Relationship Matters - Relation Guided Knowledge Transfer for Incremental Learning of Object Detectors.
CVPR Workshops(2020)
Abstract
Standard deep learning based object detectors suffer from catastrophic forgetting, which results in performance degradation on old classes as new classes are incrementally added. There has been a few recent methods that attempt to address this problem by minimizing the discrepancy between individual object proposal responses for old classes from the original and the updated networks. Different from these methods, we introduce a novel approach that not only focuses on what knowledge to transfer but also how to effectively transfer for minimizing the effect of catastrophic forgetting in incremental learning of object detectors. Towards this, we first propose a proposal selection mechanism using ground truth objects from the new classes and then a relation guided transfer loss function that aims to preserve the relations of selected proposals between the base network and the new network trained on additional classes. Experiments on three standard datasets demonstrate the efficacy of our proposed approach over state-of-the-art methods.
MoreTranslated text
Key words
relationship matters,relation guided knowledge transfer,incremental learning,object detectors,standard deep learning,catastrophic forgetting,performance degradation,old classes,individual object proposal responses,proposal selection mechanism,ground truth objects,relation guided transfer loss function,base network
AI Read Science
Must-Reading Tree
Example
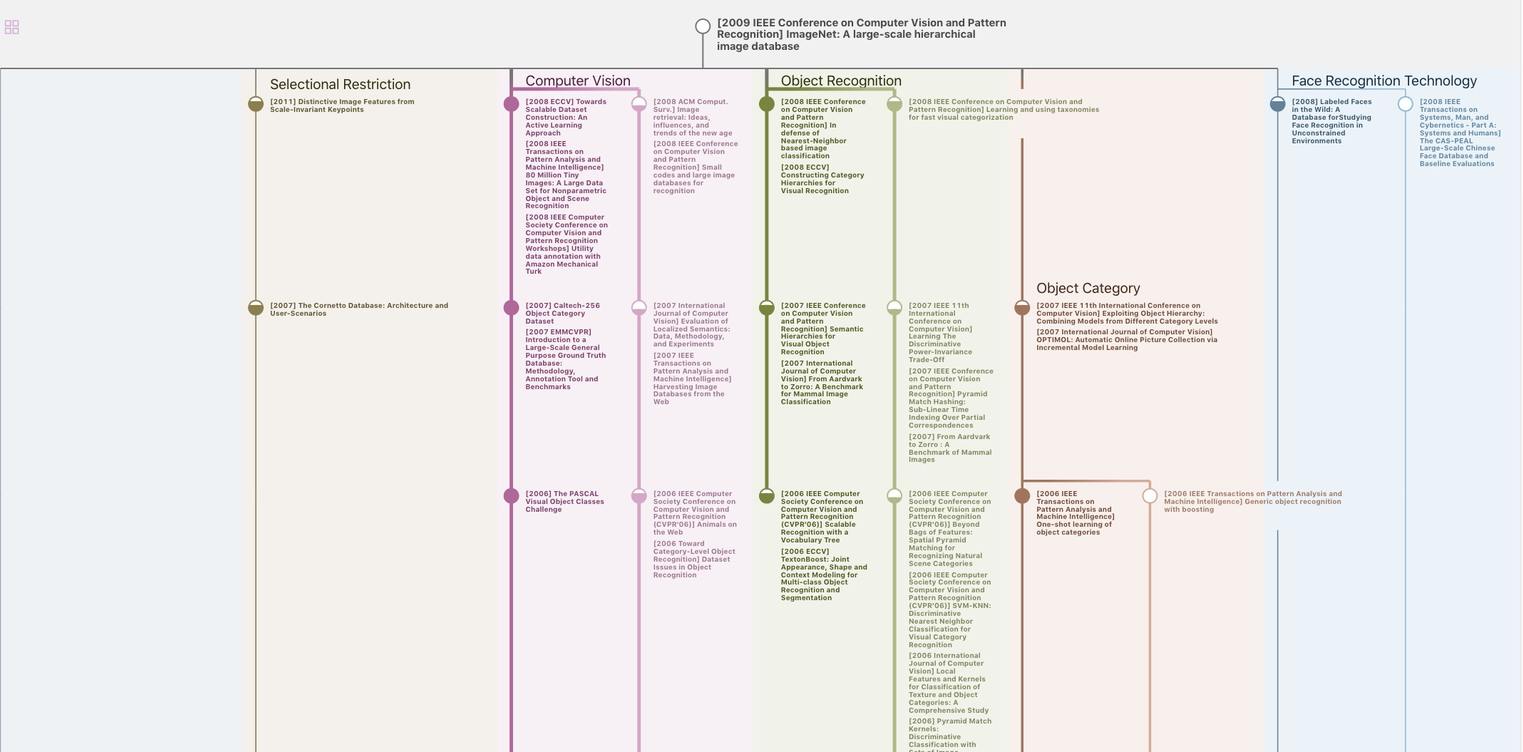
Generate MRT to find the research sequence of this paper
Chat Paper
Summary is being generated by the instructions you defined