Learning Spectral Templates for Photometric Redshift Estimation from Broadband Photometry
ASTRONOMICAL JOURNAL(2020)
摘要
Estimating redshifts from broadband photometry is often limited by how accurately we can map the colors of galaxies to an underlying spectral template. Current techniques utilize spectrophotometric samples of galaxies or spectra derived from spectral synthesis models. Both of these approaches have their limitations: either the sample sizes are small and often not representative of the diversity of galaxy colors, or the model colors can be biased (often as a function of wavelength), which introduces systematics in the derived redshifts. In this paper, we learn the underlying spectral energy distributions from an ensemble of similar to 100 K galaxies with measured redshifts and colors. We show that we are able to reconstruct emission and absorption lines at a significantly higher resolution than the broadband filters used to measure the photometry for a sample of 20 spectral templates. We find that our training algorithm reduces the fraction of outliers in the derived photometric redshifts by up to 28%, bias up to 91%, and scatter up to 25%, when compared to estimates using a standard set of spectral templates. We discuss the current limitations of this approach and its applicability for recovering the underlying properties of galaxies. Our derived templates and the code used to produce these results are publicly available in a dedicated Github repository:.
更多查看译文
关键词
Galaxy photometry,Photometry,Astronomical techniques,Spectral energy distribution,Redshifted,Cosmology,Redshift surveys,Computational methods,Astronomical methods,Astronomy data analysis
AI 理解论文
溯源树
样例
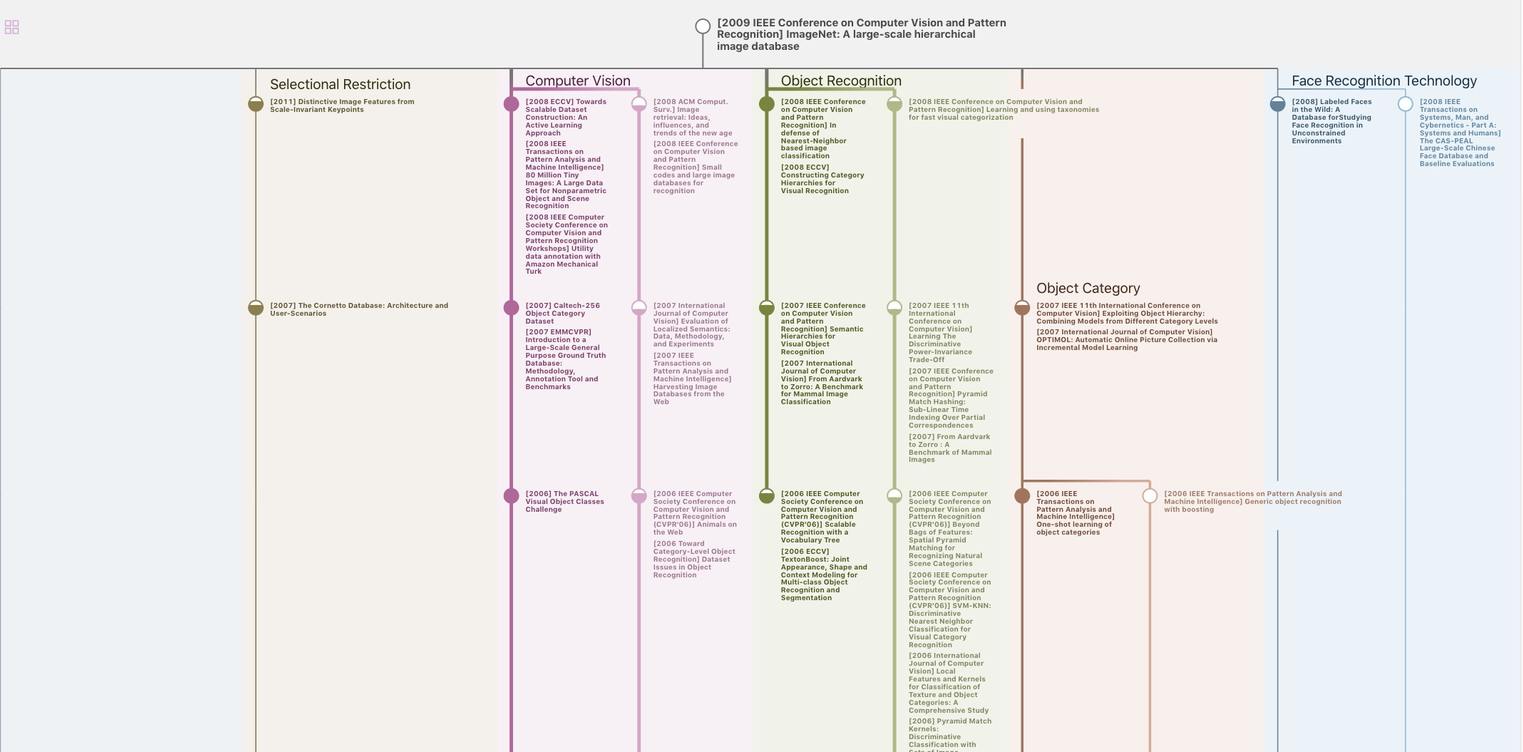
生成溯源树,研究论文发展脉络
Chat Paper
正在生成论文摘要