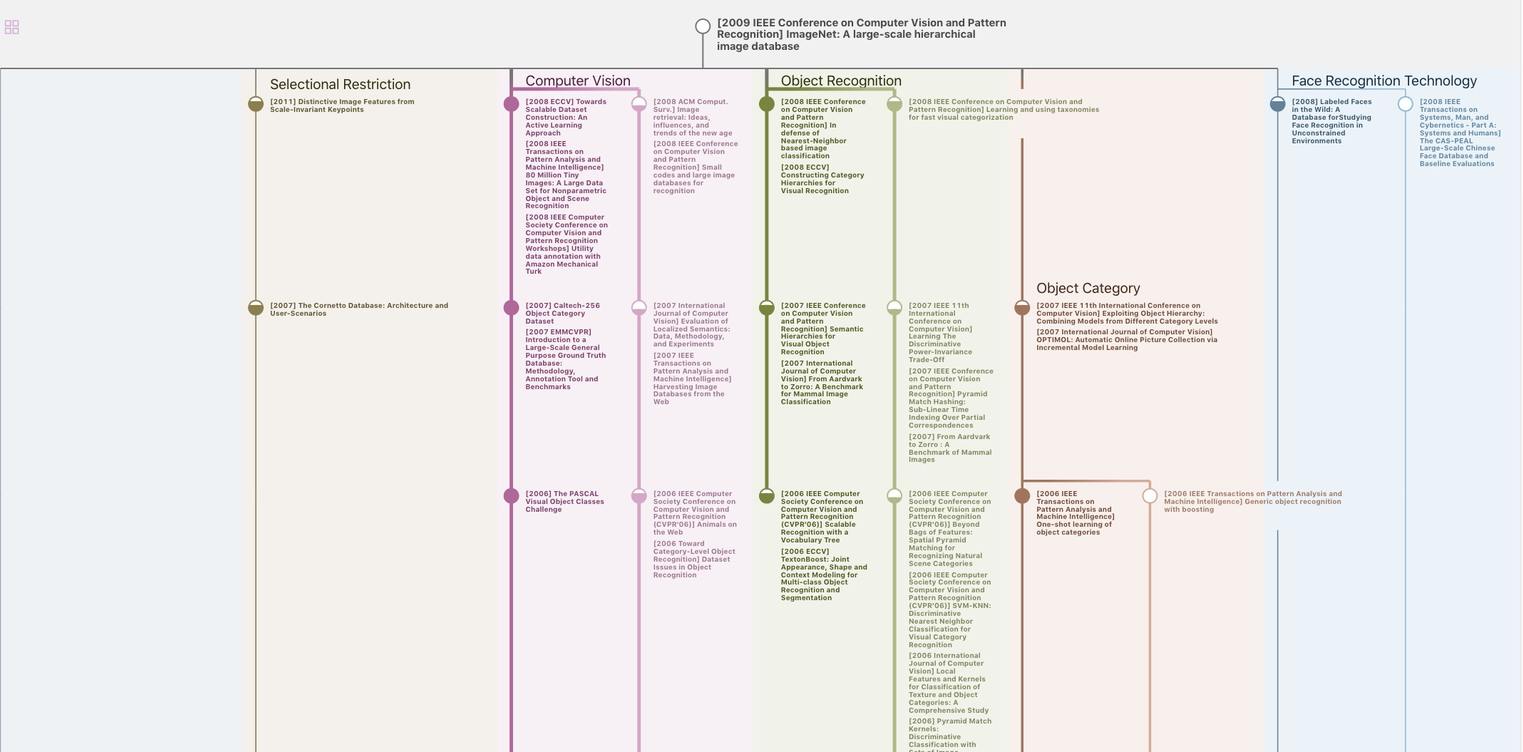
生成溯源树,研究论文发展脉络
Chat Paper
正在生成论文摘要