Stable reliability diagrams for probabilistic classifiers.
Proceedings of the National Academy of Sciences of the United States of America(2021)
摘要
A probability forecast or probabilistic classifier is reliable or calibrated if the predicted probabilities are matched by ex post observed frequencies, as examined visually in reliability diagrams. The classical binning and counting approach to plotting reliability diagrams has been hampered by a lack of stability under unavoidable, ad hoc implementation decisions. Here, we introduce the CORP approach, which generates provably statistically consistent, optimally binned, and reproducible reliability diagrams in an automated way. CORP is based on nonparametric isotonic regression and implemented via the pool-adjacent-violators (PAV) algorithm-essentially, the CORP reliability diagram shows the graph of the PAV-(re)calibrated forecast probabilities. The CORP approach allows for uncertainty quantification via either resampling techniques or asymptotic theory, furnishes a numerical measure of miscalibration, and provides a CORP-based Brier-score decomposition that generalizes to any proper scoring rule. We anticipate that judicious uses of the PAV algorithm yield improved tools for diagnostics and inference for a very wide range of statistical and machine learning methods.
更多查看译文
关键词
calibration,discrimination ability,probability forecast,score decomposition,weather prediction
AI 理解论文
溯源树
样例
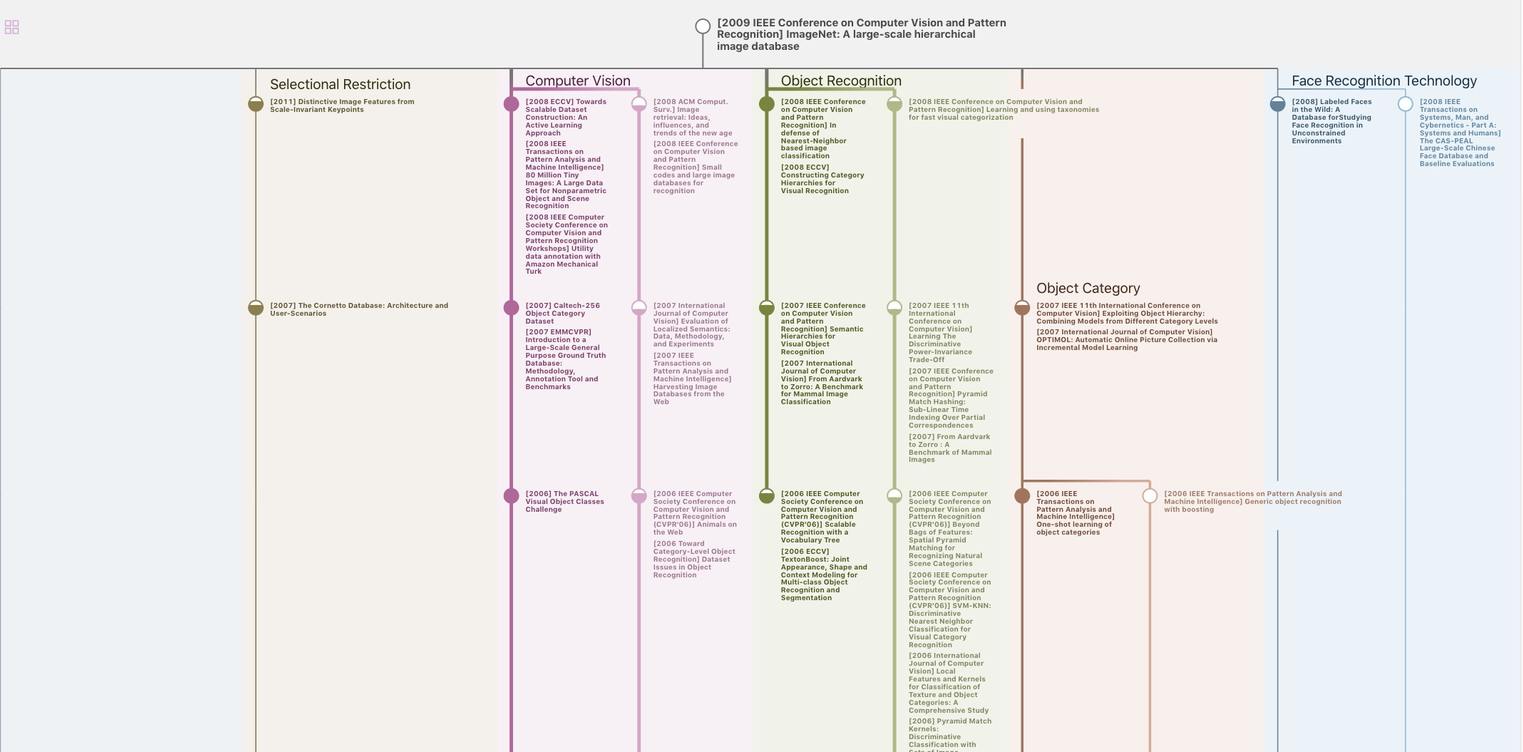
生成溯源树,研究论文发展脉络
Chat Paper
正在生成论文摘要