Identifying Sparse Low-Dimensional Structures In Markov Chains: A Nonnegative Matrix Factorization Approach
2020 AMERICAN CONTROL CONFERENCE (ACC)(2020)
摘要
We consider the problem of learning low-dimensional representations for large-scale Markov chains. We formulate the task of representation learning as that of mapping the state space of the model to a low-dimensional state space, called the kernel space. The kernel space contains a set of meta states which are desired to be representative of only a small subset of original states. To promote this structural property, we constrain the number of nonzero entries of the mappings between the state space and the kernel space. By imposing the desired characteristics of the representation, we cast the problem as a constrained nonnegative matrix factorization. To compute the solution, we propose an efficient block coordinate gradient descent and theoretically analyze its convergence properties.
更多查看译文
关键词
low-dimensional structures,nonnegative matrix factorization approach,low-dimensional representations,large-scale Markov chains,representation learning,low-dimensional state space,kernel space,meta states,original states,structural property,constrained nonnegative matrix factorization
AI 理解论文
溯源树
样例
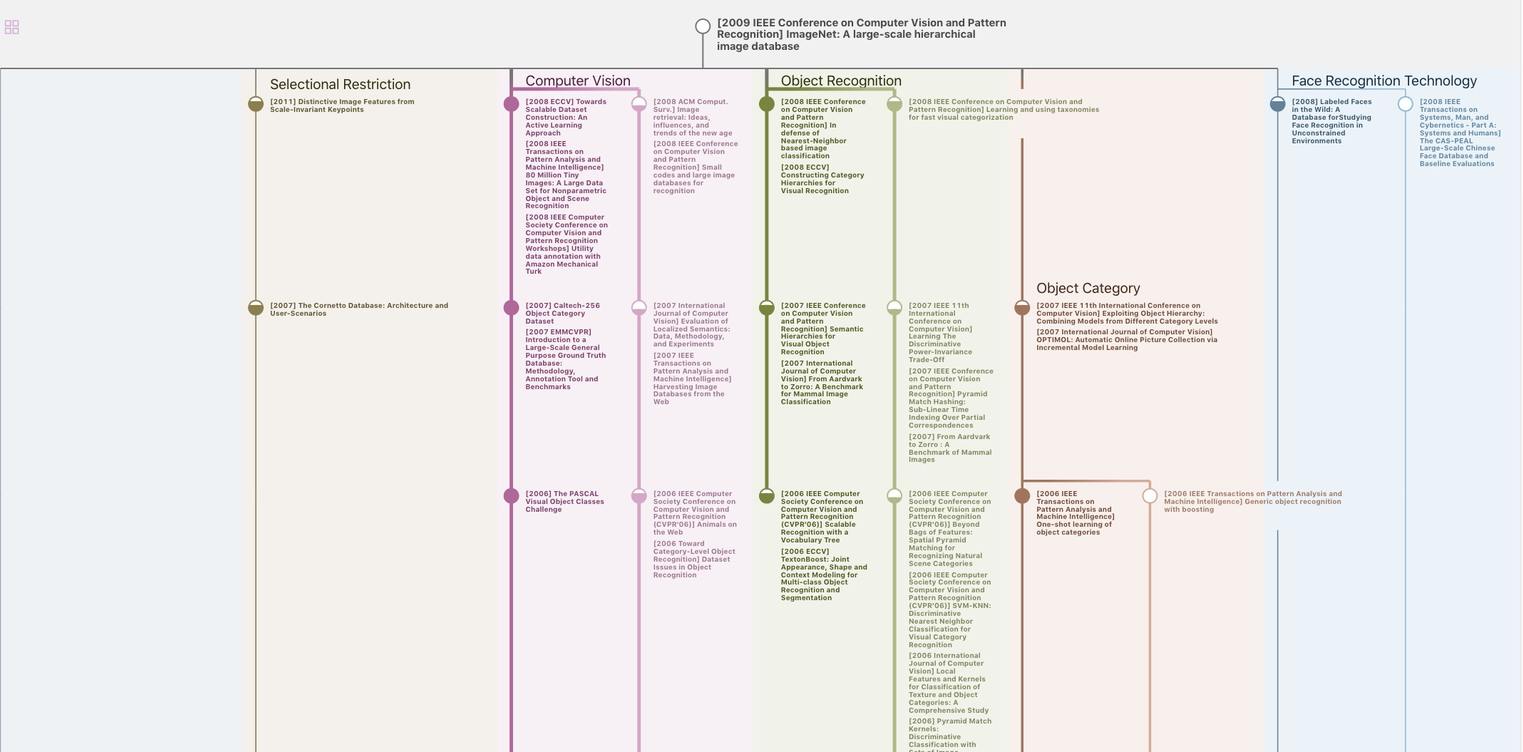
生成溯源树,研究论文发展脉络
Chat Paper
正在生成论文摘要