Improve Image Codec’s Performance By Variating Post Enhancing Neural Network:Submission of zxw for CLIC2020
2020 IEEE/CVF Conference on Computer Vision and Pattern Recognition Workshops (CVPRW)(2020)
摘要
Adding post enhancing filter after traditional image decoder to improve reconstruction quality is nowadays a very common method [1],[2],[3],[5]. Researchers use a large network filter or repeatedly stack single/multiple types of relative simple filters together. They all achieved better results. On the other hand the training materials and training time increases exponentially for a larger network scale, and the performance improvement becomes less and little. We learn this experience from the CLIC2019 low-rate track, where we proposed the VimicroABCnet and VimicroSpeed[4], with 2 post filters of different scale. The later one(5 time larger than the small one) achieved the final test's PSNR by improvement of only 0.02db@0.15bpp. In this paper, we propose a method to variate an existing post network filter(base filter). The base filter is altered into different ones, alternation only happens to weights. The key of the method is to divide the training data into different groups. Based on the pre-trained base filter, different altered filters are individually fine-trained with different group of training data. There are different ways to divide the training data, and we use a relative simple one. Sort by compression rate(with traditional codec) and bin the training images in to 4/8 group of training data subsets. With the new filters plus the base one, we now have 5/9 filters candidates in encoding phase and choose the best. The CLIC2019 test data show that PSNR increases 0.04db@0.15bpp and 0.06db@0.15bpp than the one filter VimicroSpeed method. This method requires the same training data and perfectly suitable for multi-GPU training scheme, and retraining the altered filters is much easier and consuming less time than training a relative large network filter. Also the result is better(5 filters scheme@0.04db vs VimicroABCnet@0.02db).
更多查看译文
关键词
performance improvement,CLIC2019 low-rate track,different ones,pre-trained base filter,different altered filters,different group,relative simple one,traditional codec,training images,training data subsets,CLIC2019 test data,filter VimicroSpeed method,multiGPU training scheme,improve image codec,variating post enhancing neural network:submission,CLIC2020,adding post enhancing filter,traditional image decoder,relative simple filters,training materials,training time increases,larger network scale
AI 理解论文
溯源树
样例
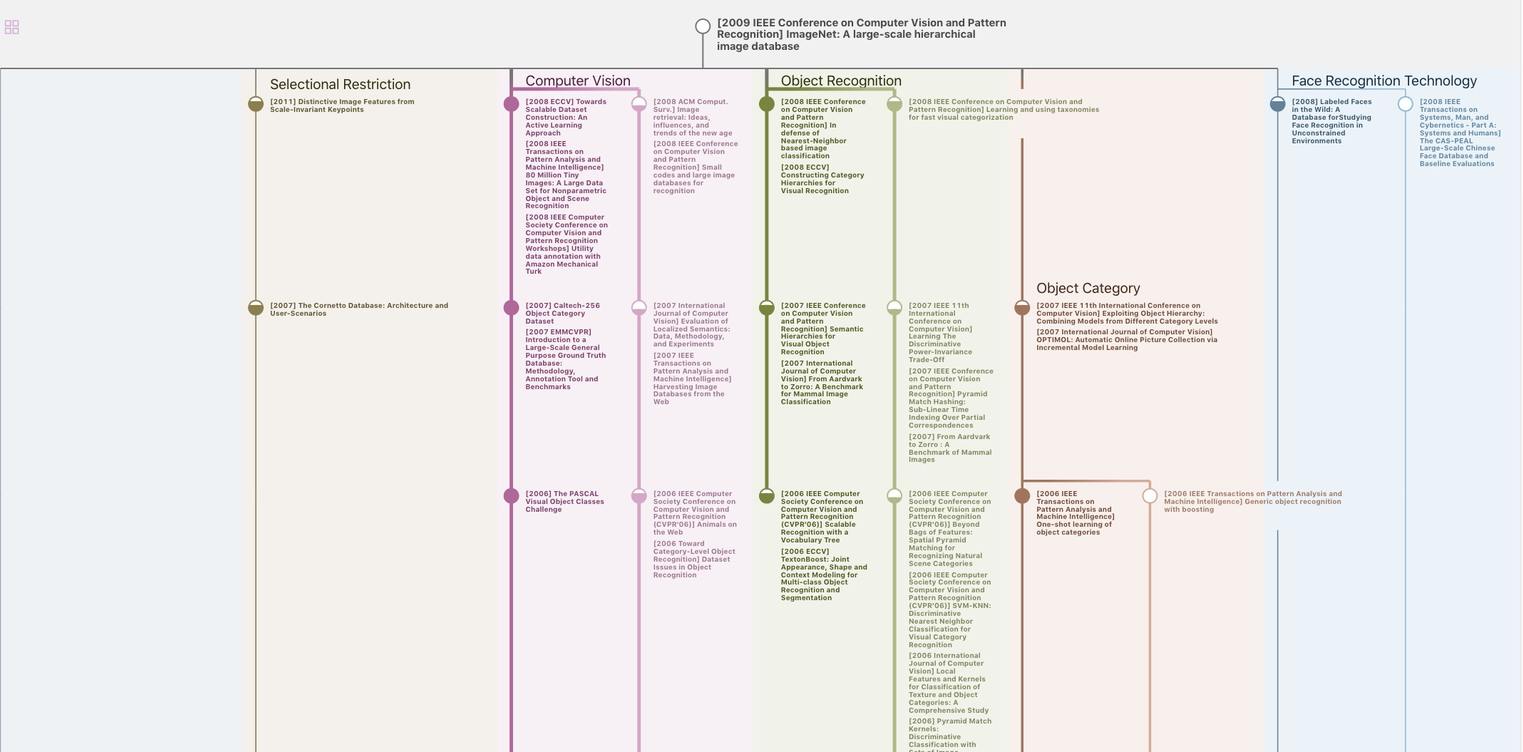
生成溯源树,研究论文发展脉络
Chat Paper
正在生成论文摘要