Generalization Error Of Minimum Weighted Norm And Kernel Interpolation
SIAM JOURNAL ON MATHEMATICS OF DATA SCIENCE(2021)
摘要
We study the generalization error of functions that interpolate prescribed data points and are selected by minimizing a weighted norm. Under natural and general conditions, we prove that both the interpolants and their generalization errors converge as the number of parameters grows, and the limiting interpolant belongs to a reproducing kernel Hilbert space. This rigorously establishes an implicit bias of minimum weighted norm interpolation and explains why norm minimization may either benefit or suffer from over-parameterization. As special cases of this theory, we study interpolation by trigonometric polynomials and spherical harmonics. Our approach is from a deterministic and approximation theory viewpoint, as opposed to a statistical or random matrix one.
更多查看译文
关键词
generalization error, interpolation, kernel spaces, weighted norm
AI 理解论文
溯源树
样例
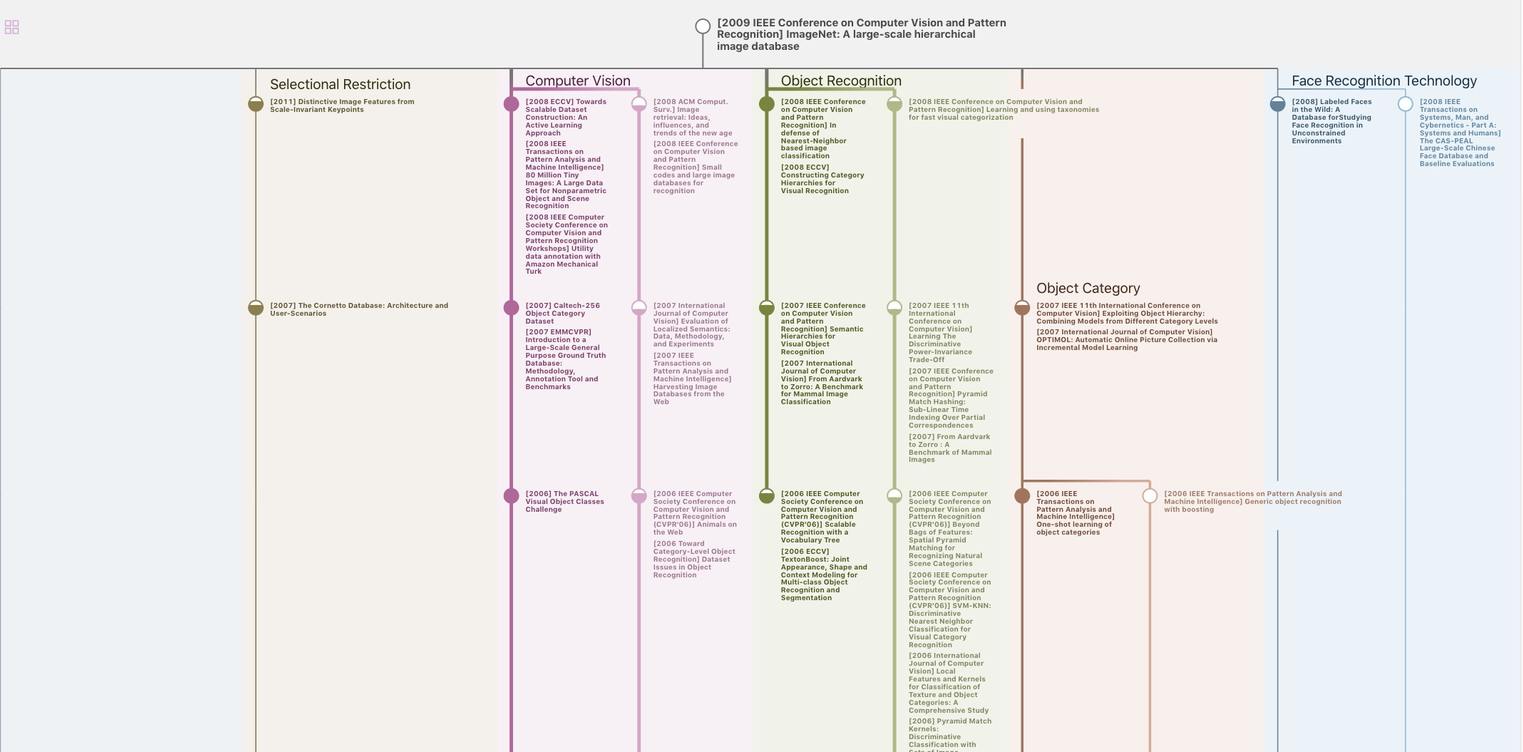
生成溯源树,研究论文发展脉络
Chat Paper
正在生成论文摘要